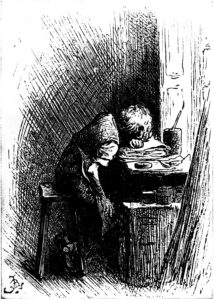
A few years ago, I was sat in my desk at a well known American university as snow fell silently outside my window. A knock on the door stirred me from my contemplation. A grad student that I occasionally worked with apologised for interrupting my thoughts and asked if he could borrow the analysis computer that sat in the corner, it’s over-worked twin CPUs gently heating my office.
“Why did you not tunnel in with SSH like I showed you and use it from your own desk?” I noticed the principal investigator of the lab appear behind the student and instantly regretted the inquiry.
“You mean like remote control? That’s cool, you’ll have to show me that sometime.” I shook my head in sad resignation and gestured for them to go ahead.
Young Tim’s (that wasn’t his name) first ever scholarly article was nearing completion, a publication that he desperately needed in order to complete his Ph.D. and start the postdoctoral fellowship that he had been conditionally offered. The gaining of a successful result was vital to the young man’s endeavours. At a prestigious university such as this, success was expected.
The professor stood over Tim’s shoulder asking pertinent questions about the various false colour images of slices of brain that were being called forth from the machine’s silicon workings. Tim dutifully explained the analysis that he had recently done and how he’d finally managed to tease out the result that he’d been looking for all these months. There were just one or two minor details that needed another set of eyes on before we could declare that we’d finally found that publishable result that would earn Tim that coveted Ph.D.
Within a few minutes, I began to become concerned. It became clear to me that entirely unintentionally, the experiment had been constructed and the analysis had been done in ways that all-but guaranteed the desired result. This wasn’t p-hacking or any simple statistical sin. What had happened here was experimenter effect. Due to the nature of the experiment, it was impossible to blind the samples because you could tell with even a cursory examination with the naked eye, which samples were which.
In the gentlest way that I could manage, I talked them both through what I saw and why I was sure the results weren’t valid. At first they didn’t believe me, so I took some of the data, changed the analysis parameters a little and changed the apparent result, proving that I could pretty much make it come out any way I wanted. There was no statistically valid way to know if the effect was real. At some point, the realisation became unavoidable. The professor walked to the opposite side of the room, leaned on the wall and put his head in his hands. The graduate student slumped in his chair, defeated, tears welled in his eyes. I knew I’d done the right thing, but it didn’t stop me feeling like I’d single-handedly destroyed this gifted young man’s immediate career plans.
What happened next I will remember for the rest of my life because it changed the way I think about how science is practiced. The professor rallied and proposed another analysis strategy; one that very well could be the correct way.
The grad student stopped looking at his shoes and desperately began to re-analyse the data. The result didn’t come out right so another idea was floated, then another. Eventually, a consistent criteria was found that would preserve the needed result. The images looked good, the graph came out right and it was a consistent analysis protocol that was applied to all samples. Even the statistics came out okay, so the problem was solved.
Only it wasn’t really solved. The way that we eventually settled on might have been the correct way to do the analysis, but we chose it because it gave the answer we wanted. You can’t reverse engineer an analysis protocol, even if you use the same protocol across all samples or data points, it still invalidates the statistics. I nearly spoke up but then I made eye contact with the professor.
Deep down, he knew, as I did that that we hadn’t proved that our result was robust, but there were other things to think about. This one experiment was part of bigger research project. We had other data that supported the overarching story of the science that we were trying to tell. The probability was that while this experiment may or may not have technically failed, it probably should have worked, so our analysis protocol was probably right. Right? To let this result enter the literature wouldn’t have a significant effect, it wouldn’t cause anybody to go down an experimental path that they weren’t going to anyway, and it didn’t rule out any life-saving approaches. There was no point being puritanical about it.
That particular experimental technique was notoriously confounded. It would be unfair to punish the grad student because the technique created results that were difficult to interpret; he was only doing the project he was given. If we were to say that we couldn’t publish the data because it wasn’t completely robust, who knows how long it would have taken that grad student to get this incredibly difficult experiment to work? A year? maybe two? Perhaps it never would and he’d have to start again with a new project. He’d certainly have lost that highly prestigious postdoc position and who knows if he’d have found another. To prevent that article from being published and seriously delay his graduation, or even prevent him from going into academic science altogether would have been a loss to the academy and unfair.
So, the question that I ask you, dear reader is: What would you have done?
Note: Almost all of the details in the the above story are made up. It wasn’t even snowing; it was June.
Discussion
31 Thoughts on "The Graduate Student’s Career – A Christmas Morality Tale"
I have never heard of a Ph.D. or a Postdoc depending on a publication. Publication is always uncertain and can take years. If true this is a fine example of funding induced bias.
At many institutions, particularly at more prestigious US universities, dissertation committees would generally expect something to at least be in press before letting a candidate defend. In recent years, the bar has gotten higher and the situation more challenging and competitive at all career stages.
In my graduate program you were expected to have at least 2 first author papers to get your degree.
Amazing. This means a lot of papers are authored by grad students. A thesis then seems redundant.
The thesis is then redundant, yes. In my field, the usual joke is that a thesis is three papers and a stapler. In practice, students generally write an introduction that serves as a general literature review for a wider audience, but this can be cranked out in a month or two by any qualified student.
In some programs, three papers are accepted in lieu of a traditional dissertation. I know for sure that the public health doctorate at Hopkins works this way.
I’m quite surprised that an accepted / in press publication is *required* (as opposed to desirable or optional) for the thesis to be submitted. I’d be very interested to see this written in the university’s rules regarding thesis formats. As I understand it, there is still considerable debate in some disciplines as to how acceptable these “alternate” thesis formats (as my university calls them) are for examination and award of a doctorate.
From a practical / administrative perspective, the unknown timeframe for scholarly publishing adds tremendous uncertainty into the term of candidature. How is this fair to the student, especially one paying tuition fees? Effectively the supervisors is saying that the candidature has a minimum term (according to university rules) but the maximum term is unknown (and indeed unknownable) and dependent of factors possibly well outside of the student or university’s control. What happens if a journal is very slow, or if multiple resubmissions are needed to get that publication? Is the student prevented – by the university – from submitting?
It wasn’t explicitly “required” but it was fully expected. If you didn’t, you needed to provide a compelling story why this wasn’t the case (and as noted elsewhere in these comments, there were cases where this happened). But the timeframe wasn’t really a consideration–it’s not like graduate students run on an annual school calendar. You graduated when you were ready to graduate, not in time to meet any particular deadline. Any deadlines were self-imposed (I want to start a new job, or I’m applying for fellowships that start on day X). There were several students who left and then came back to actually defend their thesis at a later date, and some who had a fairly understanding thesis committee willing to let them slide depending on the personal circumstances.
“But the timeframe wasn’t really a consideration–it’s not like graduate students run on an annual school calendar. ”
in Australia there is a generally a very strong focus on getting PhDs graduated in 3 years (or full time equivalent, FTE). My university – The University of Queensland – has “milestones” every 12 months (FTE): Confirmation of Candidature, Mid-candidature review, Thesis Review. Submission is then expected within 3 months (FTE) of the Thesis Review. These milestones are managed by the Graduate School. At each milestone, the candidate submits a document containing any writing done to date, an outline of the planned thesis chapter / sub chapter headings, timeline to finish, slides form any conference presentations, budget, and any issues / resources / problems etc. The first two milestones also involve an interview with an academic who is not part of the supervision team. Candidates who cannot pass the milestone must apply for an extension of 3 months (for academic reasons; personal reasons are handled differently). Only two extensions are allowed, any additional requests are managed through different processes.
I’ve not tried, but I doubt Dean would not approve any extensions because a candidate was waiting for a paper to be accepted! It would certainly be unusual at the Thesis Review stage. At that point the University is really keen to get the student – or at least the thesis – out the door.
Likely this varies quite a bit from country to country, and from institution to institution. Back in my day, most European postdocs got their degrees in 3 years, while in the US, one typically spend 4-6 years (and 4 was a rarity). Some of this may be due to the number of US students on training grants, which may offer a better economic value for a research program than having postdocs or technicians, who demand higher salaries, doing the research. The requirements for graduation, and the process, varied from department to department within a school.
A dissertation would allow the student to document the technical and interpretive problems of his experiment. As a journal paper, these important details are omitted. To me, the moral issue is not whether the results of his experiment were valid, but having his PhD advisors agreeing upon a form of public documentation that hides the technical and interpretive problems.
Interesting. I am hearing from our editors that more and more universities are going the route of having grad students publish journal articles that are then woven together to form the thesis. I have mixed feelings about this. I like that the grad students get training on how to publish a paper but they are under an intense deadline (graduation) to produce journal articles of any significance. It also feels like the universities are getting lazy when they do this. Instead of having to read the dissertation, they count on the journals to provide peer review and then just look at where the papers were published. I doubt they care very much about all the “missing” parts of a thesis when they are presented with 4-5 journal articles.
Phill: Imagine one cold morning you open an email from the Ghost of Christmas Future (aka the editor). She alerts you, the student, and his professor that a complaint has been filed with the journal. Evidently, a laboratory insider whistleblower (a fellow graduate student who had been worked to the bone in a cold laboratory with nary a wee lump of coal for the stove), contacted her with details that may undermine the validity of the research. Your institution’s IRB has been contacted as well and is beginning to assemble a committee to investigate the details. Lab books have been requested. Interviews with all involved are being set up…
As you wake from your fitful slumber, would you have made the same decision?
If this was a case of scientific fraud, you’d be right but the truth was that no data was faked or hidden, the analysis was done in a consistent way and the statistics showed significance. Everything was done to current standards in biomedical research.
What I perhaps didn’t make clear is that this incident was in no way unusual. If you listen to the way scientists talk about their work, or at least biologists, you’ll hear them talking about whether experiments ‘worked’ or not. What they actually mean is whether they got a positive result out of it. Having another go at an experiment, or reanalysing the data until the experiment works is how research is done. Science is difficult and messy, you know. Somebody doing science in accordance with common best practice is hardly grounds for an ethics investigation.
Anyway, it wasn’t actually my call.
Having sat on numerous thesis examination committees, all I would say is, tell the truth. One should not have to present positive results to get a thesis. One should show in a thesis that one has tackled a problem in a proper fashion, understands the subject, and is capable of cogently interpreting the results. The experiments may all turn out to give negative results, but if there is a coherent chain of reasoning, this should not matter.
Indeed, “negative” can usually be qualified. It means that the experiments had not worked out in the way one expected. But unexpected results are often very informative and may open up entirely new fields of enquiry. Unfortunately, a professoriat, conditioned by the demands of mission-orientated granting agencies, too often imposes a strict goal-orientated perspective on its students that militates against the recognition of novel opportunities.
One of the most valuable pieces of advice I received during my graduate career was from a well-seasoned professor who told me that a “thesis” can be defined as “whatever your thesis committee says a thesis is.”
As noted above, there was a general understanding in my program that you would have at least two first-author papers before you graduated, but this was not a hard and fast rule. I know of students who had been “scooped” while well into their research projects who were still graduated because they had displayed an understanding and an ability of how to conduct a high quality program of research.
It’s worth noting that it’s not just about getting the degree. For those that want to stay in academia, you also have to have land a postdoc position. Of course, it varies by institution, and usually, it’s the PI that makes the hiring decision, but a proven track record of being able to get published is getting increasingly important at earlier and earlier career stages.
I was speaking with somebody from the Psychology department in Edinburgh a few days ago about their experience as a supervisor. He told me that his first student created a very traditional style thesis that really hung together well as a narrative and beautiful to read. His second student focused on getting published and essentially stitched a couple of articles together in their thesis, resulting in something that was perhaps a little disjointed.
While the first thesis was ‘better’ in his opinion, the second student was the one that found a job.
On the top of the page, it says this post is filed under the headings “publish or perish” and “reproducibility” and I think it has some extremely interesting implications for each of these three topics.
Publish: The purpose, I would presume, of having the grad student publish is, in part, to ensure he is fully trained in this vital aspect of becoming a researcher. (Debate aside on whether all graduate training should have as its goal the ceaseless production of supernumerary scholars) Part of learning how to publish is learning how to conduct robust, correct, defensible research. In this moral tale, has that goal been served? As Phil Davis notes – permitting this publication without disclosure of the technical and interpretive problems is a disservice to the student and the field. And, yes, this is an awful scenario and one the adviser should have detected far earlier in the process.
Perish: How well-served is the field if someone surfaces the flaw, publicly notes that flaw and pushes this career-critical paper to retraction? Rather than delay the post-doc, this could end his career. Should it – is another question. What if the student himself eventually realizes this flaw and writes his own retraction? Will the adviser support that? Is the self-driven disclosure more valid and less damaging? Then why not do it up front?
Reproducibility: I am intrigued by the fact that Phill Jones uses the following mitigating argument: “This one experiment was part of bigger research project. We had other data that supported the overarching story of the science that we were trying to tell.” Is real “reproducibility” as necessary as the fact that the model/interpretation of the experiment creates further testable hypotheses that consistently support that model? Is it a valid mitigation that there are many other reasons to believe the conclusion of this flawed papers is correct? I worry that the drive to repeat individual experiments creates eddies in the flow of work – they are natural aspects of a river interacting with obstacles and irregularities in the banks – but too much of that recursive cycle prevents progress rather than supporting it.
Thanks for the puzzler, Phill. I love an unanswerable question!
Phill, I am not sure that I agree when you say this: “The way that we eventually settled on might have been the correct way to do the analysis, but we chose it because it gave the answer we wanted. You can’t reverse engineer an analysis protocol, even if you use the same protocol across all samples or data points, it still invalidates the statistics.”
Experimenters often develop experiments specifically because they know what they are looking for. The charge on the electron, for example. This case sounds like the same sort of thing (but we have no specifics). If the analysis is statistically valid then its origin (or intentionality) should not matter. What am I missing?
I happen at the moment to be developing semantic statistical tests to indicate bias in scientific publications. I would hate to think that the fact that I know what I am looking for somehow invalidates my methods.
Without going into too many details, the catch was that it was possible to change the result by varying the initial analysis parameters. I was able to make the observed effect appear and disappear. Essentially, the analysis wasn’t robust.
Thanks Phill, but I am still a bit confused, because you say this: “The way that we eventually settled on might have been the correct way to do the analysis, but we chose it because it gave the answer we wanted.”
If it was in fact the correct way then it was the correct way, regardless of the motivation. Math, if correct, is independent of motive. That is its beauty. If you found a proper mode of analysis that gave you the answer you hoped for then that was an achievement, not a dodge.
Regarding the parameters, I think it was Von Neumann who said that given four parameters he could make an elephant, and given five he could wiggle its trunk. The point is that the proper parameter values are empirical properties, hence part of science, not part of math.
Mind you I think that the nature of math, vis a vis science, is one of the greatest unanswered philosophical questions of our day, but that may not relate to your story.
The discussion of grad student publishing raises an interesting bibliometric issue, as follows. As I recall, it is estimated that something like two thirds of the people who publish a paper publish only one in their lifetime. Something like 80% of authors publish either one or two, then the numbers who publish more drop off rapidly. The point is that if a lot of grad students publish one or two papers then it may well be that the majority of papers in the literature, or in any case a large fraction, are written by grad students. Is this widely known?
The situation you describe ignores key players: the journal’s reviewers and editorial team. You and your partners are looking at some obvious problems. It seems rather cynical to assume the journal will just miss the problems and publish the paper. What happens if/when a reviewer finds the same obvious problems and asks embarrassing questions? My recommendation would be to present the work along with its limitations and to hope the journal finds the total effort worthy of publication.
Thanks Ken for your thoughts.
I’m not really ignoring the editorial team or the reviewers. The unfortunate truth is that the sort of situation I describe is extremely common and impossible to spot from reading the final paper. I feel like there is a lot of evidence to support the assertion that the literature has many irreproducible, non robust or slightly questionable results that don’t rise to the level of fraud or malpractice. Some of these results are generated through researcher error, and sometimes researchers know that they’re sailing close to the wind but feel like ‘they have to’ or that ‘everybody does it’.
Particularly when it comes to highly specialised techniques, the only way to really spot that the data is equivocal may be to re-analyse it from scratch and so to a great extent, we rely on researchers to self-police. As an aside, that’s essentially the reason that many open data proponents believe that making data available will help with reproducibility.
Part of the problem that I think we have with reproducibility is that for many researchers producing regular, positive results that can go into high impact journals is not the icing on the cake but a requirement for continued employment. This puts them in a position of occasionally having to choose the lesser of two evils, particularly when it’s somebody else’s job that’s at risk.
There is actually a lot of interesting research going on in this general area, Phill, which I refer to as “funding-induced bias.” For example, a very recent article on fudging the statistics in econometrics, to make them significant, is here: American Economic Journal: Applied Economics 2016, 8(1): 1–32
http://dx.doi.org/10.1257/app.20150044
Here is the Abstract:
“Using 50,000 tests published in the AER, JPE, and QJE, we identify
a residual in the distribution of tests that cannot be explained solely
by journals favoring rejection of the null hypothesis. We observe a
two-humped camel shape with missing p-values between 0.25 and
0.10 that can be retrieved just after the 0.05 threshold and represent
10– 20 percent of marginally rejected tests. Our interpretation is that
researchers inflate the value of just-rejected tests by choosing “significant”
specifications. We propose a method to measure this residual
and describe how it varies by article and author characteristics.”
More generally, I recently published a taxonomy of 15 different types of funding-induced bias, including a literature snapshot for each. See http://f1000research.com/articles/4-886/v1. Your case and the AEJ:AE cases sound like the type that I call “model bias.” In these cases they are statistical models.
You are quite right that this is subtle stuff.
Phil, I have to agree you’re right that most journals would not catch a subtle error in statistics or experimental design. Volunteer reviewers simply don’t have the time or inclination to do this unless something raises a red flag. I suppose an editor should be happy if the reviewers look closely enough to screen out fraud or deliberate bias!
With any luck, the real clunkers that hurt future research will become the subject of Discussion articles or later papers, to alert readers to their flaws. I guess the lesson is, one must continually follow the literature.