Editor’s Note: Today’s post is by Mark Hahnel. Mark is the CEO and founder of Figshare, which currently provides research data infrastructure for institutions, publishers, and funders globally.
There has been much made of the recent Nature news declaration of the NIH Data Policy (from January 2023) as ‘seismic’. In my opinion, it truly is. Many others will argue that the language is not strong enough. But for me, the fact that the largest public funder of biomedical research in the world is telling researchers to share their data demonstrates how fast the push for open academic data is accelerating.
While a lot of the focus is on incentive structures and the burden for researchers, the academic community should not lose focus on the potential ‘seismic’ benefits that open data can have for reproducibility and efficiency in research, as well as the ability to move further and faster when it comes to knowledge advancement.
What has been achieved in the last ten years?
My company, Figshare, provides data infrastructure for research organizations and also acts as a free generalist repository. We recently received funding as part of the NIH GREI project to improve the generalist repository landscape and collaborate with our colleagues at Dryad, Dataverse, Mendeley Data, Open Science Framework, and Vivli. This community of repositories has witnessed first-hand the rapid growth of researchers publishing datasets and the subsequent need for guidance on best practices.
The growth in citations from the peer-reviewed literature to datasets in these repositories can be seen in this hockey-sticking plot from Dimensions.ai. Remember, this is just the generalist repositories; it doesn’t include institutional or subject-specific data repositories.
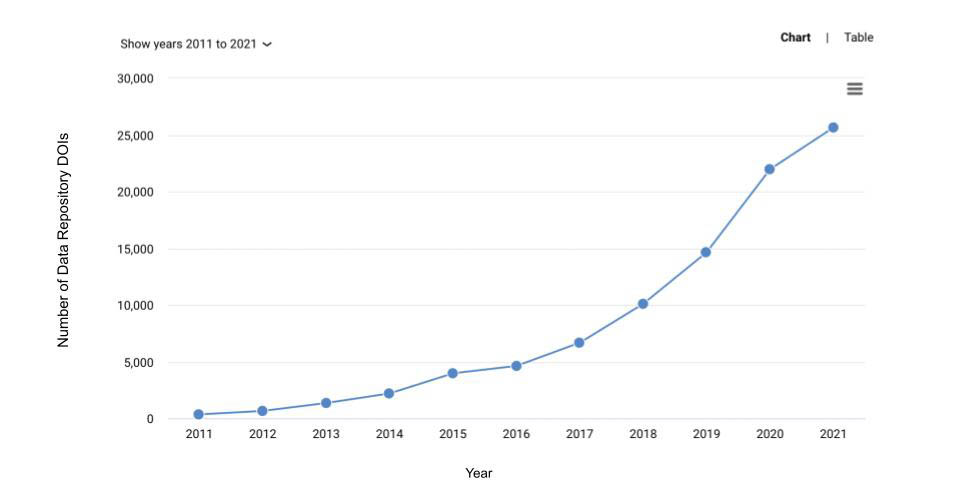
Reflecting on the past decade of open research data, there are a few key developments that have helped speed up the momentum in the space, as well as a few ideas that haven’t come to fruition…yet.
The NIH is not the first funder to tell the researchers they fund that they should be making their data openly available to all. 52 funders listed on Sherpa Juliet require data archiving as a condition of funding, while a further 34 encourage it. A push from publishers has also acted as a major motivator for researchers to share their data. This goes as far back as PLOS requiring all article authors to make their data publicly available back in 2014. Now, nearly all major science journals have an open data policy of some kind. Some may say there is no better motivator for a researcher to share their data than if a publication is at stake.
In 2016, the ‘FAIR Guiding Principles for scientific data management and stewardship’ were published in Scientific Data, and a flurry of debate on the definition of Findable, Accessible, Interoperable, and Reusable data has continued ever since. This has been a net win for the space. Although every institution, publisher and funder may not be aiming for the exact same outcome, it is a move to better describe and ultimately make data outputs usable as a standalone output. The principles for Findable, Accessible, Interoperable and Reusable data emphasize that when thinking of research data, future consumers will not just be human researchers — we also need to feed the machines. This means that computers will need to interpret content with little or no human intervention. For this to be possible, the outputs need to be in machine readable formats and the metadata needs to be sufficient to describe exactly what the data are and how the data was generated.
This highlights the area (in my opinion) that can create the most change in the shortest amount of time: quality of metadata. Generalist repositories will always struggle to capture metadata at the level of subject-specific repositories. This is why subject-specific repositories should always be a researcher’s first port of call for depositing data. It is unlikely, however, that we will see a subject-specific repository for every subject in the next decade. What we will see is a multi-pronged push for better metadata for every dataset. This can be achieved in multiple ways:
- Software nudging users into best practice. A simple first step is to encourage researchers to title their dataset as they would a paper. Hint: titling a dataset “dataset” is as useful as titling your paper “paper”
- Institutional librarians being recruited to curate metadata for outputs before they’re published
- More training for academics on the benefits of making their data more discoverable by making it more descriptive. More discoverable in theory means more potential for reuse and more impact for the researcher — the biggest incentive of all
- Services offering curation. Dryad have been doing this for a decade and we are beginning to see a wider variety of solutions available for a variety of data
- Marking up existing metadata using related information openly available on the web.
For publishers, there is a huge opportunity to aid the researchers in data publication. Most policies require data publication at the point of publishing the associated paper. While the paper will always be the context and interpretation, the machines need metadata around the objects sourced either from the papers directly — meaning linkages between the two are of the utmost importance — or encouraged by editorial staff before the outputs are made public.
Where else is there work still to be done?
One area where progress hasn’t been made significantly is the publication of negative results, or null data. Providing tools for researchers to make all of their academic outputs openly available is just half of the story. For the most part, researchers have zero incentives to publish negative results.
And while the number of researchers sharing data is growing rapidly, this does not mean that researchers want to do so. Evidence from The State of Open Data, suggests that the majority are publishing data for compliance reasons. 39% of researchers surveyed said they are not receiving appropriate credit or acknowledgement. 47% of survey respondents said they would be motivated to share their data if there was a journal or publisher requirement to do so. This lack of incentives, combined with fear over being scooped, may also be responsible for a recent spike in the amount of researchers citing “data available upon request” in their articles; this is something I consider to be bad practice.
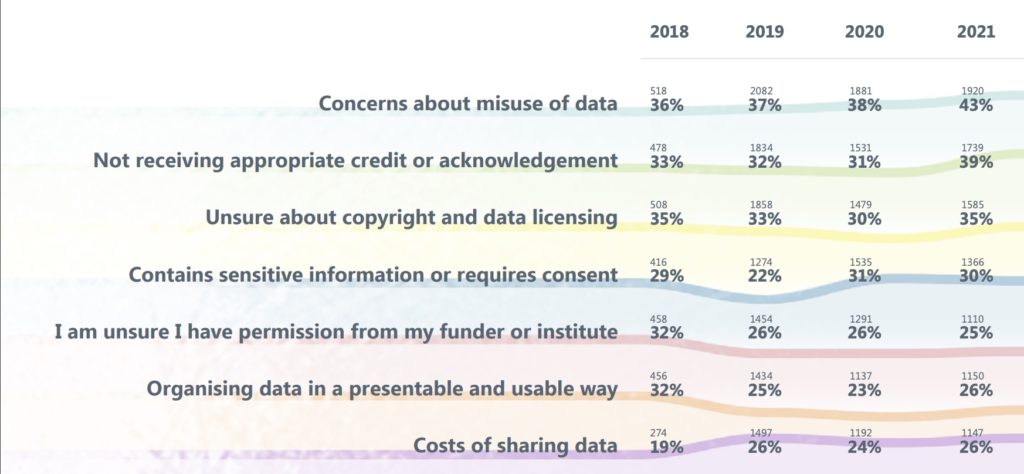
What happens next?
If the last 10 years were about encouraging researchers to make data available on the web, the next 10 years should be about making it useful. The concept of a Fourth Paradigm in academic research is envisioned as a new method of pushing forward the frontiers of knowledge, enabled by new technologies for gathering, manipulating, analyzing, and displaying data. The term seems to have originated with Jim Gray, who contributed to the publication of The Fourth Paradigm: Data-intensive Scientific Discovery in 2009 but sadly is no longer with us to see how his predictions have come to fruition.
I have spent a large part of the last decade hypothesizing what the benefits of open data could be in accelerating the rate at which information becomes knowledge. We are beginning to see real world examples, such as AlphaFold, a solution to a 50-year-old grand challenge in biology. A core part of this success story relied on AI training data from the Protein Data Bank — a repository that itself is over 50 years old — pulling together homogenous datasets, ideal for AI and machine learning. The associated quote on publishing the findings from Deepmind also highlights how a combination of well described open data and AI could achieve the lofty goals set out in the Fourth Paradigm:
“This breakthrough demonstrates the impact AI can have on scientific discovery and its potential to dramatically accelerate progress in some of the most fundamental fields that explain and shape our world.”
As such, well-described, open data is primed to accelerate the rate of discovery by providing fuel for our machine overlords to crunch through. The sheer size and volume of the data may continue to outpace the ability to store and query academic outputs in meaningful ways, even more so if we don’t get the fundamentals around subject-specific community best practices in place today. Machine learning and AI can help look for patterns and relationships in the data; that will always be beyond the realms of human endeavor. Human comprehension of these results will always be needed to push the needle further still, much in the same way that a combination of humans and machines proved to be the sweet spot in competitive chess.
As we nurse our COVID hangover, the world has never been more aware of the need to move further faster when it comes to knowledge discovery. The missing puzzle piece to achieve this in the traditional academic publishing process is well-described open data. The effects could be ‘seismic,’ some may say.
Discussion
9 Thoughts on "Guest Post: A Decade of Open Data in Research — Real Change or Slow Moving Compliance?"
Kudos for the spot on post and for your work at figshare.* As one who has proselytized for open data in my field (pollution science) to little avail, I think if open data practices are to spread, it will be from top down pressure from journals, institutions, or funders. As your chart shows, there’s too little incentive and too much hassle for many researchers to willingly take this on. I’d also add lack of training in data management best practices for researcher as a factor that both increases the lift for researchers and reduces the interoperability of the data.
My (occasionally persuasive) arguments have appealed to self-interest, such as 1) if you post your data, your study is more likely to be meaningfully incorporated into syntheses or comparative studies which will boost the influence and exposure of your work; 2) the scientist most likely to benefit from your data archiving is your future self: how many a server flushes, cloudbursts, computer replacements, office downsizes or moves, or maybe employment shifts might come in the next 10-15 years?, and 3) where will your data go when you retire?
Still, it mostly falls on deaf ears. Academics are the worst. The coin of their realm is publications, citations and funding. Government scientists, such as myself, are pretty good because it’s required and beaten into our psyche. Industry scientists in my field are pretty good because they’re often publishing with regulatory interest and to build a case with regulators, they need to show their work.
* I’m a fan but I worry whether figshare and similar services will continue to have legs as a data sharing and archiving platform. How does something free plan to last forever?
Just to answer the last question about something free lasting forever. Our sustainability model involves providing data publishing infrastructure for organisations, such as University College London, the Department of Homeland Security and PLOS (and 100s of others).
To my mind FAIR is somewhat orthogonal to promoting researchers to share the data from their articles: an author can make a dataset perfectly FAIR and still have their article be completely irreproducible by (for example) withholding ten other datasets. FAIR is a great answer on ‘how’ to share data, but it doesn’t have much to say about ‘what’ data should be shared. For the latter, we need to take each article by itself and establish for both the authors and the stakeholders which datasets need to be made public and follow up when they’re not available.
In the US the White House OMB Circular A-110 defines data as “The recorded factual material commonly accepted in the research community as necessary to validate
research findings.” Does not include null data.
People using the “data available upon request” line is a holdover from the FOIA amendment (1990s) stating that if research is funded by the US government, then it must be saved for a minimum of 3 years and made available to anyone upon request. FOIA is federal law, I don’t think the mandates from the agencies are law.
Excellent article. You and other readers might like to read the recent report from the Australian Academy of Science entitled “Advancing Data-Intensive Research in Australia”. It’s available at https://www.science.org.au/supporting-science/science-policy-and-analysis/reports-and-publications/advancing-data-intensive-research-australia. While focussed on Australia (and with Australia not as advanced as other nations) its findings and recommendations are universal. Your comments are fully aligned. While policies matter, the critical issue is researcher behaviour as you make clear. That has to change!
Thanks for a thought-provoking article on data sharing and its numerous potential benefits to the research community, if we can collectively get the ecosystem right by ensuring common metadata standards, interoperability, and data search capabilities.
One self-interested reason why researchers might want to share their experimental data despite their misgivings on this score is that papers with associated data sets are consistently more highly cited than papers with no associated data sets, after correcting and normalizing for field and the year the papers are published. Increasing the citation impact of your own research is a universal goal–good open data practices aligning with the FAIR principles can be an important aspect of attaining that goal.
I always struggle with these types of studies, as they almost always fail to account for confounding factors. Perhaps people who publicly post their data are just doing better science than those who don’t? Perhaps those who can afford to pay for data curation and hosting are better funded than those who can’t, and that may lead to more citations? Without a randomized controlled trial, the most one can do is point to coincidence.
I also always worry about the ethical dimensions of telling researchers they can cheat the system and drive their careers through tricks, rather than focusing on doing really good science and the true value that open data (and open methods!) bring to the world. Even if you find these sales pitches as acceptable, their effectiveness decreases the more they are successful, as any advantage created will fade as more and more people take up the practice.
The point is well-taken regarding potentially confounding factors, this finding doesn’t account for those factors and is not a controlled study.
I personally don’t think it is unethical or a “trick” to say that sharing open data may yield higher readership and citation of one’s article, any more than a researcher trying to publish his or her work in a top journal to get more exposure to it is a trick. After all, open access advocates have consistently claimed similar benefits to publishing OA.
Appealing to the researcher’s self-interest may not be as high-minded as appealing to the abstract principle of the benefits open data can have to the field and dat sharing’s broader societal impacts, but given the resistance to sharing data that we still see among many researchers, it may be more effective. It’s also perfectly possible and reasonable to appeal to both.
Sorry, no, I can’t agree. Appealing to a researcher’s self-interest is one thing, selling open access or open data as a means to cheat the system is entirely something else. I wrote about this way back in 2011:
https://scholarlykitchen.sspnet.org/2011/04/05/gaming-the-system-is-citation-still-a-valid-metric-for-impact/
It’s particularly egregious when the appeal is based on financial ability — telling a researcher of privilege that they can essentially pay for an APC and buy prestige that their less well-funded colleagues cannot. By all means, appeal to self interest — open data makes your work more credible and shows the true productivity of your research by creating assets available for the community rather than hiding them in a filing cabinet. But selling these practices as sneaky tricks to make mediocre work look more impactful is dishonest (both because there has not been a causal relationship shown between the two, and that at best, it’s a short term phenomenon, as once more people take up the practice, the advantage disappears).
We take publishers to task when they pull tricks to improve the rankings of their journals — joining citation cartels, forcing authors to cite works from the same journal, publishing editorials that cite a long list of Impact Factor-counting papers. We should also hold authors and advocates for practices like open data to the same standard.