As artificial intelligence (AI) systems become increasingly integrated into scholarly research and knowledge dissemination, publishers face a critical challenge: how to best integrate their content into this new ecosystem. Currently, an increasing number of publishers are collaborating with AI system suppliers to license their content for large language model training, small model development, and retrieval-augmented generation applications. Many of the largest publishers have announced licensing arrangements with the largest model developers and likely many, many more are under the radar at other publishers as well. Although few can discuss the process, it is easy to imagine the difficulties some of the deals are facing behind the scenes. With all these discussions happening behind closed doors and often under the cloak of non-disclosure agreements, there might be an opportunity for transparency to support the entire publishing industry to move toward more efficient and effective outcomes. The absence of a standardized licensing framework, similar to what has been in place for licensing to the library community, is probably leading to inefficiencies and legal complexities.
During a Book Industry Study Group meeting last month entitled Doing Rights Right 2025, I spoke about the opportunities and challenges of licensing content to AI systems providers for the publishing community. In that talk, I described how the current situation has resonance with the challenges faced during the early days of online journal subscription licensing for libraries. By learning from past licensing efforts, the scholarly publishing industry — hopefully in partnership with partners from the AI development world — can develop model license frameworks that streamline negotiations, mitigate legal uncertainties, create a more equitable environment among the partners, and establish clearer expectations for AI system developers.
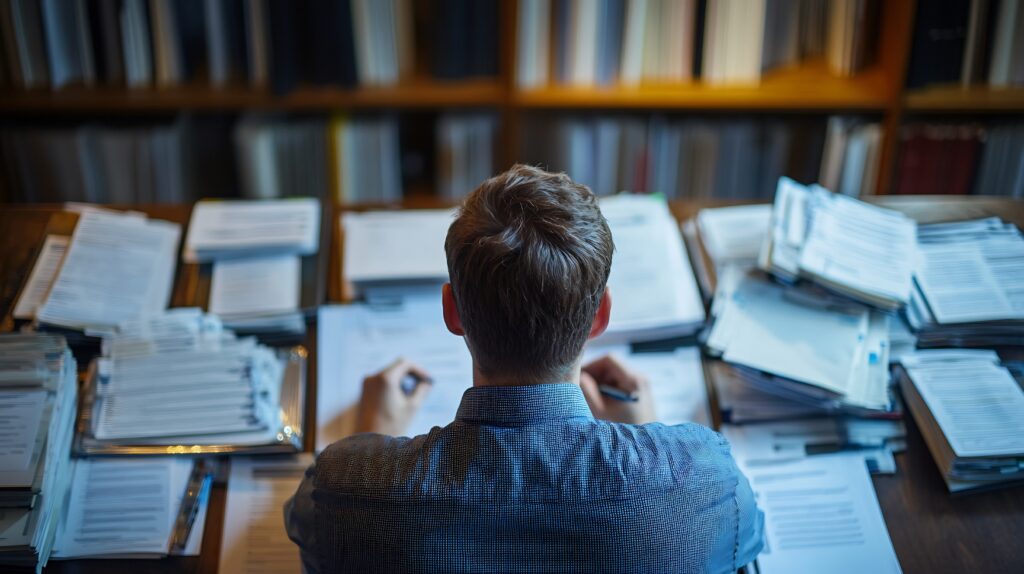
Learning from the history of standardized licenses
When online journal subscriptions became pervasive in the academic marketplace in the late 1990s, the licensing process was initially inconsistent, cumbersome, and time-consuming. Each license was a bespoke agreement, typically initiated with a draft from the publisher’s legal office. Even after pricing and business conditions were settled, legal teams from both the publisher and the subscribing library engaged in extensive negotiations over contract specifics. This slow, intricate and expensive process did not scale effectively across numerous publishers, products, and libraries.
In the late 1990s, an initiative between the UK’s Publishers Association and JISC (formerly the Joint Information Systems Committee of the Higher Education Funding Councils) jointly developed the PA/JISC model license. The early 2000s saw other efforts to develop model licenses or statements of principles with recommended terms from consortia and ICOLC, which greatly simplified these negotiations. Through the twenty-teens, these licenses continued to be updated to address ongoing developments and applications. A 2013 study by Eschenfelder, et. al. on the adoption of license and an analysis of their terms showed both significant increase in the number of licenses and convergence on consistent application of recommended terms.
Today, most library licensing agreements begin with an agreed-upon template, significantly expediting the process. While most publishes have their own preferred templates, the consistency brought about through years of collaboration and discussion about terminology and core issues have helped to speed the negotiation process, saving untold number of hours in legal negotiation and likely millions of dollars in cost savings. In addition, having a public conversation about model terms, language, and issues allowed for collective analysis and discussion of goals and outcomes, as exemplified in the Escehfelder study as well as other work done around that time and since.
The Current Landscape of Licensing Content for AI
Today, the AI licensing landscape is in a similarly fragmented state as content licensing in the 1990s. Publishers and AI developers must negotiate agreements from scratch, often with widely varying terminology and expectations across providers. This lack of standardization results in protracted negotiations and operational inefficiencies. Having public conversations about the community expectations and developing model licenses for AI content usage — just as scholarly publishing did for digital content access — can facilitate smoother transactions and reduce barriers to entry for both publishers and AI developers.
There are several startups focusing on this issue such as Calliope and Created by Humans, which are attempting to aggregate and license copyrighted works for ingestion by AI models. An association of many of these players have organized into the Dataset Providers Alliance. Launched last summer, the Alliance aims to “[advocate] for the interests of rights holders and works to create a sustainable and equitable ecosystem for the licensing of intellectual property content in the AI and ML industries.” While the group might have potential to advance the issue more broadly, to date it appears to have only produced a single white paper. In addition, the Alliance, given its scope, isn’t likely to specifically address the key concerns for the scholarly community. Also the Copyright Clearance Center has promoted a solution for collective licensing models for AI, though this approach has its limitations and also serves a different market need.
Another interesting aspect of this ecosystem is the somewhat flipped nature of the power structure in these arrangements compared with the relationship between libraries in the 1990s and the AI systems developers of today. In the 1990s, libraries and consortia were keen to attempt to exert some collective control over the direction of license negotiation as purchasers of content, when they felt sidelined regarding some their interests. Today, those seeking to license content seem to be in a more dominant position when dictating terms, such as non-disclosure, perhaps because of their size and resources. The approach of software tool providers has been, unlike libraries, to take what they want and then ask permission after the fact. We will see whether this approach has any legal standing, but at least initially the fair-use arguement hasn’t survived early challenges.
How Model Licenses Facilitate Deals and Simplify Business Agreements
Model licenses have existed in the scholarly landscape for several decades. A model license provides a common starting point for negotiations, ensuring that essential legal terms and conditions are agreed upon in principle at the outset, simply by the act of adopting the model. Parties may — and often do — build on these terms and negotiate the fine details to suit interests of both parties to the license. But having the model in place, publishers and AI developers can focus on customizing those specific business terms rather than debating foundational legal concepts. This approach allows for:
- Efficiency – Reducing the time spent in legal negotiations accelerates the deployment of AI models trained on high-quality, vetted content.
- Consistency – Establishing industry-wide definitions for key licensing terms creates transparency and trust between publishers and AI developers.
- Legal Clarity – A model license reduces ambiguity, ensuring that content usage is clearly defined and that both parties understand their rights and obligations.
- Fairness – Creating a transparent environment reduces the power imbalance based on knowledge gaps in the process of licensing.
It is important to note that the existence of a model doesn’t remove completely the process of negotiation. In a discussion with a librarian about this, the response was along the lines of “Even with the model licenses, I still have to spend a lot of time negotiating terms.” While this is certainly true, having a model allows for parties to focus on the 10-20-30% of the terms that are contentious, rather than the entire document.
Model frameworks have a long history in content licensing. They establish a common framework without codifying specific business terms such as pricing, revenue-sharing models, or exclusivity arrangements. They are also subject to negotiation and therefore ensure compliance with antitrust regulations while still fostering efficiency and fairness in negotiations.
Influencing AI Development Through More Standardized Licensing
There are at least three ways in which control can be exerted over the development of AI systems. The first is through legislation, which is a slow and heavy-weight approach to governing technology. One need only look to Section 230 as an example of outdated solution, that while well-intentioned, has led to a variety of problematic outcomes. Regulation is another approach to establishing boundaries and guidance for technical implementation. While the regulation process can be slightly faster than legislation, it lacks the permanence of legislation. At present, we are seeing many shifts in regulation and how these changes can impact marketplaces. Much like legislation, the regulatory process is challenged by the current pace of technological advancement. There is potentially significant power in the use of contractual terms of licenses to affect change in the marketplace for AI systems and how they represent published content.
There is exemplified interest — because of the growing number and value of publicly announced deals — among some AI tool developers in gaining licensed access to content. Publishers could be well positioned to use this leverage to drive more consistent recognition of the value of existing content and its role in AI systems. This would in the interests of authors, publishers and even end-users.
This leverage could be used to shape AI developers’ practices and establish industry norms for content representation in AI-generated outputs. The publishing community can proactively influence how AI systems engage with and reproduce copyrighted materials and provide for consistent use of content. For example, some agreed community guidance could provide terms that would improve systems by dealing with:
- Verbatim quotation policies to ensure AI-generated content accurately represents original sources or prevent users from not relying solely on AI-generated outputs
- Attribution requirements to properly credit publishers and authors, so that authors and creators can get credit for their work
- Content integrity safeguards to prevent misrepresentation or hallucination of scholarly material
- Inference representation to understand and portray to users how connections were developed between research objects that supports knowledge generation.
- Ethical use of scholarly information to protect privacy of subjects, ensure retracted information is removed, and secure potentially harmful research outputs
- Permissible data usage that provides guardrails about what developers or end users may do with the data provided by publishers to the model
- Usage tracking to provide business intelligence that can support future editorial development, royalty payments or similar processes
The scholarly publishing industry has already navigated the challenges of standardizing content licensing for online journal access. While the framework of model licenses hasn’t removed the need for negotiation, nor entirely replaced the need for legal review, it has simplified the process and saved everyone’s time. These past efforts provide a valuable blueprint for addressing the emerging complexities of AI content licensing. A community initiative to support the development of model licenses for the use of scholarly content by AI developers could streamline negotiations, reduce costs, and encourage more responsible AI content-usage practices. In turn, using license frameworks could ensure that AI-generated outputs respect the intellectual contributions of academic authors and uphold the credibility of published research. As the landscape is rapidly evolving, getting such an initiative moving quickly should be a priority.
Discussion
3 Thoughts on "We Could Use a Model Licensing Framework for Scholarly Content Use in AI Tools"
As far as I see, even the thought there will be restrictions on use of scholarly content by AI tools is the best argument I have heard for open-access publication. I doubt any author wants their work restricted, which will simply push the transformers to Facebook and Reddit posts. I am thinking how my typical, very niche, research publications can be made more accessible to machine learning so the publicly funded, and I hope rigorous, work is exploited.
I agree that citation is a problem for where LLM-generated material gets its information. However, it is not restricted to AI as many major journals limit the number of citations in research manuscripts to something like 30 to 60. It is hard to believe that most work published in, say, Nature builds on only 50 previous works, so presumably many papers influencing the work (and the methods) are uncited.
Thanks, Todd. Hopefully it’s not too late to get this going, as it feels like so many big players in the space have already torrented and left. I would also add we need standards on the outputs side for genAI scholarly search results… all literature summaries by tools like Scott, Elicit, Undermind, PaperQA, etc should have open answer contribution scores for papers/journals/publishers.
Err that’s Scite