At the start of the year, our fearless leader and Executive Editor David Crotty, wrote an excellent and thought-provoking article where he correlated a sudden step jump in the perceived rate of change in our previously quiet bit of the information industry to two factors; industry consolidation and regulation. Do read it again.
This year, I’ve been pondering how best to take advantage of the mind-blowing array of tools and toys available to the aspirational technologist, and as David talks about in his article, it feels a bit like the options and increments are all happening at once. As he observes, whilr you can certainly get someplace much much faster, it can be equally as hard to apply the brakes, and certainly in technical terms, it can be rather expensive to do so if you end up getting too excited about something that doesn’t pan out in the end.
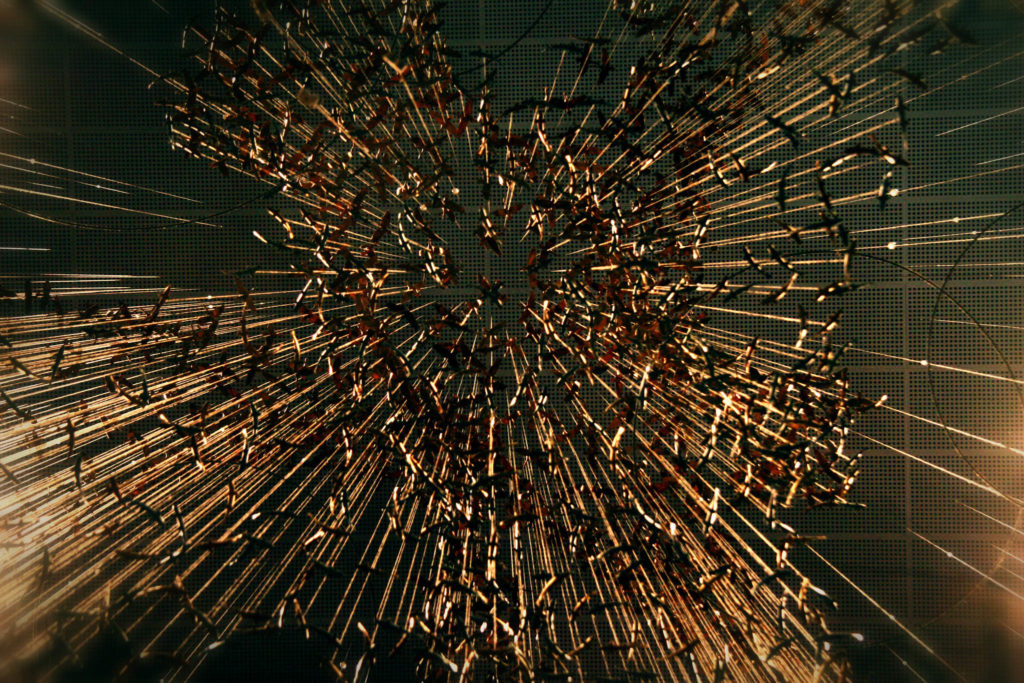
For me, the great acceleration is being experienced most directly by the ability to tap into insane amounts of compute capability available from (mainly) Amazon and Azure — the main cloud compute suppliers. About 15 years ago I had a conversation with a Google person who was lamenting that even though they had basically infinite compute capability available to them, they still had to go to the trouble of putting a business justification in to use it — oh the horror. I was suitably sympathetic.
Fast forward to now, and that infinite capability is available to all of us, so long as we can put forward a suitable justification. You can now spin up well — just about whatever you want. Say you have a bunch of data you need to chew through — well if there’s enough of it, a cloud provider can send you a fancy hard-drive if needed — you plug it in, it slurps your data, you have it delivered to your cloud provider and then you click a few buttons in a web browser and you’ve got yourself a high performance data munging monster, all for a few hundred £ or $ (do remember to turn it off when you’ve finished).
Or perhaps you want to build some sort of AI thing. Well you can, pretty quickly, and pretty cheaply. And people are. And a lot of them are very bad indeed. This is worth considering carefully. Our new power brokers are trying to lower the barrier to the uptake of most of their tools, but particularly AI and machine learning ones, by making it something of a point-and-click type operation. Amazon offers pretty much this, and there’s a thriving marketplace with all sorts of ‘solutions’ to your AI problems. This includes some pretty alarming offerings that will allegedly proof your shiny AI thing from bias, by the application of, well in this author’s opinion, a whole lotta woo (so I haven’t linked to them, but check out an AI tech talk or two, you’ll see them). There’s example after example of ‘off the shelf’ algorithms connected to some data from somewhere, promising all sorts of things. The healthcare field is particularly awash with such things. Take five minutes, visit this site, I’m fairly sure it will shock you with its capabilities.
I bet there are some of you reading this article who are looking simultaneously at how they can leverage AI things into commercial offerings, and simultaneously consume other AI things for efficiencies and so on. Perhaps the AI that can do the first stage interviews for prospective employees, a sort of inverse Voight Kampf test as it were. Now, your organization has got training in the application and ethics of AI in the workplace right? You’ve got a policy on your AI ethical stance yes? I’m not being flippant.
Compute is the power supply of the information age. The raw power has finally caught up with the thinking of 30 – 40 years ago. When the power resource is no longer the constraint — and in this new world, it simply isn’t now, what you get is a revolution. You can, for example, figure out what a black hole should look like, and then run simulations in order to figure out which sim best matches the data you have — and then you have your first picture of a black hole.
The skill sets are now the scarcity. You will remember what happened when we all got access to ‘desktop publishing’. Imagine a world of ‘desktop AI’. It’s coming. I’m betting a couple of years from now there will not be an operating system that doesn’t come packed with a whole suite of AI tools, ready to be deployed. You’ll even be aware of some of them.
And this matters, because a 21st century scholarly infrastructure should be leveraging this capability and I don’t think we are — not really. And it’s not just AI. We aren’t really leveraging how very large datasets could be used. We aren’t leveraging scale and resilience. There’s a big conversation to be had here about what the structural building blocks of a true scholarly infrastructure should be, and how the dissemination and verification and classification and curation and ethics should be.
Candidly, this year I’ve been rather disappointed by the reveal of the various Open Source toolkits out there. They still need a lot of work before most organizations could consider using them in anger (pull up a chair, lemme talk to you about charitable procurement policy…) and there are serious concerns I have about sustainability. The goals are laudable, the challenges in building communities of use, formidable. But the thing that does seems to be missing, is the issue of what scholarly infrastructure ought to be enabling.
I recently sat on a panel about replication and reproducibility (some very exciting start-up work in this area, all chasing funding and early investment customers) and it was apparent that if this matter is to be worked on systematically, then a rethink of the way scholarly outputs are put together and used and queried and assembled is needed. Perhaps we could take inspiration here from other places (for example, the BBCs work on object oriented media is most fascinating, with all sorts of parallels to these eyes). Wouldn’t a ‘world of scholarly content built just for you’ be the ticket here? Subject to funding of course.
And there’s another thing accelerating towards us at frightening speed. Climate Change and what it is that WE are going to do in mitigating its effects. In the twenty years I’ve been in scholarly publishing, the CO2 level has gone from 369ppm to 410ppm. The goal is to try and stop it at 450ppm. We have maybe twenty years. I look at the all the cool things I’ve done with the power at my command over the last few years, and I wonder what the carbon burden is of the tech my team has built. It’s rather difficult to figure the reality out as it happens. Something to think about over the holidays, and after.
Discussion
6 Thoughts on "Revisiting The Great Acceleration: A Technology Perspective"
Re carbon burden, https://www.websitecarbon.com/ is fun to play with. Questionable accuracy but it makes the impact tangible. When you multiply up from the 10,000 monthly page views the calculations are based on publishers need an awful lot of trees to absorb the carbon we’re creating!
Thanks Helen, I’m currently poking around the multitude of carbon calculators and the variety of answers you can get shows that this is a real problem. To do something to bend the curve, one needs reliable ways to figure out where one is starting from.
David,
I completely agree with your statements:
“And this matters, because a 21st century scholarly infrastructure should be leveraging this capability and I don’t think we are — not really. And it’s not just AI. We aren’t really leveraging how very large datasets could be used. We aren’t leveraging scale and resilience. There’s a big conversation to be had here about what the structural building blocks of a true scholarly infrastructure should be, and how the dissemination and verification and classification and curation and ethics should be.”
“a rethink of the way scholarly outputs are put together and used and queried and assembled is needed”
Moreover, research scientists in many areas are measuring and experimenting in a manner which is primarily that which they need for publications. Few are trying to make data more useful, more available and or measuring and reporting the data which is needed if we are going to use AI/ML. In some areas it is effectively meta-data that is being reported and stored (e.g. the way chemical structures are generally stored for organic chemistry/pharmaceuticals etc.) or often an insufficient range of data over an insufficient number of variable parameters is measured.
Dear David: I’m such a luddite I didn’t understand more than 20 words of your article. I would agree with you that the acceleration of computing is mind-boggling. But I work in a library. Part of our traditional role is conservatorship of primary source documents. Your thoughts on protecting tradition while embracing modernity?
Hi Melinda, well I certainly hope no one is foolish enough to threaten the physical manifestation of knowledge that is the library. One thing that I think is a problem is the fragility of the bits that make up the digital objects that we store and manipulate. It’s not only the issue of backups and such like, its the issue of files and the programs and operating systems needed to run them, even the hardware… Perhaps you need to become conservators of those things as well.
Thanks for your thoughtfulness about this crucial issue.