Editor’s Note: Today’s post is by Christopher Leonard. Chris works at Cactus Communications as Director of Product Solutions.
Peer review, a process popularized during the post-war expansion of academic journals publishing, is struggling to keep up with the sheer volume of research we publish today. A process that worked for getting two or three suitably qualified peers to pass judgement and suggest improvements on a worldwide output of 100,000 papers in the late 1950s is, unsurprisingly, struggling as we have now surpassed the five million annual published papers mark.
Given that each paper is typically reviewed by at least two reviewers (and more for papers that are rejected and resubmitted elsewhere), then each and every one of the estimated eight to nine million active researchers in the world could be expected to provide at least one peer review report each year. Which sounds manageable, but of course only a fraction of all researchers are asked to review, compounding the problem on the shoulders of active reviewers who get invitations to review every few weeks (if not every few days). This is not only unfair to those reviewers, but inequitable in terms of the lack of opportunity for those who are not invited.
And it’s not just reviewers who are struggling with all these invitations and demands on their time. A quick trawl on Twitter/X reveals many publishing editors bemoaning the fact that they’ve sent out 20+ reviewer invitations and not had any acceptances, meaning a reluctant desk rejection after many weeks of waiting for the unlucky author.
How did we get here? Well, the current system of using publications to adjudicate career advancement is certainly a part of the problem — but let’s save revolution in that arena for another post and another day. Expansion of the reviewer pool is another simple remedy that we could apply (e.g., relaxing the criteria for what qualifications a peer reviewer must have, plus training and inviting a wider range of reviewers), but that would only work in a limited way and for a limited time.
Since we’re living in the age of AI, surely there is a way to use the various tools at our disposal to speed up the peer review process — and, while we’re at it, to address some of the other problems around bias and quality. Language and text processing are what the current range of large language models do best, so is it a good idea to include LLMs (Large Language Models) in the peer review process? Would doing so also give researchers back some of the estimated 15 thousand person years per year spent on peer review so they can do more actual research?
In order to answer that question, I’m first going to take a step back and define what we mean by peer review. It can be construed in many ways, but for the purposes of this analysis, I’m defining peer review as one of the final parts of the quality and validation process for academic research manuscripts. A partial list of areas where it is possible to increase quality of an academic manuscript includes:
- Idea generation
- Experimentation and writing a paper
- Submission to a journal
- Research integrity evaluation
- Peer review reports
- Post-publication review
I’ve broken the process down like this for two reasons. First, it shows that even before we get to formal peer review report generation, there are many other opportunities to increase the quality of the paper, so that we’re not sending junk out to reviewers, but rather high-quality submissions that deserve their valuable time. Second, as we move from items 1 to 6, there is a dual trend where the usefulness of AI is strong and tails off as the necessity for human input increases.
If you’ll forgive the generalizations and liberties I’ve taken with the process, we now have a framework for discussing where AI can be useful today, before we discuss where it might be useful tomorrow.
Using AI Today
- Idea generation. Anyone who has spent some time playing with ChatGPT will be aware of its capability for associating two ideas in a number of interesting ways, as demonstrated in the screenshot below.
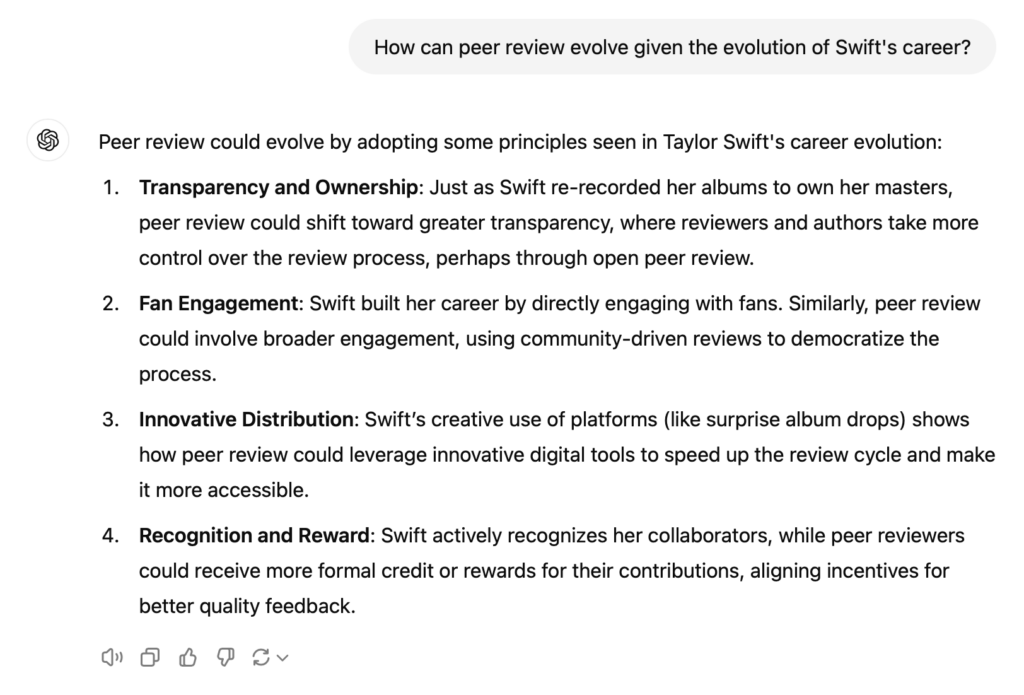
This, and other LLMs, are tools that can be used by researchers looking for a new angle on a research problem. Half an hour spent on ChatGPT could spawn many suggestions, and although they probably shouldn’t be used on their own, they are helpful for triggering thoughts for getting out of a dead end, or for new research directions. More ideas hopefully lead to better ideas, and better resulting papers.
- Experimentation and writing. While the concept of using AI for experimentation may still be some way off (although Sakana AI are showing that time may not be far away), artificial intelligence can help with the writing stage. Authoring an academic paper is hard for native English speakers; for the majority of the world’s researchers, for whom English is a second language, it’s even harder. Many tools are available to help authors improve their language, grammar, and other aspects of their written communications, during the authoring process. Tools like Paperpal for Word even sit alongside the manuscript and give in context help. Better writing leads to better papers.
- Journal submission. At the point of submitting a manuscript, a variety of checks are performed to ensure the manuscript fulfils all of the criteria for a full submission. Information about length of title, self-citations, presence of funding statements, and whether all figures and tables are cited in the text are mundane tasks that AI largely excels at and peer reviewers can be easily relieved of, allowing them to focus on the content of the paper.
- Research integrity. Evaluating papers for a range of research integrity issues has become a big problem (partly thanks to AI itself, of course). But again, many checks on research papers can be automated — for example, to determine citation cartels, possible authorship sales, figure manipulation, and good old-fashioned plagiarism. While many services exist to flag potential problems, we’re not at the point where AI can definitively determine the existence of a research integrity problem. Human intervention is required here to analyze each red flag and pass judgement on whether this is a serious problem which requires a desk reject, or a minor infringement that can be ignored or addressed with a revision.
- Peer review. All of the steps outlined above show how AI can help improve the content and structure of papers *before* they even reach the peer review stage. This is the stage where the use of AI becomes more controversial, and I address it below.
- Post-publication peer review. If everything else has gone well, this shouldn’t be necessary — and, given that AI has done all it can to this point, anything which remains can only be found by human experts. This is an important, but hopefully infrequently needed, check on the whole process.
Peer review report generation today
As I hope I have illustrated, the use of AI in peer review sits at the points where AI is useful, but human oversight is still a requirement. If you feed a manuscript into most of the popular LLMs today and say ‘write a peer review report of this manuscript’ it will return something which looks like a peer review report: a summary of the paper, some of its strengths, some weaknesses/areas for improvement, and an overall recommendation about whether to accept/minor revisions/major revisions/reject. Amazing!
But scratch beneath the surface, and all is not as it seems. The manuscript is evaluated as an entity in itself — there is little or no comparison with previous literature, there is little or no evaluation of novelty, suggested references are prone to hallucinations, and there is a depressing tendency to rate everything as a minor revision.
BUT, there is *something* there. LLMs have an ability to spot problems human reviewers sometimes miss or gloss over. LLMs don’t get fixated on one aspect of the paper and keep coming back to it, and LLMs are quick. The best use of LLMs in peer review today could be their use by the editor to cross-check human peer review reports and make sure nothing has been missed or downplayed before querying with the reviewer. This requires smarter prompting than just ‘write a peer review report’, but is still a simple way to check the reviews before they are returned to the author. In this sense we are kind of at Phase 4 in the 5-phase transition to ending dependence on humans for peer review.
Generating an LLM report and asking a reviewer to edit it is a more risky approach. The reviewer is likely to accept whatever the LLM has generated rather than being sufficiently critical. A mix of human reviews and AI checks therefore seems the best way to incorporate AI into peer review today.
Peer review report generation tomorrow
Where is this all leading? Predicting the future is a fool’s game, but since I was asked to write this, here goes:
The objections to using AI alone to create a peer review report are likely to disappear over the next year or so. Privacy concerns (around the unpublished work being used to train the model, and potentially appearing in answers) can already be addressed with use of APIs or judicious use of LLMs. Knowledge of the previous literature and measures of novelty are likely to be solved with knowledge graphs and the next generation of LLMs. ChatGPT 5 and Claude 4 may well amaze us for content and structure issues in the way ChatGPT3 did at the end of 2022. Given that we are only two years into the LLM revolution, what the next five years have in store is worth playing out in your head.
It is the distant future — the year 2026. We are still encouraging authors to write manuscripts themselves, not to use the ‘instant article’ feature in Claude 4 (in my imagination) to turn data into a manuscript. Once they have written their paper, it can be analyzed using the (still imaginary) ChatGPT5 Peer Reviewer bot, which not only links the research to previous papers (assessing novelty and providing missing references where necessary), but also polishes the language, structure, tables, and figures so that they match the standard you might see in The Lancet or Nature today. One great paper (or as good as the data allows) is now ready for submission. Then what?
Will they submit it to a journal that charges $x000 for an APC? Or will they submit to a preprint server which has inbuilt functionality to create three peer review reports for each submission instantly, assign a score to each review, and publish the paper, score, and reviews? Heck, readers can even generate their own report to highlight their own areas of interest and how the article relates to their own work.
So, what role is there for journals in this scenario? We have a little time to figure that out, but probably not as much time as you think.
Discussion
1 Thought on "Guest Post — Is AI the Answer to Peer Review Problems, or the Problem Itself?"
The suggested method will be perfect for articles written by another LLM that can use those three peer reviews to improve itself.
Humans are not perfect, but indispensable.