Scientific impact has become synonymous with the counting of citations.
That this measurement has hung on so long in an era of digital publishing and social network analysis is partly historical momentum, and partly the lack of research on comparing different measurement techniques.
A new manuscript in the arXiv, A principal component analysis of 39 scientific impact measures, attempts to compare many of these techniques. The authors, Johan Bollen, Herbert Van de Somple, Aric Hagberg and Ryan Chute, all work on the MESUR project at the Los Alamos National Laboratory.
Scientific impact is an abstract construct that may mean very different things. It can mean prestige but also popularity, and traditional forms of counting citations (like votes) simply equates the two.
Using citation data and usage data, the authors used principal component analysis to compare the relationships among 39 different types of impact measurements, such as journal impact factor, PageRank, H-index, and various measures developed for social networks. Their analysis involved nearly 7,000 journals.
Principal component analysis is a statistical tool for reducing the dimensionality of complex datasets to reveal a simpler (often hidden) structure underneath. These dimensions, called “components,” are not always easy to understand and often require some interpretation. The first two components that explain the most variation in the data are often plotted. The graph below summarizes their main results:
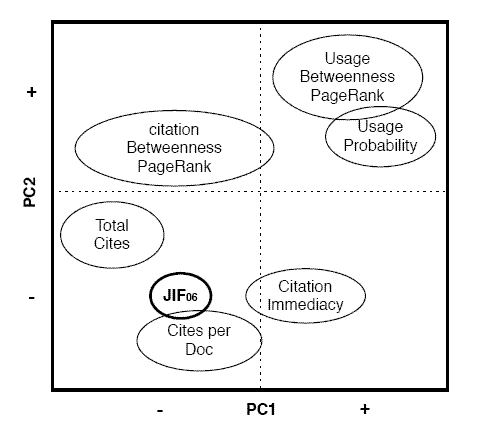
The first principal component (PC1) separated the citation measures from the usage measures (with the exception of citation immediacy), and could explain over 66% of the total variation.
The second component (PC2) may be interpreted as distinguishing popularity from prestige, and could explain 17% of the total variation.
JIF on this graph represents the journal impact factor, and its location in the plot did not go unnoticed by the researchers. The authors remarked:
These results should give pause to those who consider the JIF [journal impact factor] the “golden standard” of scientific impact. Our results indicate that the JIF and SJR [Scimago journal rank] express a rather particular aspect of scientific impact that may not be at the core of the notion of “scientific impact”. Usage-based metrics such as Usage Closeness centrality may in fact be better “consensus” measures.
What does “consensus” mean here? It simply means “consensus” among the different measurements. Consider that we have 39 blind men all touching an elephant, each reporting a different experience of what “elephant” means. Some of these blind men are in close agreement with each other, say a group of men touching the trunk and another group touching a leg. One single man may be touching the elephant’s tail. Picking the middle point — the belly — as a consensus among all of these points does not really represent a “consensus.” It represents a distinct body part.
While this manuscript represents phenomenal empirical work, “scientific impact” on philosophical grounds will always remain a complex construct; and because of its complexity, it will resist a single measure. We may all agree for practical purposes that it be redefined with a new counting tool. But that new tool is simply a different view of an enormous and complex beast.
Discussion
6 Thoughts on "Scientific Impact Measures Compared"
Hi Phil, great summary of our paper. I like your “blind men” analogy, but much depends on the size of the beast and the number of blind men. 200 blind men describing a dog, still a complicated beast, would do much better. Also, nothing is to stop each blind man from feeling various parts of the beast, or in fact the whole animal, and then comparing notes.
The latter may in fact be the correct analogy because each of our metrics is calculated on the entire citation/usage data set, the resulting rankings are compared over all +7000 journals, and our loadings indicate about 85% of all variation is covered by the first 2 components.
This paper is now officially published by PLoS ONE:
Bollen J, Van de Sompel H, Hagberg A, Chute R, 2009 A Principal Component Analysis of 39 Scientific Impact Measures. PLoS ONE 4(6): e6022. doi:10.1371/journal.pone.0006022
http://www.plosone.org/article/info%3Adoi%2F10.1371%2Fjournal.pone.0006022