At the recent Researcher to Reader conference in London, Mark Allin (@allinsnap) had the job of doing the conference round-up, which is the slot immediately before the closing keynote where the themes and take-homes of the conference are brought together. In his four summary themes, Allin inevitably drew out Open Access / Open Science. It’s almost impossible to have a publishing or library conference without it, however, in terms of significance, he put it at the bottom of the list, almost as an afterthought. His reasoning is that open science now feels like an inevitability. With a clear trend towards both open access and open data mandates among funders, institutions, and publishers, the question that each of us must ask ourselves isn’t whether it will or should happen, but how are we going to adapt as change continues.
This post isn’t about Researcher to Reader. If you’d like to read about the conference, check out the hashtag (#R2Rconf). It was a lively one, I promise you. Instead, I’m going to write about the meeting I attended the day after. This meeting was about what some publishers are doing to respond to and support open research, or more precisely, open data. The STM Research Data Workshop series, facilitated by Joris van Rossum and Fiona Murphy, of which last week’s meeting was the second, is part of the STM Association’s Research Data Year (RDY). The RDY, whose theme is Share-Link-Cite, is a collaboration of 11 publishers*, which according to Rossum — who is STM’s research data director — publish over half of all journal articles. The workshop was attended by select representatives from member publishers and technology organizations important to the effort including SCHOLIX, Crossref and Ripeta.
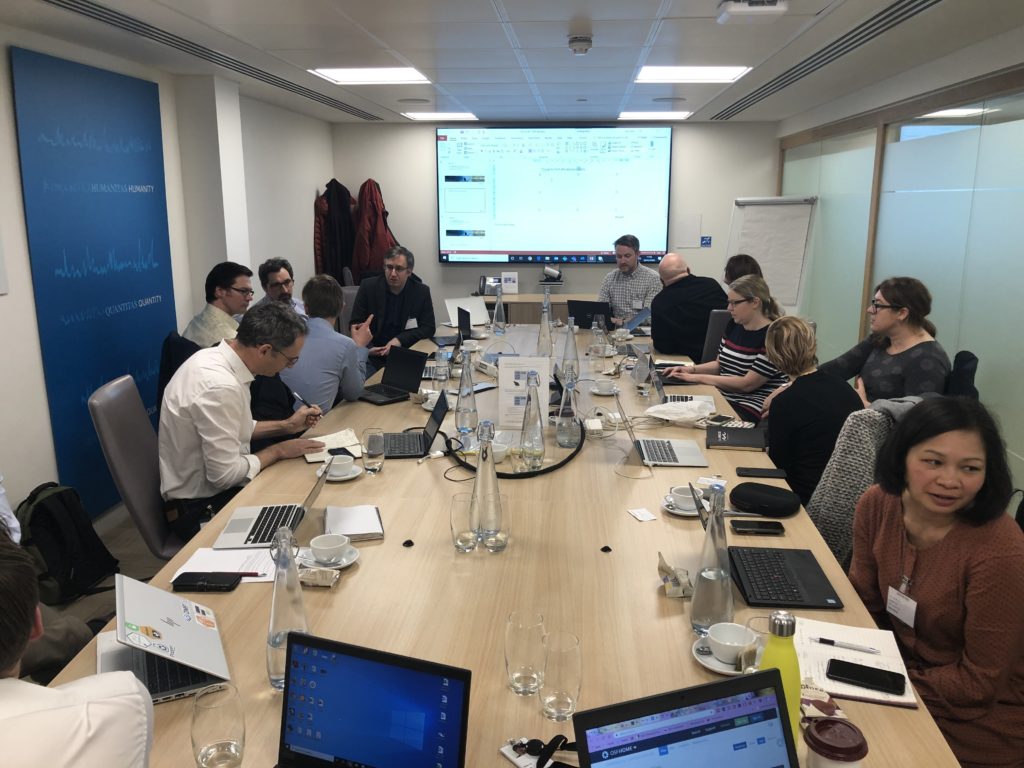
The rise of data and the data policy
Practices around open research data are gaining traction. In 2019’s The State of Open Data Report, 64% of respondents claimed that they made their data openly available in 2018. That’s a rise of 4% from the previous year. Comprehensive information on the prevalence of open data policies is hard to come by, but there is a general sense that publishers, funders, and institutions alike are all moving towards firstly having data policies and then steadily strengthening those policies over time.
The JoRD project, based at Nottingham University in the UK was funded by Jisc and ran from December 2012 until its final blog post in 2014. In this article, Sturges et al., report that JoRD found the state of open data policies among journals to be patchy and inconsistent, with about half of all the journals they looked at having no policy at all, and with 75% of those that did exist being categorized as weak.
Unfortunately, the short timescale of the JoRD project limits its findings to a snapshot. However, there has since been piecemeal evidence of progress towards a more robust open research data landscape. The case studies presented in this article by Jones et al., — a different Jones, not me — describe how both Taylor and Francis, and Springer Nature have followed the path of steadily increasing the number of journals with data policies while strengthening those that exist. Like all of the publishers that were represented at the STM workshop, both Taylor and Francis, and Springer Nature use multiple progressive levels of policy, from broadly advisory to firmly mandatory in terms of the need to share data as well as how to share it.
With the level of socialization of open data practices varying over discipline, geographical region, and community, there’s no one-size-fits-all data policy that journal editors should adopt or publishers should mandate. Among some communities, in the humanities for example, there isn’t much data to be shared and when there is, the social norms and mechanisms may be in their infancy or not developed at all. There are also communities that have significant portions of their data that can’t be shared. Being clear on the limits of openness, for example in order to respect patient confidentiality, the locations of endangered species, or commercially sensitive data is critically important.
The lowest levels of policy can be as lightweight as requesting that authors provide a data availability statement (DAS) where they state whether they will share their data. Stricter policies can require a DAS without mandating sharing, require sharing in general, the use of specific approved repositories with particular data types conforming to specific structures and standards, and most strictly, peer-review of data, whatever that means.
Guidelines and structures
Those who follow trends in open data will be aware of the Transparency and Openness Promotion (TOP guidelines). For data sharing, TOP describes four levels:
- Not implemented: Journal encourages data sharing, or says nothing.
- Level 1 – Disclosure: Article states whether data are available, and, if so, where to access them.
- Level 2 – Mandate: Data must be posted to a trusted repository. Exceptions must be identified at article submission.
- Level 3 – Verify: Data must be posted to a trusted repository, and reported analyses will be reproduced independently prior to publication.
Many publishers have taken these as a starting points for policies, although none have gone as far as requiring independent reproduction of analyses, which is arguably out of scope for traditional scholarly journals and would require significant infrastructure investment. It’s important to bear in mind that TOP is about a lot more than open data and as a result, its view of open data is fairly high level, and constitutes a set of principles. Those principles can and should be interpreted in a community specific way, but at the same time, it’s important to limit complexity so as not to overburden editors and authors.
It’s a tricky balance.
The Belmont Forum, which represents a group of 26 funding agencies, ratified a DAS Policy and templates document in 2018 that articulated a set of minimum requirements for a DAS. These included confirmation of the existence (or not!) of the data, preferring the use of persistent identifiers rather than URLs where available, and providing licensing information. More recently, the Research Data Alliance (RDA) have sought to address the need for clarity and consistency by creating a specific framework for data policies based on a harmonization of existing publisher policies. A number of funder policies, the CODATA best practice guidelines, and the TOP guidelines were also referenced. The rubric, shown below consists of 14 policy features with 6 policy levels that gradually increase the number of included features.
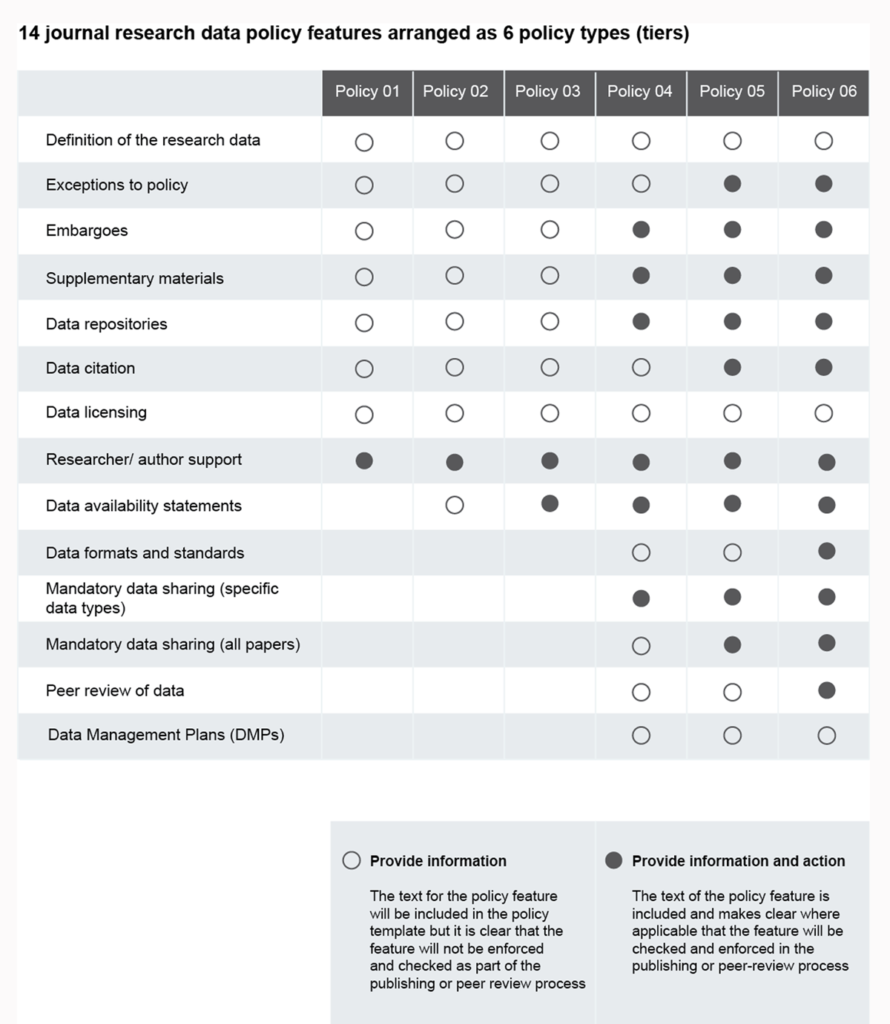
What’s next on the road to open data
Some great progress has been made towards a more robust, consistent, and less confusing open data landscape, but much more needs to be done to make things more accessible to researchers, editors, societies and many smaller publishers.
The identification of the need for a framework that caters to different levels of community development towards openness is an important step forward, but such frameworks are currently still being developed and socialized themselves. The RDA framework was discussed at the workshop and agreed to be on the right track, but attendees weren’t quite ready to adopt it as a standard before exploring how it maps to the TOP guidelines, and their own specific policies. Nevertheless, we seem to be on the road to clarity and standardization.
Meanwhile, many publishers are continuing to work with their editors and communities to both socialize open data and steadily roll-out more and stronger open data policies as appropriate.
Some longer term thoughts
Many data policies from publishers and funders, including the European Commission, make reference to FAIR data. While open and FAIR are connected concepts, they’re not synonymous.
For instance, corporate R&D divisions and knowledge managers are increasingly using FAIR principles to accelerate discoverability of internal, proprietary datasets that are not intended to be shared outside of the company research environment.
By the same token, placing a dataset on a publicly accessible personal website with no supporting metadata may be open, but it wouldn’t be findable, and if not structured correctly, may not be interoperable or reusable.
In many ways, FAIR data is a more complicated problem to solve than openness. At the workshop, there was a sense that editorially policing that data referenced in the article is accounted for in the DAS and actually exists is time consuming and expensive. Making sure that the data is structured correctly, with the right metadata, requires a lot more time and domain knowledge.
At the workshop, Leslie McIntosh from Ripeta discussed their work with natural language processing, which analyzes manuscripts with a machine identifiable DAS definition to validate its presence. Eventually, similar technologies might help editors assess compliance with data policies.
Beyond the DAS, there’s a challenge around specifying and certifying the correct structures for data types in the first place. There have been some great early successes in areas like Genomics and the environmental sciences, where big, well-structured data sets are the norm. As we move into the very long tail of data types — which stretches all the way down to one-off experiments and everything in between — there are open questions of how far down that long tail publishers can helpfully go and where the resources are going to come from to create and enforce the thousands of data structures that will be needed.
It’s clear that open data, and its close cousin FAIR data are both important challenges for the scholarly communication infrastructure. At the 2nd STM Data Workshop, I was impressed by the clarity of thought that had gone into understanding this thorny set of problems and by the practicality of some of the solutions put forward.
There’s a lot that publishers can already do, at the very least to help socialize the idea of open data within their communities. The lightest policies can be purely advisory with the next step up being just to mandate the existence of a DAS. While that lowest level might not require any greater openness on behalf of researchers, at least it can let people know that such things exist and that the editor values them. The various resources I linked to above are well worth a read, but if you’re a publisher looking for a very quick primer, the data availability tips from STM are a good place to start.
Thinking more long-term, there’s still a lot of work to be done to make open data requirements both flexible enough to suit communities and consistent enough to not be confusing. There’s also a need to address sustainability, as it seems that with current approaches, policing stricter mandates would be unsustainably expensive, if it were done by all publishers for all research journals.
Despite these challenges, progress continues to be made. As Allin said, openness feels inevitable. It will be interesting to see how we get there.
*Publishers participating in the STM Research Data Year:
- Cambridge University Press
- De Gruyter
- Elsevier
- Emerald Publishing
- IOP Publishing
- Karger Publishers
- Oxford University Press
- Sage Publishing
- Springer Nature
- Taylor and Francis
- Wiley
Discussion
6 Thoughts on "Is it Finally the Year of Research Data? – The STM Association Thinks So"
Thanks Phil. I am hoping to hear that RDA plans help with standard approaches.
Hi Valerie,
I believe that RDA have a big role to play in advancing and standardising research data standards.
The thing that we need to avoid is fragmentation in our approaches. There are many initiatives going on at the minute in FAIR data, open data, and the related issues around research evaluation. The importance of cross-stakeholder collaboration is more important than ever.
Hi Phill, thanks for the interesting post. This sentence stood out for me:
“With the level of socialization of open data practices varying over discipline, geographical region, and community, there’s no one-size-fits-all data policy that journal editors should adopt or publishers should mandate.”
I think we need a distinction between the field-independent and relatively objective gold standard for open data (and open data policies), where all the data required to reproduce the results in the article are publicly available, and the progress towards achieving that standard, which varies massively by research area (as you note).
To use a cycling analogy: even if some fields are still struggling to get onto their tricycles, everyone has the same goal of being riding around on a big kid bike. The idea that some fields are best off sticking with stabilizers for the foreseeable future does them, and open data, a disservice.
Hi Tim,
You raise a very interesting point.
I agree that it’s important to separate the degree to which different disciplines and communities have gotten on board with the ideas and practices of data sharing and open data from the gold standard of practices.
That said, I’m not sure I agree that best practice is field independent. Research outputs and data types vary enormously across disciplines. In some disciplines, data is straightforward are essentially relatively small lists of numbers, in others, you need independently funded data centres if you’re going to store raw data because there’s just so much of it. Some fields almost exclusively use accepted experimental techniques with standardized outputs that can be put into structured databases. In others, they’re designing and adapting new technologies to the extent that standardisation wouldn’t be appropriate or even possible.
I think the popular definition of ‘all data required to reproduce the results’ is a bit of an oversimplification. If I think about the experiences of the researchers that I know, and to my own experiences, that sentence could be interpreted in many different ways. Thinking about one experimental method I used, my data went through multiple stages. Raw, processed, analysed, curated and publication ready. Which one of those is needed to reproduce the findings in the paper? You could redraw the graphs based on the analysed data, but what about the processing steps? How would that be validated? Maybe it’s the raw data that should be shared? That was about 100Tb per experimental run, and needed high performance computing resource to process and reduce, which means it wouldn’t be very reusable to a wet lab biologist that does their analysis on a laptop using SPSS. That’s before we get into what data means in many under-discussed areas of humanities and social sciences.
Research is diverse and complicated. None of these problems are insurmountable. I think it’s possible to create general guidelines that can be interpreted and operationalised by individual communities. That’s what I meant by the impossibility of a one-size-fits-all policy.
Great summary!
Two points that I would like to comment on: “Many publishers have taken these as a starting points for policies, although none have gone as far as requiring independent reproduction of analyses, which is arguably out of scope for traditional scholarly journals and would require significant infrastructure investment.” While true that these computational reproducibility checks require investment, I’d like to point out that this is indeed being taken up. The American Economics Association, for example, has dedicated resources to this workflow https://www.aeaweb.org/news/member-announcements-july-16-2019 and there are a total of 15 journals, to our knowledge, that implement this workflow (sort by “Data Transparency” at http://www.topfactor.org to see these “Level 3” data policies).
“The RDA framework was discussed at the workshop and agreed to be on the right track, but attendees weren’t quite ready to adopt it as a standard before exploring how it maps to the TOP guidelines, and their own specific policies.” I agree that a bit more clarity would be helpful here, we can work with the members of that group to clarify how these correspond to TOP (see for example how TOP maps with various publisher policies here https://cos.io/blog/landscape-open-data-policies/ ). To start off this mapping, let me point out that the RDA Policy 3 requires data availability statements (which complies with TOP Data Transparency Level 1) and Policy 5 requires all underlying data to be made available (TOP Level 2). Policy 6 may, in some cases correspond to Level 3 if peer review requires reproducibility checks.
Hi David,
Thanks for that very useful comment. I’ll have to take a look at what the AEA are doing in the area of independent validation. A few publishers I spoke to were surprised by the idea of doing independent validation of data analysis prior to publication, which was where my statement came from about it being arguably out of scope. That said, it’s not that long ago that many publishers considered involving themselves in research data at all to be out of scope. I’ll be interested to see how that approach develops and how broadly it’s adopted.
The RDA framework mapping is very useful information and I’m sure the folks from the STM year of research data will find that useful. It’s really important that we don’t end up with multiple standards and frameworks that end up adding to the confusion.