Reproducibility of published research results is increasingly coming into question. Efforts from funding agencies and publishers are calling for more and more transparency around research data, but so far, little attention seems to have been paid to a crucial aspect of experimental reproducibility: publication of detailed methodologies. A new NIH-led effort seems a first step in correcting this oversight.
While access to research data is valuable for potential reuse and extension of research results, much of the emphasis stated for data policies has been to improve the reproducibility of the research behind it. As a recent PLOS blog post puts it:
Availability of the data underlying a published study is probably the most significant way in which journals can, now, ensure reproducibility of the published literature.
I’m not sure I agree. Being able to review the data does indeed allow one to see if a researcher’s analysis and conclusions drawn are accurate for that dataset. But it does little to validate the quality and accuracy of the dataset itself. I can look at the gene expression data derived from your cell lines and see if it really shows the activity of the gene you claim it shows, but I can’t tell if you really used the cell lines and conditions you claim you used.
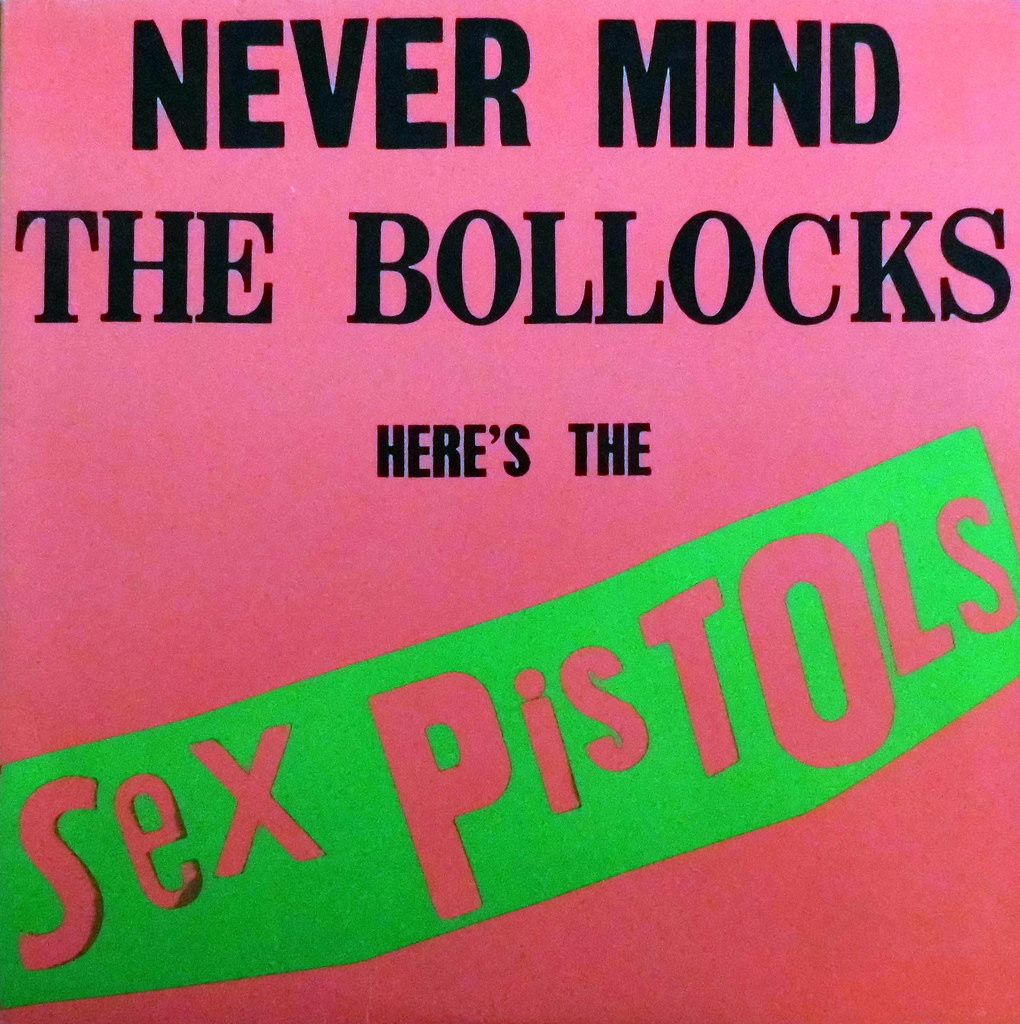
If I have serious doubts about your conclusions, then your data is less valuable to me than your protocols–the detailed methodologies you followed to derive that data. I need to see if your approaches were methodologically sound. If I hope to replicate your results, I need to know exactly what you did.
This is more complicated than you might think. The smallest variations in technique or reagents can lead to major differences in results. The scant information offered by most journals’ Materials and Methods sections makes replication fairly impossible. Often when describing a technique, an author will merely cite a previous paper where they used that technique…which also cites a previous paper, which also cites a previous paper and the wild goose chase is on. Methodologies evolve over time, and even if you can track down the original source of the technique, it likely has changed a great deal over the years.
In the past, access to data has been required by most journals, but only upon request. If funders and journals are now declaring that this is no longer acceptable, and that all data must be made public, then shouldn’t we hold researchers to the same principle when it comes to methodologies?
I will admit to some bias in this area. I spent years creating and acquiring laboratory manuals for a publisher, and then was one of the creators and the Editor in Chief of a biology methods journal. The mid-2000s saw a brief boom in the rise of methods journals, with CSH Protocols, Nature Protocols, Nature Methods and JOVE all appearing over a few years. Each journal seems to be alive and thriving to this day, and it’s perhaps surprising to me that the trend seems to have fizzled out. Sources of trusted protocols are more valuable than ever, particularly with the demise of the print laboratory manual.
I suspect that some of the reason is because getting researchers to write up their protocols is a bit like pulling teeth (at worst), or running a review journal (at best). Most researchers don’t think about writing up their methodologies. In my experience, this meant that many articles had to be commissioned. That’s a lot more work for an editorial office than sitting back and letting the submissions roll in. With little career reward offered for the development and sharing of methods, most researchers weren’t willing to invest the time and effort needed to put together a detailed and well-vetted protocol.
Cut to 10 years later, and we’re now in the midst of a data journal boom, with announcements of new entrants coming regularly. Researchers, however, may be faced with the same dilemma. While it’s repeatedly suggested that credit be given for creating datasets that are reused, until that becomes a solid reality, the career incentives for doing the work to create data publications may not yet be there.
In June of this year, the NIH, along with the Nature Publishing Group and Science, held a workshop on the subject of rigor and reproducibility, that resulted in a set of guidelines for reporting preclinical research. Many of the principles set forth are meant to improve both experimental design, and the reporting of that design. This is tremendously important, as Francis Collins and Lawrence Tabak note, poor training in experimental design is a key factor contributing to problems in reproducibility. The NIH has pledged to develop a training module with emphasis on experimental design (and for those who can’t wait, I highly recommend this book on the subject, with the disclosure that I was an editor on the first edition).
Other suggestions, such as setting high standards for statistical analysis and careful identification of reagents, cell lines, animal strains, antibodies, etc. will also go a long way toward replication.
These sorts of efforts are aimed at the pre-publication stage and if followed will result in a better quality literature, though one wonders how they will impact editorial costs and how additional procedures will fit with journals that have deliberately pared down the peer review process. Once the paper is out though, two suggestions are offered to help subsequent researchers replicate the results: data/material sharing, and expanding journals’ Materials and Methods sections to produce accurate reporting and key information.
The Nature family of journals, for example, will be expanding their Methods sections to allow for more detail. While this is undoubtedly an improvement, I’d like to see both funders and journals go even further. If we require release of data, then we must also require full, detailed reports of the methodologies used to derive those data. These could be published separately and cited, deposited like data in a repository and linked, or simply included in supplementary materials.
Preclinical research seems an obvious area to start these sorts of requirements. But why stop there? Detailed methodologies would be of tremendous value across the spectrum of scientific research. The validity of many types of sociological studies, for example, depends greatly on how those studies were conducted. Why not offer all the gory details to better help readers understand whether the experiments were well conducted so we know whether the data is worth reusing? Beyond reproducibility, increased availability of trusted protocols would be a boon to scientific progress simply because more people would have more access to more techniques.
Lest you doubt the power of the development of new techniques, go back and look at the last decade of Nobel Prizes in Chemistry and Physiology/Medicine: development of super-resolved fluorescence microscopy, the development of in vitro fertilization, discovery and development of green fluorescent protein, introducing gene specific modifications in mice, RNAi.
That’s a significant number of prizes awarded to methodologies. If the Nobel Committee can retroactively recognize the impact of sharing powerful methodologies, why don’t we offer that same recognition in real time? While it’s great to see the data behind an important experiment, if I’m going to take the next steps (or even check to see if that experiment was right), then I need to know how it was done.
Discussion
16 Thoughts on "Nevermind the Data, Where are the Protocols?"
This is a very good summary of the incompleteness of the recent reproducibility resolutions. Everyone who works in the lab knows that even perfect data is impossible to reproduce unless one (practically, not theoretically) knows the methods used to obtain the data. So the solution of the reproducibility problem is impossible without finding a better way to transfer the knowledge of methods between scientists.
The science publication culture focused on results, not methods, is the result of specific material circumstances: scientists are rewarded (get grants) if they discover something new. Therefore, for publishing scientists it is more important to describe the role of gene X in cancer than to describe a method on how to find such genes. There are rational reasons for such strong focus on results, but now this rationale makes disservice to science, especially to biomedical sciences.
At this time, the reproducibility debate is sliding on the path of least resistance toward data quality and availability. It is maybe because these issues look easy to fix by changing publication instructions and introducing IT solutions. I hope that once this stage is over and everyone realizes that the problem is not solved, the attention will turn toward the real problem, which is the methods knowledge transfer.
Back in the days of dinosaurs, at least in chemistry, researchers published protocols including instrumentation models, reagent sources, etc as part of the report. Thus it was easy for bench chemists to reproduce the results. It was not just the “data” but how it was obtained.
Today, especially in the bio sciences, the ability for one lab to reproduce what another lab has done may, as the article suggests, be next to impossible even if the second party had identical resources, the time and the fiscal capabilities to validate as well as carry out their own work. For physics experiments, not every one has a linear accelerator in their adjacent lab to validate work. So there is an element of “trust” that is needed and which appears, now to be in question, not necessarily due to attempts to mask the methods for whatever reason.
Recently, there have been articles on the pressure to publish and to do so often. That plays into the picture from both the publishers who have volumes and deadlines as well as for authors who need lines on resumes. This, too, mitigates against careful reviews (maybe even testing the data but getting no credit with blind reviews).
As this column suggests, its not just the data. It looks more like a death spiral to be slowed by creating more journals on data evaluation and related publications. There are now “slow food” movements and even a “slow money” movement. One wonders where the care and collegial nature of scientific research can find a path. One is reminded of the Peter, Paul and Mary folk song refrain, “when will they ever learn?”
With respect to the “the days of dinosaurs” you mentioned, it is possible that the reproducibility problem is worsened due to the growing complexity of the science practice. For example, since the end of 90s, the biological science was flooded by new technologies (e.g. microarrays, complicated gene-knockout methods, sophisticated microscopy). It would require a scientist’s lifetime to learn using even a big part of them. So from a science with a limited number of methods that most of practitioners knew how to use (e.g. DNA gel or Western blot), biology became a complex practice with large number of sophisticated tools used. It such situation, the lack of a proper infrastructure for dissemination of how-to became more detrimental than before.
Fundamentally, I think you’re right, David. It’s intuitive that the reproducibility problem cannot be solve entirely through data sharing. I say ‘entirely’ because data publication does have a role to play. Aside from the simple issue of data not supporting the conclusions in a paper through author error or deliberate over-interpretation, quite often, how the data is treated is just as much a part of the protocol as how fast the centrifuge was spun and which buffer was used. Particularly in fields like microscopy, which is playing an increasing strong role in biomedical research, the data can go through several iterations of processing and analysis.
Of course, this opens up the question of which version of the data is the one that should be published and I think that this objection gives a clue as to where we need to be heading as we try to adapt scholarly communication to make science more efficient.
I hesitate to use the term ‘open science’ because to many, that simply means open access but to me, there’s much more to it than that. This might sound like a bit of a pipe dream but I personally think that we should be headed to a situation where we think of a unit of research to begin when a researcher gets an idea and end when the impact of that idea has been maximized. Everything generated along the way might be considered an output from the technique to the digital lab notebooks to the results and interpretations.
Admittedly, this is all a bit blue sky and would require changes to the way in which we assess research impact and incentivize researchers. I would suggest though that the expanded interest in methods that we saw 5 years or so ago, and the focus on open data now are the first steps along the way to this Utopic vision.
I think you’re right in terms of how the ideal way things should be done, but there are always practical considerations that have to be balanced along the way.
First, there are an awful lot of papers being published every day, and most researchers complain about not being able to keep up, even with these simplified summaries of research projects. I’m not sure how realistic it is to expect hardly anyone to spend weeks digging through the enormous volume of notes, dead ends, data, etc., that accompanies a research project. Other than high profile papers, most of that material would never be looked at, or at best, maybe by one or two researchers. So that gets you into questions of time and cost, and balancing all the efforts needed versus the practical value. If I’m required to spend an extra 6 weeks putting together the documentation around every experiment, that’s 6 weeks where I’m not doing experiments.
There’s also the question of limited funds and limited job opportunities. We have set up a system based around competition–this is good in some ways as it is an excellent motivator, but bad in others as it favors those who are more secretive than others. If you give me your lab notes and data before you’ve figured them out, then maybe I figure them out first (see Rosalind Franklin’s x-ray image of DNA as the classic example).
Also, in thinking about a system of rewards and recognition, we want a system that rewards success, not effort. There are lots of bad ideas, and lots of experiments that go nowhere. You don’t want to promote and fund the researchers who don’t accomplish anything with their research. There has to be some threshold output where things start to matter, and perhaps the paper is not a bad model for it.
Until we have unlimited time and unlimited funds, we’re not going to reach the ideal you describe. The goal then, is to find the right focus and the right level of compromise. To bring out the most important aspects and go from there. And I think these efforts toward data and (hopefully) methodology transparency are good examples of practical implementations that make things better, but not perfect.
You make an excellent point, and you’re right, it’s an idealized model.
It’s important to make sure that the innovations that we as technologists, librarians, publishers and funders develop are not overly onerous and don’t detract from the real work that researchers are doing. That’s why it’s important, I think, to develop tools that seamless enable communication by integrating directly into a researchers workflow, especially if that tool actually adds value for that researcher at the point of use.
An example would be the ability to push digital lab notebooks to the web. Experienced researchers will tell you that taking a little time to keep proper notes as you go along will save you time in the long run, so a digital notebook that’s done right will save a researcher time and be invaluable to principle investigators, who often have to deal with loss of knowledge when students and postdocs move on. Systems like this will inevitably take time and a little bit of trial and error to get right.
The advantage however, wouldn’t come through lots of people being able to use each item, but from the fact that the objects were available in case they needed to be audited. Improving transparency in this way ought to make researchers more aware of the way in which they not only perform experiments but also handle their data. The point is well taken, however, that compliance with mandates to do such things would be poor without researcher buy-in.
I agree that there has to be a lot of thought put into the risks of data sharing for individual researchers. I was talking to an electrophysiologist about this just last week. He said that he’d want an embargo on original data because he often writes several articles based on the same data set. Since data sets take a couple of years for him to get, they’re highly valuable.
Taking good notes is indeed important, but is there really any advantage for the researcher in doing so publicly and online?
Perhaps the model to learn from is journals with open peer review systems. Researchers do seem to write better quality reviews when they know they’re going to be published, but at the same time, it takes them longer to write them, and they don’t speak anywhere nearly as freely as they do when anonymous and private. Keeping a public notebook probably does mean better quality notes, but they’ll come slower, and most researchers (as you note with the requested embargo) are going to hold back quite a bit.
Really it would need to be more of a cultural shift rather than something that can be legislated. “Make all your data publicly available” is not an enforceable policy, unless you’re constantly monitoring each and every researcher and know what experiments they’re performing and what data they’ve collected, so you can see if they’re really fully compliant.
The idea of an embargo is helpful, but again, how long should it be, and how would it be enforced? Maybe focusing on the paper is the best way to go–that’s a set event, when the paper comes out, the data (and methodologies) used to derive the conclusions in the paper should be released. This gives the researcher freedom to keep exploiting data that hasn’t yet been used, but creates that audit trail that you seek.
And the other practical considerations pile up as well–where do you keep it, who pays for that, how long must it be kept, what do you do about archaic filetypes and software, etc.
Please start a Journal of Properly Documented Research. Peer review of the detail and comprehensibility of methods would be great. I’m not sure that the Nature checklist or the PLOS data availability policy have really made much of an impact yet. It will require a gradual cultural transition, as you say.
I agree that focusing on the time of publication is probably a good starting point. One minimal standard could be requiring enough data and code to generate every graph and statistical result in the paper, sort of like Biostatistics papers with the reproducibility kitemark. Personally I would much rather review or read papers if I had that much information available. In many cases the code is easier to understand than terse prose descriptions in methods sections. Even better would be if the editor verified that the data and code supplied generated a rough version of all the graphs in the manuscript before sending it out for peer review—now that would be added value!
Another problem is endless chains of citations to previous work. More tolerance for verbatim copying (indicated with quotation marks and citation) could help.
There certainly are important trade-offs between research questions that can be addressed and the researcher effort required to make their work more reproducible (including both methodology and data). More time required to make work reproducible means less research questions, and less papers produced. However, we may be all better off when less research papers are produced but they are easier to follow, reproducible and of generally higher quality. It could lower retraction numbers, lessen researchers from following flawed research, and be better use of research dollars.
Of course, the incentives in research being as they are, a young researcher knows more papers = higher reputation now…
Back in the good old days, maybe 17th Century through early 20th century publishing was a collegial activity where my experiments, in detail were offered to the community to reproduce, use with credit and similar activities. Peer review was also a form of collegial exchange.
Today, what group of peer reviewers have the time, resources and inclination to engage in such an exchange to validate and advance the science while managing their own research and other obligations stretched thin?
As agreed, the data is only one piece (including how collected, assembled and massaged). The other piece is the validation by the larger community, particularly those committed to the responsibility of peer review, most of which is un or thinly funded by the journals. Publishers cannot stand outside and kibbitz.
In the natural sciences, sampling does not always take place under neat and clean laboratory conditions. Electroshocking fish, for example, requires a lot of adjustments to local conditions. Here’s where technologies like the wearable camera can be a big help in documenting exactly what was done. With new journal apps on the horizon capable of showing video, we may find our documentation methods changing significantly.
David, this is a great expansion on an idea that you have surfaced often in the comments – that having “the data” is not the key to being able to reproduce a result in all fields.
If there is a dataset that comprises the research materials, where the data are the actual “stuff” on which research is done – then having the dataset allows the analysis to be confirmed, re-performed, modified, etc. In those specialized areas of study (which include bibliometrics), the data themselves are required for reproducibility. (http://jcb.rupress.org/content/179/6/1091.full) But there are vast areas of research where “the data” are the result of an experiment on some other “stuff” – cells that are observed, gene activity that is induced, proteins that are labelled, patients that are treated. All you can do with these data are, really, look at them. You can re-run the maths if you suspect the wrong statistical technique was used, maybe analyze photographic data to determine it wasn’t altered. You can’t reproduce these data, nor build on them directly to extend the inquiry.
Maybe we need is more rigor in the semantics, since it seems like “reproducibility” has become a kind of catch-all term for too many different kinds of post-publication activity. Let me propose a rubric that parses reproducibility according to some particular goals:
– Validation: Did you do the experiment? This requires access to the original data to demonstrate that the experiments were performed and data collected and analyzed appropriately. This has long been the go-to for investigations of misconduct or misrepresentation. This is necessary occasionally and data should be made available on request.
– Replication: if I do the same experiment as you did, do I get the same results? For all experiments, this requires protocols to be sufficiently detailed. For experiments where data are the actual materials of the experiment, the data are necessary for this step, but the protocol or algorithm or maths are also necessary. Replication is often a first step before expansion (below).
– Expansion: if your conclusions are right, then this other thing is also true, and I want to test this other thing. For this, you require the protocol, NOT the data.
If you are consistently unable to expand the prior work by demonstrating its “next steps,” you often retrench to replicate the prior work. If that doesn’t work, the validity of the original might be questioned.
I do not object to data being openly available – I just do not see that as a magic fix for the inherent messiness of empirical research. And the cost in effort to make “the data” open is not always matched by the usefulness of having them.
For most primary research articles, I would like to read the exact description of the methods before I read the “significant results”; without the methods I cannot know whether the “significant results” are to be taken seriously or unsubstantiated hype. Yet, in most cases — including in redesigned articles formats to enhance transparency — the methods, even if described, are hidden deep into supporting informations. This act of relegating methods to materials that most readers never see speaks louder than the hundreds of editorials trying to bring attention to methods. The relegation of the materials and methods section is a very unfortunate and pernicious trend for scientific journals. It is an appropriate practice for news magazines but not for journals publishing original scientific research: http://majesticforest.wordpress.com/2014/09/13/reproducible-science/