Last month, the second NISO Plus Forum in Washington DC focused on the topic of Artificial Intelligence (AI), and Andrew Pace provided one of three provocations for the audience. His talk focused on “second questions”, when faced with the impacts of AI systems on scholarly communications. He laid out a variety of thoughts on what obvious first questions related to AI use, about how will we address the use of AI in article writing, what will be the roles of publishers and librarians in this new ecosystem, and how will we react when someone uses our content for AI training. He also asked the second questions like, how do we cite AI’s role in authorship, how can we ensure that AI systems are aligned with human values, and how can we use AI ethically. In thinking about the second order implications of AI’s use, I’m considering what are the implications if generative AI is successfully adopted and used more widely by researchers in scholarly publishing. Many have focused on the micro level of the ethics of the individuals, credit assignment, and the validity of ‘hallucinations’ of this model or that.
Paper creation had been growing at a somewhat steady pace for decades, but not because the average researcher has become more productive and producing more papers. A 2009 report from the STM association on the growth of publications concluded that the increased pace of content generation tracked closely to growth in the population of researchers. According to a recent preprint by Mark Hanson, et al, in recent years, however, the size of number of articles has been increasing significantly and this is putting a strain on the publishing community. This could be a result of either the COVID pandemic, the inclusion of a wave of Chinese, Indian, and other non-US/European authors, or some other driver (more reflections on the paper are forthcoming). Even before the widespread use of generative AI tools, the ecosystem was under growing strain.
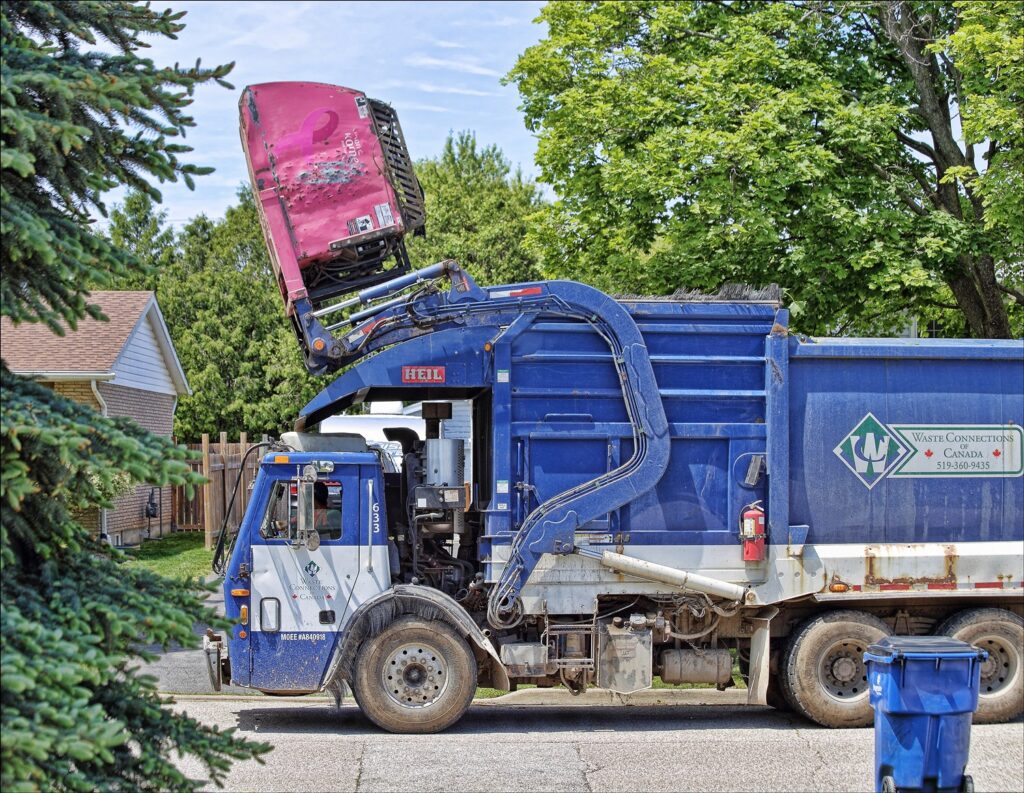
Many threads of discussion make it clear the advance in AI-driven generative content will impact our community, and likely not in the ways that most people are concerned with today. I am dubious that any significant number of researchers would fully trust a generative large language model to produce a text that they would submit for peer review under their own name. (Though one Spanish professor did apparently.) This is particularly true today, given the widespread awareness of generative AI systems to “hallucinate” – i.e., make up things up. One scenario I suspect is more likely will be that researchers will use AI tools to speed the writing process. One might think this is a path to greater efficiency and increased output. Possibly, yes, but my worry is that greater quantity will actually create bigger second-order problems.
Most are familiar with Stewart Brand’s 1984 quote about information wanting to be free. Some may even recognize his equally important prior sentence, “…information sort of wants to be expensive because it is so valuable — the right information in the right place just changes your life.” If information creation becomes ever easier, in the world of economics, as the supply increases the price will decline. This simple economic model presumes that there is a substitutional property of information that is the same or at least analogous to other products. If you are looking for a pair of sneakers, than a pair of white shoes is nearly equivalent to a blue pair and therefore the two are substitutable. You might not be pleased with blue pair, but they will do.
However, when it comes to information, particularly specialized, vetted, and novel information, there likely isn’t a substitute. The latest advances in large language model computer engineering or in mRNA research are likely not substitutable with just any old paper on neural networks or in biochemistry. Particularly, when researchers are interested in the best and newest papers in a field, those papers can be quite unique. This kind of information falls well into Brand’s second less-well know statement that information wants to be expensive. During the NISO Plus Forum, I had an opportunity to speak with someone from a high-value content provider, who noted that all AI-generated content was carefully vetted because, “people pay a lot for our service” and their reputation could be jeopardized by inaccurate information. The perceived economic value of accurate and timely information was paramount and the service that people are willing to pay a lot for.
A very much less-well known, but related concept from Esther Dyson came out a bit more than ten years later in an e-mail that she sent to David Weinberger on his influential listserv at the time. In that email she wrote these insightful sentences:
The new wave is not value-added; it’s garbage-subtracted. The job of the future is pr guy, not journalist. I’m too busy reading, so why should I pay for more things to read? Anything anyone didn’t pay to send to me… I’m not going to read.
Yes, in a world full of content and advertising and pr, I still want to know what your friends and mine are thinking, but I want only what they think is so good that they’ll pay to have me read it — because they honestly believe it will raise their stature in my eyes.
In Dyson’s vision, the problem develops as information explodes and overwhelms her. As a result, her view was that people will increasingly recognize the importance and value of selectivity. We will seek things that reduce the flow of information coming our way. In a world of ubiquitous information, curation becomes the most coveted service. Reduction, selection, and curation become the highest value an organization can provide. We need to subtract from the flow of information, by “deleting the garbage” in Dyson’s description.
Into this environment, generative AI systems will only exacerbate that problem. In the same way that robotics have made manufacturing processes more exact, more efficient, faster, and cheaper, AI tools will help everyone generate ever more content. As large language models and generative text creation AI systems make the authorship of content easier, ultimately this will only generate more and more content. At this point, I am not focused on the quality of machine-generated content. Let’s presume for a moment—a significant presumption to be sure—, that AI tools are used simply to speed the process of content generation and that the human researchers are reviewing, editing, and clarifying anything that the computer generates. The “garbage” in Dyson’s framing needn’t be the fake text that a generative AI hallucinated. It could be very respectable content that simply isn’t valued by the reader.
In this utopian vision, let’s presume that researchers are simply using these tools to be more productive by 20%, 30%, or even 50% more efficient in generating papers. If this is true what will be the implications? While automation tools are helping to speed the review and vetting of these papers in the editorial processes, there is also some concern that editorial vetting isn’t keeping pace with the increased workload. It isn’t yet clear that there is a marked decrease in quality. Regardless of the growth in dissemination, there isn’t a similar increase in the capacity of researchers to consume that additional content. Some tools exist to support highlighting the most relevant content, and these will very likely increase in value. The selectivity of the top journals in any domain will also likely increase.
The challenge with selectivity is that it is an expensive process. Determining what are the best papers to include in a journal issue requires dozens of editors or possibly hundreds of peer reviewers. If submission rates increase because more papers are being written with the support of generative AI systems, then the problems of editorial review will only multiply. Probably, these new papers will find some publication home in some journal or find their way into some pre-print repository. This increase in the average amount of content produced per researcher could increase the potential of having some great new discovery. Unfortunately, it will probably just be more content overall. The act of selection and curation will be increasingly more valuable, because the volume of content will overwhelm practically every field and every subdomain.
Reflecting on Dyson’s quote highlights a path forward for scholarly publishers and librarians alike. The notion that true innovation and value lies not in piling on more features or content, but rather in carefully curating and refining the offerings to deliver a more customized, streamlined, and user-centric experience. Ideally this will deliver, in Brand’s words “the right information in the right place” so that it can change lives.
Discussion
6 Thoughts on "AI Will Lead Us to Need More Garbage-subtraction."
Thank you, Todd, for the insightful post. While enabling an explosion of published content, AI-enabled discovery tools also provide a solution to finding and synthesizing information. Understand this does not address the added stress of more content in the publishing ecosystem. Still, it opens a question of whether or not publishers and other content owners will allow their content to be mined by AI discovery tools and applications.
For my part the anticipated increase in volume simply amplifies the value of human to human connections and networking. I will read what people I trust recommend. Better still when those people are part of shared communities of practice.
Great post, thank you! I share your concerns, although I think those of us working in research integrity would strongly disagree with this statement: “I am dubious that any significant number of researchers would fully trust a generative large language model to produce a text that they would submit for peer review under their own name.”!
While we can do our best to weed out AI generated submissions, ultimately I fear it will be our reviewing communities hit hardest. We may need to think more about reviewer recruitment, retention, training, and reward in this environment than ever before.
Perhaps this as good a time a time as any to reflect what science is and isn’t. Scholarly publishing is an important but only one part of science and probably the most abstracted part at that–a largely artificial and insular world of its own. I realize that this may not go down all that well in this venue, but as someone with a foot in both camps, I think it’s worth remembering.
On-the-ground science is a lot more, including physical work and interactions in labs, seminars and hallway chats, consultations and discussions with patients and other stakeholders, and myriad other things. With researchers and clinicians already being forced to drink from the firehose as readers and reviewers, AI may represent a tipping point forcing a reckoning with the outsized valuation of publishing for its own sake. If not, many, both within and outside science, will find themselves in a world of hurried abstraction that they no longer recognize and value as their own.
“Meaning emerges from engagement with the world, not from abstract contemplation of it.”
― Iain McGilchrist
This is an insightful and valuable post, I always appreciate a supply and demand analysis. A more succinct way of thinking about this is that with generative AI systems in use the supply of words in cogent order drops precipitously. Yet there remains a very limited finite resource of human attention. I second Edmund Lee’s and Kim Eggleton’s comments here. The role of humans in the editorial and peer review process is only going up in the short term. In the long term, perhaps someday a competent AI peer reviewer method will be developed.
Agree on all points. The second topic to ponder could be “but will both the Chefs and the dinner guests actually go for this?”. AI will make “McDonald’s free” and the “Michelin star restaurants more expensive”. How will people respond to that? Right now it looks like people choose fast, free, high quantity over quality…