If there were two issues that seem to have defined the year since the last gathering at the Frankfurt Book Fair, they would most certainly be integrity and artificial intelligence. Looking over the Scholarly Kitchen, the two topics consumed a lot of the “space on our digital table.” With the explosion of attention toward natural languages model applications in the academic and popular imagination over the past year, many have been reflecting on AI’s implications for scholarly communication.
In particular, how do these technologies impact the key trends of open access and research integrity in scholarly discourse? This fascination continued through the halls of the Messe and many associated events. So when several Chefs and other leaders from SSP were given the stage at the Frankfurt Studio, those two topics provided fertile ground for a lively discussion. Certainly the resulting conversations did not disappoint.
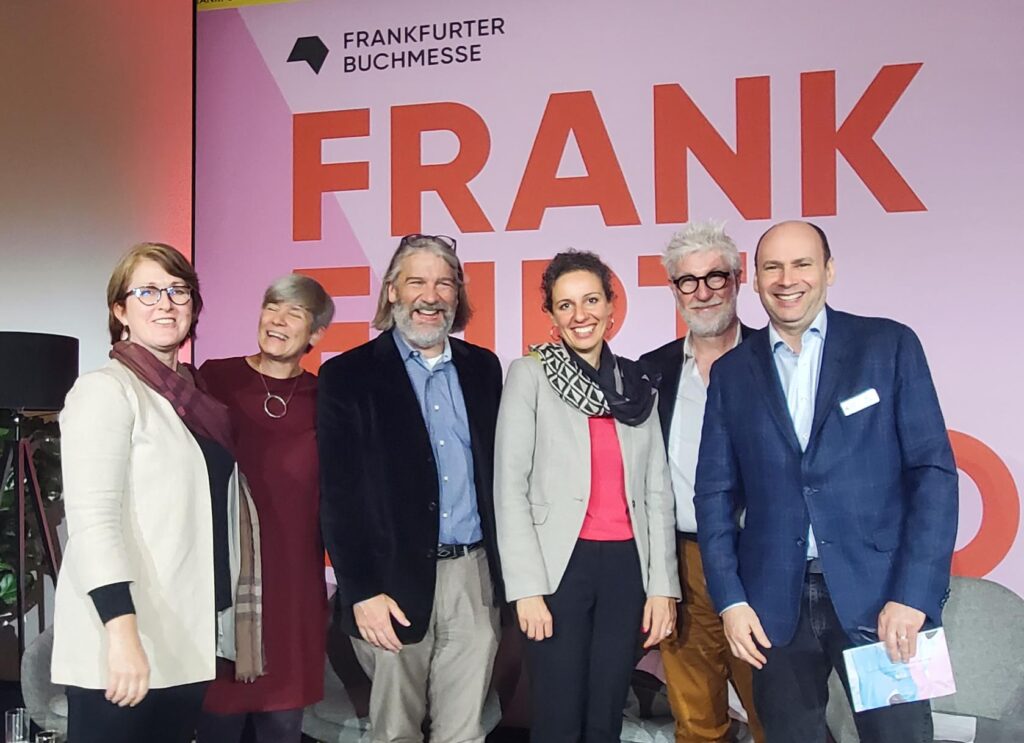
I had the honor of moderating this lively conversation with fellow Chefs Roy Kaufman and Robert Harrington, along with guests Leslie McIntosh, Anita de Waard, and Julia Kostova. Unfortunately, Chef Avi Staiman couldn’t join us because of the tragedy in his home of Israel and the ongoing situation taking place as a result. In the short time we had, we covered a lot of conceptual ground, extending from copyright implications around open content to how generative-AI models affect concerns around authorship, and how models consume open science content to how AI is challenging our notions of accountability. If you’d like to watch a recoding of the panel, you can do so on the Frankfurt Messe Youtube channel.
How to (or not to) train your model
At the moment, there is a fascinating interplay between open content and the development of AI tools on the one side and the implications for creation and use of open content on the other. As Kaufman pointed out during the panel, part of the increasingly established definition of open content is focused on the license and copyright sharing framework of that content. Meanwhile, many copyright and licensing questions are the subject of several lawsuits and growing regulation — at least internationally regarding copyright — to deal specifically with AI’s uses.
This has led some of the people developing large language models (LLMs) to train their models on open content, of which there is now a significant and rapidly growing corpus. It should be noted that many organizations have apparently trained their models on publicly available content, which likely is not covered under a Creative Commons license, or even proprietary content that isn’t generally publicly accessible, hence the lawsuits. Other openly licensed content, such as datasets of various types, can also serve as additional training resources. As was noted, it is in everyone’s interest to be training these important models with trusted and vetted scholarly content rather than “just anything a model might find on the internet”, where the content can range from dubious, to potentially questionable, or even intentionally incorrect.
Positive uses of AI for open content
Whereas open content can play an important role in the creation of LLMs, these tools also hold significant potential to accelerate and advance open science, as Kostova stressed. AI-based tools are already widely adopted and used in various domains of science, allowing researchers to more accurately and more rapidly generate scholarly output. We must remember that while at the moment much of the attention is focused on generative models like ChatGPT, BARD and LLaMa, many domains are using the same types of artificial intelligence computational models to review medical images, scan through space image data, and develop meteorological models. Similarly, computational models are supporting discovery, navigation, and analysis by creating new paths for semantic understanding and novel advances of science in biological sciences, in chemistry, in genomics, and many other domains. Using machine-generation tools could also provide significant opportunities to engage the wider community, either through translation or connecting with those outside of the core of scholars engaged in the work, which is another mission of most scholarly societies, noted Harrington. He also noted several ideas he’d covered in his recent piece on the topic, on how AMS is considering incorporating AI-based tools in their editorial and educational outreach. While wariness is warranted, we shouldn’t view these new tools as being existentially bad and the disruption as being entirely negative.
We want to trust our AIs, but how?
On the side of integrity, there were lively thoughts as well on the potential opportunities and challenges that flow from increased use of AI-based tools. Beginning with the question of why the issue of integrity is so critical to scholarly communications, the conversation quickly turned toward how AI tools provide both opportunities and challenges. As McIntosh pointed out, there are ample amounts of free water in most parts of the world. “I can walk you down to the Main [River] and hand you a glass of free water, but would you drink it?” It is the quality and integrity that makes you trust the water you pour from a tap, but that you should be reticent when it comes straight out of a river flowing through an industrial city. The same should be true of scholarship, McIntosh stressed. de Waard built upon this, reflecting on the role of standards in developing new frameworks for sharing digital scholarship and how each of the elements of a scholarly work fit together to form a cohesive whole. AI tools can help discover, navigate, and understand this complex ecosystem.
Similarly, though there are risks inherent in any technology noted McIntosh. For each positive use of a technology there are concerns about its misuse, in paper mills or in image manipulation. McIntosh described the work that Ripeta has done identifying a network of potential trust metrics associated with a paper using AI-based tools that are calculated based on trust metrics and have been applied to millions of objects. Elsevier similarly has deployed other AI tools to assist in the editorial and production processes. Once these tools are implemented, are there ways that this information can then be shared along with the content, noting what types of integrity checking has been undertaken. Here, de Waard noted a technical standard recently published by NISO, the Content Profile/Linked Document specification, which is meant to support such communication. In the end, the single most important thing that we do (i.e., the scholarly publishing industry) is to confer trusted information to subsequent generations. It is therefore vitally important that we use every tool in a sensible way to ensure the record remains viable and trustworthy as we can.
Where can we go from here?
As the session closed, I inserted a point at the end of the session about the role of standards and trust, building upon de Waard’s comments. During the NISO Plus Forum focused on Artificial Intelligence applications in scholarly communications that my organization hosted earlier this month, participants identified a number of potential projects that we could try to encourage in our community. More than half of the potential project ideas could be broadly conceived as addressing issues around integrity and trust.
For example, one of the project ideas involved extending the CReDIT terminology to include machine generation of the content. Two additional ideas involved the inputs in generating machine outputs using AI-related tools. The first was on the trustworthiness of the data that is used to develop a model, such that it can be trusted in terms of quality, bias, or scientific rigor. Another idea was focused on the assessment of a model and its application for a specific purpose. Similar project ideas have been suggested for engineering issues, but not specifically around scholarly communication issues. There were more suggested ideas than NISO could ever pursue and develop on our own. We hope that when the report of the Forum is circulated, members of the community will engage with our efforts to ensure reasonable, equitable, and inclusive use of AI-based tools moving forward.
Perhaps it is too soon to fully understand the full implications of these new tools, but there are many lenses to view the challenges and opportunities. Ideally, community conversations like this one hosted by SSP’s Scholarly Kitchen will help people to grasp the potential impacts on their organizations and the scholarly community at large. Hopefully, those engaged in various elements of these various worlds will work together to explore innovative approaches to applying these tools in our community. There is certainly a lot to explore, lots to discuss, and a lot to implement.
A recording of the Frankfurt Studio Scholarly Kitchen session is available.
Discussion
1 Thought on "Chefs Panel Discusses AI, Integrity and Open Content in Frankfurt"
Yes, it is too soon. And the machine learning used in Healthcare shouldn’t be compared to the ‘black boxes’ that are the chatbots. I would recommend against using the latter for anything serious(ly life threatening) 😉