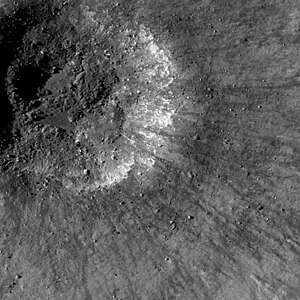
Three information scientists at the Université du Québec à Montréal are claiming that digital journal publication has resulted in a weakening relationship between the article and the journal in which it is published. They also claim that highly-cited articles are increasingly being found in non-highly-cited journals, resulting in a slow erosion of the predictive value of the journal impact factor.
Their article, “The weakening relationship between the Impact Factor and papers’ citations in the digital age,” by George Lozano and others, was published in the October issue of the Journal of the American Society of Information Science and Technology (JASIST). Their manuscript can be also be found in the arXiv.
The paper continues a controversy between those who believe that digital publishing is narrowing the attention of scientists and those who believe it is expanding it. There are theories to support each viewpoint.
As it requires access to a huge dataset of citation data in order to answer the hypothesis — data that greatly exceeds most of our abilities to acquire and process — we need to focus on evaluating the specific methods of each paper. Different kinds of analysis can return very different results, especially if the researchers violate the assumptions behind statistical tests. Large observational studies with improper controls may detect differences that are the result of some other underlying cause.
No study is perfect, but the authors need to be clear what they cannot conclude with certainty.
The Lozano paper is based on measuring the relationship between the citation performance of articles against the impact factor of their journal. The authors do this by calculating the coefficient of determination (R2), which is used to measure the goodness of fit between a regression line and the data it attempts to model. Lozano repeats this calculation for every year from 1900 to 2011, plotting the results and then attempting to fit a new regression line through these values.
There are a number of methodological problems with using this approach:
- R2 assumes that the data are independent observations, which they are not. If we plot the relationship between article citations for each article (X) and the impact factor of their journal (Y), then Y is not variable — it is exactly the same value for each article. Moreover, the calculation of the journal impact factor is based on the citation performances of each article, meaning that the two numbers are correlated. The result is that when calculating the R2, larger journals and journals with higher impact factors will have disproportionate influence on their results.
- Attempting to fit a grand regression line through the R2 values for each year also assumes that a journal’s impact factor is independent from year to year, which by definition, it is not. The impact factor for year Y is correlated by the impact factor at year Y+1 because half of the articles published in that journal are still being counted in the construction of the next year’s impact factor.
- The authors assume that the journal article dataset is consistent over time. Lozano uses data from the Web of Science which has been adding more journals over its lifetime. In 2011, for example, it greatly expanded its coverage of regional journals. Over a decade ago, it added a huge backfile of historical material–the Century of Science. Lozano does not control for the increase of journals in his dataset nor the growth of citations, meaning that their results could be an artifact of the dataset and not an underlying phenomenon.
- Last, the authors assume natural breakpoints in their dataset, which appear to be somewhat arbitrary. While the authors postulate a difference starting in 1990, they also create a breakpoint at 1960 but ignore other obvious breakpoints in their data (look at what happens beginning in 1980, for instance). If you eyeball their figures, you can draw many different lines through their data points, one for every hypothesis you wish to support. There have been many developments in digital publishing since the 1990s that don’t seem to enter the discussion. While the authors try to make a causal connection between the arXiv and physics citations, for example, there is no attempt to look for other explanations such as institutional and consortial licensing, journal bundling (aka, “The Big Deal”), not to mention the widespread adoption of email, listservs, or the graphical web browser. There is no discussion of other possible causes or explanations in their discussion.
The paper reads as if the conclusions have been written ahead of the analysis, conclusions which included the following:
Online, open-access journals, such as in the PLoS family of journals, and online databases, such as the ArXiv system and its cognates, will continue to gain prominence. Using these open-access repositories, experts can find publications in their respective fields and decide which ones are worth reading and citing, regardless of the journal. Should the relationship between IFs and papers’ citations continue to weaken, the IF will slowly lose its legitimacy as an indicator of the quality of journals, papers, and researchers.
This may explain the cheers heard around the altmetrics communities when this article was first published.
I’ve had a couple of great discussion with colleagues about this paper. We all agree that Lozano and his group are sitting on a very valuable dataset that is nearly impossible to construct without purchasing the data from Thomson Reuters. My requests to see their data (even a subset thereof) for validation purposes have gone unheeded. New papers with new analyses are forthcoming, I was told.
Discussions with colleagues surfaced two different ways to analyze their dataset. Tim Vines suggested using the coefficient of variation, which is a more direct way to measure the distribution of citations and controls for the performance of each journal. I suggest setting up their analysis as a repeated measures design, where the performance of each journal is observed every year over the course of the study. We all agreed that the authors are posing an interesting question and have the data to answer it. Unfortunately, the authors seemed too eager to make strong conclusions from inappropriate and rudimentary analyses, and the authors’ unwillingness to share their data for validation purposes does not give me confidence in their results.
Discussion
19 Thoughts on "Is the Relationship Between Journal Impact Factors and Article Citations Growing Weaker?"
R² doesn’t make any assumptions about independence: it’s simply a summary of the variation in the data. it doesn’t matter that the X is the same for one journals, R² is looking at the variation over the journals. I’m not sure if the correlation between IF and citations is because citations are included in both numerator & denominator – the way I understood it, citations in year t are ergresssed against IF calculated from citations in years t-1 and t-2.
I think the question of whether R² or CV is a good measure depends on the data – you’d have to look at the plots. I also agree the change-points are horrible: I think I’d fit a spline to the time series.
The half-life of citations might also be interesting to look at: I think a higher half-life will reduce the R², and this might account for some of the decline.
A lot of the analysis of this data would require playing around with it to see how what it looks like, so I agree that having the data available is important for evaulating the paper.
Bob, thanks for the correction. R2 alone does not assume independence, but using R2 to fit a linear regression line through the data most certainly does assume independence.
I’m not quite sure what the right analysis is for this problem, but I’m fairly sure that the R^2 is the wrong one. They basically assembled a gigantic list for each year that looked like e.g.
Journal Article IF citations
Nature #1 30 130
Nature #2 30 110
…..
Science #1 28 150
Science #2 28 70
….
and so on, for ~ 1 million articles
The signal we’re trying to detect is one of authors citing articles independent of the Impact Factor of the journal they’re in, and this should manifest as an increase in the spread of citation rates within journals.
Lazano et al’s analysis does sort of do this, but with two oddities. First, as Phil points out, setting the data up like this means that some journals have orders of magnitude more entries than others and thus more influence on the R^2. Since it’s the spread of citations within journals that we’re interested in, the journal and not the article is the natural unit of replication.
Next, the spread of citations within a journal would be given by the variance of citations, which is
SUM( [citations article i – mean no. citations]^2)
However, the equivalent for Lazano et al is calculated as:
SUM( [citations article i – Impact Factor]^2)
and, as everyone here knows, the IF is not the mean number of citations: it includes citations to editorial material that are not included in the article count, and there’s an unknown correction factor. Big differences between journals in the amount of citable editorial material or changes in either over time could account for some of the patterns that they observe.
I’d therefore be be interested to see these data reanalysed using the coefficient of variation of citations within a journal (i.e. st. dev. (citations) / mean (citations), as this removes the effect of journal size and IF. Plotting the average coefficient of variation through time for a particular field should show whether the spread of citation rates really is increasing. Testing this statistically would (as Phil says) involve a time series analysis.
R^2 doesn’t measure the strength of a relationship. It could arguably be considered to measure the strength of the relationship relative to the noise in the data, but this isn’t the same thing unless the noise and any biasing factors is constant (and the noise won’t be constant). The independence issue is therefore a bit of a red herring. Far more fundamental is the point that the R^2 could increase even in the strength of the impact-citation link decreased and vice versa.
Modeling the citation pattern over time is a better way to go (multilevel or time series analyses could accomplish this). Even a crude look at the unstandardized regression slope from year to year would be better than looking at R^2 (but not ideal).
Phil, what possible effect do you think ISI’s occasional revisions to inclusion criteria might have on these inflection points? The big journals can introduce sweeping innovations more easily, but also can get caught out if ISI changes inclusion criteria. Did the authors adjust for these well-known shifts within the citation data? Some of them (Lancet’s “Brief Reports” issues in the 1990s comes to mind, but there have been others) probably took a lot of citations out of play for IF calculations.
If you take a close look at their figures, you’ll notice that their annual coefficient of determination jumps quite a bit from year to year. This may be a factor of the kind of adjustments that are made to high profile journals like The Lancet that publish a lot of articles will have a disproportionate effect on the calculation of each year’s R2.
However, I imagine that major changes to Web of Science would have a much larger effect, for instance, when WoS starts indexing a few thousand more regional journals, since the citations won’t gets distributed evenly–a disproportionate number of them will be directed toward high-impact journals.
So, if your critique is valid, why was this paper published in the first place? Presumably this is a reputable journal. That it seems to have generated such interest just goes to show how little correlation there is between a paper’s quality and its impact!
I don’t know. They say that they’re working on follow up papers, but they should probably focus on writing a corrigendum/retraction for this one.
Without the data, I cannot say that their findings are right or wrong. The authors are making a pretty bold truth-statement without being able to defend it by sharing their data. This makes me suspect that their findings are not defendable.
The authors claim that their license with Thomson Reuters prevents them from sharing their data. If they could not agree to even basic allowances –such as sharing for the purposes of validation– the authors should have considered this before publishing a scientific paper on the topic.
You can’t have it both ways.
But we already knew that there was no relationship between the impact factor and citations. I wrote about it
http://garymcguire.wordpress.com/2012/07/09/citations-and-impact-factors/
and I gave some links to others who wrote about it before me.
I’m not a scientist and for the most part the math discussed here is above me, however I do enjoy reading the the scholarly kitchen. As I read this article it dawned on me that the data and the tools available to mine the data could provide an opportunity to determine an “ancestry of citations” if you will. One could map out which articles are siblings, parents, or cousins. I just thought that might be compelling to someone.
There’s quite a bit of data on this in Web of Science. Parent-child relationships are directly visualized as cited papers and citing papers associated with each record. Grandparent/grandchild papers can be gathered using the citation map. There are also “cousins” – or “related records” which mean that the two papers share one or more ancestors. Dr. Henry Small started this work many years ago at ISI as “co-citation analysis.” It can reveal some of the more subtle topical relationships among papers that might not be apparent using keywords or direct citation relationships.
Many, many studies have been written over the years using citation ancestry to trace the way a subject or field has developed across time.
Thank you Marie McVeigh, I was not aware but glad someone thought of it and it is being used in a productive way.
Just FYI, the three scholars who did this study aren’t all associated with Université du Québec à Montréal; at least one is with Université de Montréal.
Actually, all three are associated to the Université du Québec à Montréal; one is also associated to the Université de Montréal.
The decreasing relationship makes sense if you consider greater competition among journals. If editors did not actively solicit papers, then all papers would fall into a journal that matched their expected citations. But there is competition! I work hard to seek out top authors and convince them to publish in our journal. If I out-compete my fellow editors, our Impact Factor will hopefully rise, if I’m less successful, it will fall.