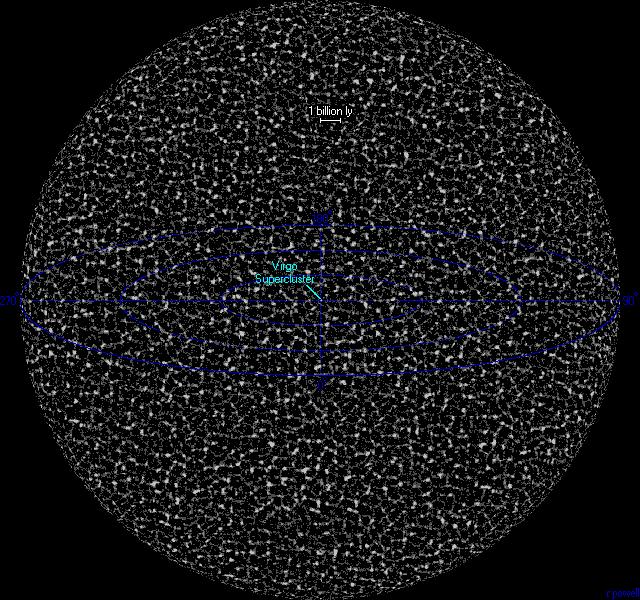
Scholarly information discovery is a broad topic, going well beyond search, and it varies tremendously across different user types. Some students are looking for three references to meet the requirements of a (poorly designed) undergraduate assignment. Most researchers are striving to maintain current awareness of the new scholarship in their fields. Whatever the research task at hand, scholarly publishers strive to support the discoverability of their works through all such workflows, while libraries strive to support the researcher’s discovery across all relevant works.
I have been tracking one kind of discovery – what I will call the quest for comprehensiveness – that is widespread among researchers but seems comparatively quiescent in professional discussion about supporting researcher needs. Researchers need to be confident that not only have they identified many sources on a given topic, but actually that they have located all of the sources that might be of conceivable relevance. This quest for comprehensiveness is expressed in a number of different types of projects that we do not always see as related to one another.
Scholarly papers and monographs typically provide at least some review of the related literature. These literature reviews are conducted in the course of research projects and grant applications. In addition to situating the present work in its intellectual context, they often have signaling purposes to potential reviewers, which is the topic for a discussion all its own.
There is also the standalone review article. In some fields, these are more typically written by a graduate student, often as a dissertation chapter, while in other fields these activities are taken on by more advanced researchers or with the extensive support of a librarian. Regardless of the exact contributors, preparing a review article is yeoman’s work for one’s field, providing bibliographic infrastructure to help others navigate a given topic. Review articles are so important that they are at the center of stand-alone periodicals in many fields.
The quest for comprehensiveness is not without its anxiety. For example, in a project about their research practices, historians told Ithaka S+R researchers again and again of a paradox. Given vast digitization and new search tools, it was becoming easier for them to discover items on any given topic. But the same digital abundance was making it harder for them to feel confident that they had found everything they would need to find in order to reach and defend their research conclusions.
In another project several years ago, I recall interviewing a political scientist, who shared his approach to conducting a literature review. He ran all his searches through the current version of the citation management tool EndNote, through which he could connect to many popular content platforms. By using EndNote as the front-end for search, he was able to track not only the searches he had conducted but also their complete result sets. Subsequent searches could be filtered against previous searches’ result sets, allowing him to quickly look at only the newly discovered items. When sufficient search iterations successively retrieved no new results (and all relevant references in relevant articles had been pursued), he was able to satisfy himself that his work was done. While this workaround helped him, it is obviously not the optimal way to provide for this type of much-needed functionality.
I share these examples not because they will seem surprising to those who are familiar with research habits. Rather, I offer them up to raise the question that for me connects them: Would it be possible, I wonder, to develop a discovery tool that is designed not to find the best items but rather to provide some assurance that you hadn’t missed something?
There could be a variety of approaches. The basic feature of the EndNote method described above is to allow result sets to be filtered against a list of all the items that a researcher has already reviewed and deemed to be relevant, and irrelevant, for the project. This could ideally work across platforms, so that an item that had previously been discovered via PubMed would be available to one’s filter when searching at Scopus.
I also wonder if bringing co-citation analyses into discovery environments would be helpful. In such a case, a researcher could somehow upload to a discovery tool a list of the references seen as most relevant on a topic. An automated analysis of what is most likely to be cited with those works would be returned – not unlike the way that a social network can suggest additional friends.
I offer these two approaches as more concrete examples of how discovery systems could be designed to help scholars in their quest for comprehensiveness, as they conduct literature reviews as well as other types of discovery processes. Are there opportunities for scholarly publishers, their platform providers, and perhaps other kinds of organizations as well, to support more effectively this type of research work?
See Part 2: Repackaging the Review Article
Discussion
11 Thoughts on "Thinking Through the Lit Review: Part 1: The Quest for Comprehensiveness"
Several years ago I developed just this sort of algorithm for the US Energy Department’s Office of Science. It finds all and only those articles that are closely related to a given problem or topic. It even ranks them by closeness. I have yet to find anyone interested in using it, so I am surprised to hear that there is a concern about comprehensiveness. Even for a narrow topic there are typically more papers than one can read.
I also wonder if bringing co-citation analyses into discovery environments would be helpful. In such a case, a researcher could somehow upload to a discovery tool a list of the references seen as most relevant on a topic. An automated analysis of what is most likely to be cited with those works would be returned – not unlike the way that a social network can suggest additional friends.
Correct me if I’m wrong, but ISI pioneered this approach 20 or so years ago by comparing the reference lists of multiple documents. Documents that shared a lot of references were likely related to each other and therefore of interest to the researcher. This approach can discover papers that are published around the same time as authors these papers would be unaware of each other. I don’t remember this feature (View Related Records) was used much by librarians or our users, however.
Phil, I know of several “similar” article algorithms, some of which may use citations as a signal. This one sounds pretty useful but I do not have working familiarity with it. If I understand correctly from your comment, it is not really about surfacing “everything,” although I imagine there could be done similarities in the algorithm.
“Everything” is a difficult concept, obviously. However, recommendation engines will consider references, keywords and co-authorship (and probably some other variables), when ranking similar documents. The population of potential matches can also determine what is recommended: the Web of Science is based on a much smaller and more selective collection than Scopus or GoogleScholar.
My algorithm uses term vector similarity, in effect making an entire document the analyzed object. This provides a great deal more useful information for measuring closeness than citations or keywords provide. Moreover the language used in the article is much more objective than the citations or keywords. The topic compels the language, while citations and keywords are chosen.
The concept of “everything” is indeed ill defined, because science is seamless, as it were. For every paper there are several closely related papers, going off in different directions. So one can move from one closely related paper to another, repeatedly, and get very far away from the first paper. From nuclear physics to forest management, for example. In this sense there is no such thing as everything on a given topic.
My algorithmic procedure solves this problem as follows. First specify a set of one or more papers as being central to the topic in question. Then I provide a measure of closeness to this set for all other papers. Once you specify a desired degree of closeness using this measure, everything within that degree becomes well defined, so you can in fact find everything. Solving the “everything” problem was fun.
When I was conducting user studies at Thomson Reuters, many researchers relied on Web of Science (ISI Citation Indexes) as a key resource for a comprehensive literature review for a grant application. Because they can’t miss something significant for the application, researchers commonly used WoS + ScienceDirect + Google Scholar + PubMed (where relevant) in tandem during a session. In EndNote, the ability to export or import citations from all major platforms supports this behavior of relying on multiple platforms.
The related records feature Phil mentioned uses co-citation analysis, but the seed of citations are the citations from an article/chapter/paper. The algorithm finds papers with shared citations and ranks them by the number of references in common. It would be interesting to see if it would be beneficial to apply this same algorithm to an EndNote library or customized list of references.
Thanks for the thought-provoking post.
Step 1: scholar uploads their current bibliography — all the articles she has used to date, hoping to get hear back what she’s missed.
Step 2: system performs two checks. First, as you suggest, it checks its corpus of citations for (missing) co-occurrences. Second, it does some textual analysis (using topic modeling or similar) on the articles to determine what the article is *about* and compares this to the full text within its corpus. This second step would pick up on items that might be highly relevant but not well-cited (for whatever reason).
Step 3: scholar sees a list of articles that she might have missed (given that we’re dealing here not with binaries but with probabilities, this list would probably be quite long but in descending likelihood of having been truly missed).
Of course you’d need a sufficient corpus to run this on. Google scholar could do this. Then within specific disciplines, maybe Scopus, maybe Sciencescape, possibly JSTOR (or JSTOR Labs ).
The challenge, as David points out above, is that true comprehensiveness is pretty nearly impossible because good grief, so to combat that you’d need ways to filter/prioritize that overall list based on other criteria (impact/currency/discipline, etc.) so that the researcher can determine how long she wants to chase that receding horizon…
Interestingly no one has mentioned the use of semantic search engines that can work across and into articles and selectively extract based on a variety of criteria including context. An interesting example is the presentation of Temis’ Daniel Mayer at the Mark Logic 2013 conference:
http://www.marklogic.com/resources/semantic-enrichment-for-the-information-centric-organization/
If one conducts a search for semantic search engines, I suspect that there are over 50 that range in complexity and sophistication. One of the keys here is access to the literature, including grey lit. Even in the STM area, these databases are often curated by publisher specific search strategies.
But the key here is that there are computer strategies that make human search almost quaint but relevant in the near term.
Actually I mentioned term vector similarity thus morning, which is a popular form of semantic analysis. So is keyword search for that matter. It is certainly true that my algorithm can only be executed by a computer, but human intelligence is an essential ingredient. If you know of a computer based comprehensive analysis system that does not rely on human input I would love to hear about it, but I doubt it exists. Most of the semantic engines that I know of are focused on question answering, not comprehensive analysis. As Roger points out, comprehension seems to be off the table.