There are some things in this world that follow a normal (bell-shaped), distribution: the height of an adult moose, human blood pressure, and the number of Cheerios in a box of breakfast cereal. For these kinds of things, it makes perfect sense to talk about average height, average pressure, and average number of O’s per box.
However, there are many more things in this world that follow a highly skewed (or long-tail) distribution: family income, concert ticket sales, article citations. For these measurements, it makes a lot more sense to talk about medians — the midpoint in the distribution. If we treat these distributions like moose, we would end up with a very distorted view of the central tendency of the distribution. The Rolling Stones and Taylor Swift sell a lot of concert tickets, while most musicians barely eke out a living playing Tuesday evening open mic.
At a recent publishing meeting, two senior biomedical scientists both lashed out at the Thomson Reuters representative sitting in the audience about how the Impact Factor was calculated and why the company couldn’t simply report median article citations. The TR representative talked about how averages (taken to three decimals) prevent ties and how her company provides many other metrics beyond the Impact Factor. They audience just about exploded, as it often does, and turned the discussion session into a series of pronouncements, counterpoints, and opinions. I’ve attended this circus before: the strong man flexes his muscles, the human cannonball is shot above the crowd, the trapeze artists slips and the audience gasps. At conferences, the penultimate event before the ringmaster ends the show is the old professor standing up and lecturing the crowd about how, in his day, scientists used to read the paper!
The purpose of this post is not to further the antics and showmanship that accompany any discussion about metrics, but to describe why we are stuck with the Impact Factor — as it is currently calculated — for the foreseeable future.
In the following figure, I plot total citations for 228 eLife articles published in 2013. You’ll first note that the distribution of citations to eLife papers is highly skewed. While the mean (average) citation performance was 22.1, the median (the point at which half of eLife papers do better and half do worse) was just 15.5. The difference between mean and median is the result of some very highly-cited eLife papers–one having received 321 citations to date.
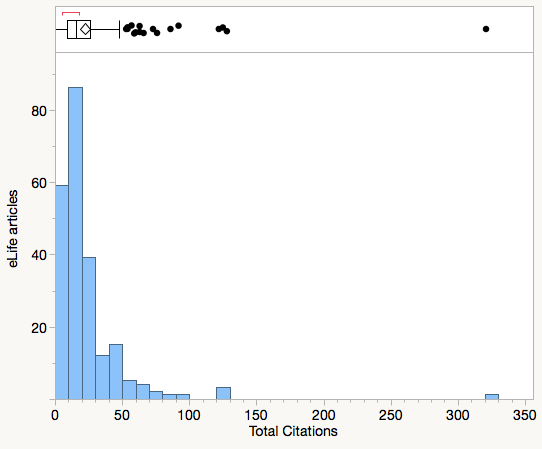
So, if I can calculate a median citation performance for eLife, why can’t Thomson Reuters? Here’s why:
Sometime in the middle-to-end of March, Thomson Reuters takes a snapshot of its Web of Science Core Collection. For each journal, they will count all of the of references made in a single year to papers published in the two previous years. This number, reported annually in their Journal Citation Report (JCR) is a different number than what you will get by summing all article citations from the Web of Science. Why?
The reason for this disparity is that the JCR is trying to account for citation errors — incidences of references that cite the old name of a journal or the wrong page number or misspell the author’s name or omit an important detail like the year of publication. Authors, as any copy editor can attest, can be very bad citers. The result of citation errors is that papers don’t always link up to their intended target reference and why the Web of Science often undercounts the true number of cited references.
As an illustration, last year, eLife received 2377 citations to 255 citable items, for an Impact Factor of 9.322. If you went into the Web of Science and counted all of the citations made in 2014 to eLife papers published in 2012 and 2013, you would get only 2213 citations, or 7% fewer citations than were reported in the JCR. Put another way, if you relied on reference-target matching from the Web of Science, you would miss 164 intentional citations to eLife papers. For many journals that I study, the differences between citations reported in the JCR and Web of Science are on the order of a few percent. For others, the difference can be huge. The Web of Science appears to do a much worse job on e-only journals that report just article numbers rather than those that provide redundant information like volume, issue, and page number.
The counting method used in the JCR is much less strenuous than the Web of Science, and relies just on the name of the journal (and its variants) and the year of citation. The JCR doesn’t attempt to match a specific source document with a specific target document, like in the Web of Science. It just adds up all of the times a journal receives citations in a given year.
So, what does this have anything to do with medians? In the process of counting the total number of citations to a journal, the JCR loses all of the information that would allow them to calculate a median. While you can calculate an average by just knowing two numbers — total citations on the top, total citable items on the bottom — calculating a median requires you to know the performance of each paper.
Over beer with Janne Seppänen, co-founder of the Peerage of Science and an avid hunter, I explained this in terms of moose. (Janne knows a lot about moose!) If Janne were interested in calculating the average weight of a herd of moose, all he would need to know was their combined weight and the number in the herd. In order to calculate the median weight of a herd of moose, Janne would need to know the weight of each moose.
Thomson Reuters could, in theory, calculate a median citation metric, but it would need to abandon their two-product solution (Web of Science as a search and discovery tool; JCR as a reporting tool) for just a single product, something I explored in 2014 post. With its Intellectual Property and Science Business up for sale, it is highly unlikely that Thomson Reuters will invest anything in these products, let alone consider combining them. In contrast, Scopus, a competing index and metrics tool owned by Elsevier, is based on a single database model.
Companies that are up for sale don’t invest in new products nor attempt to reinvent themselves but are singularly focused on cutting costs. It is just as unlikely that the new owners of the Web of Science and the JCR would invest anything (except rebranding) as their new parent company will ultimately look at how much profit they can squeeze out of their new purchase over the next five-to-ten years. For a company that truly wishes to invent a better product, it would be easier to develop one from scratch rather than invest in a legacy system that dates back to the 1970s.
Last year, Thomson Reuters unveiled two new metrics in its 2014 JCR: A Normalized Eigenfactor score and a Journal Impact Factor Percentile score. There are now 13 metrics provided in their panel of journal scores, but don’t expect median citation scores to come any time soon. Thomson Reuters simply cannot calculate them using their current production model. Should a competitor be able to provide such a median performance metric, I fully expect those advocating for medians to readily adopt it, even if it means that their journal will appear far less competitive.
Discussion
29 Thoughts on "On Moose and Medians (Or Why We Are Stuck With The Impact Factor)"
Thomson Reuters’ suggestion that taking averages to three decimal places is beneficial because it prevents ties is unhelpful. Presenting the average to three decimal places creates a false impression of accuracy – and what would be so bad about journals being tied? Many journals are in more than one category anyway, so already aren’t straightforwardly ranked.
We are only stuck with the impact factor because funders, institutions, authors and therefore publishers, are unwilling to embrace any of the other metrics on offer. Scopus matches citations to articles rather than just counting the total citations for a journal, so are arguably able to provide a better dataset and a more transparent metric, but there is a still a reliance on the impact factor.
It would be interesting to research into, or to read research on, how many citations are incorrect, and therefore how many citations the Web of Science actually misses. If the whole point is providing a metric of comparison between journals, and authors (more or less) cite incorrectly consistently across disciplines, then why not use the Web of Science data to create the JCR rather than a separate dataset? Thomson Reuters would then be able to provide a median rather than an average. It isn’t clear what advantages the JCR provides over the Web of Science. In theory, it is that the JCR provides a more accurate count of citations, but given that we can’t see what is citing/cited it is difficult to verify this. And if the main outcome is the creation of the impact factor, which masks the distribution and is incorrectly used given that citations aren’t normally distributed, then the heightened accuracy that the JCR is intended to provide seems an illusion.
Scopus, presumably, are in a position to provide a median, given that they have a single database model. It is just more evidence of the prevalence of the impact factor that their metrics have focused on imitating the impact factor rather than providing something new. It would be doubly interesting to see what would happen if Scopus were to launch a metric based on the median.
I suspect that one other change that a median based metric would create is grouping of journals with a median citation score of zero, one or two, particularly in the social sciences and humanities. Although I don’t have the data to hand to prove it. This could be a positive factor in removing the illusion of rankablity created by the current Impact Factors 3 decimal places. Another consideration of using medians, quartiles or other statistics, is how many people will understand them? Although given the lack of understanding around the current Impact Factor calculation I doubt this would make the situation any worse.
Good point!
For Biochemistry and Molecular Biology (n=290 journals), the median 2014 Impact Factor was 2.672, meaning that half of the 290 journals in this category scored lower and half higher. Given that the Impact Factor provides an inflated measure of central tendency for citation distributions, the majority of journals even in Biochem & Mol Bio would have median scores of 0, 1, 2 or 3. For History (n=87 journals), the median IF was 0.239 so I think we can be assured that the vast majority of History journals would be tied for a median score of zero. I suppose if anyone wanted to use medians, using a longer observation period, like 5 years, may be more appropriate.
It seems the IF and the median tell us about very different things. A median of zero, one or two tells us primarily that many articles were not cited. Is this useful? The IF gives an indication that some articles were probably highly cited (given the skewed distribution). The latter information is arguably more important because it indicates the extent to which the journal attracts important papers.
Thanks Phil for explaining the JCR vs WoS difference in terms a hunter-ecologist-entrepreneur can understand (though the practical hurdle of piling them on a scale is no less daunting than Thomson Reuters putting some developer time to make products sane).
That same floor-podium scene inspired me too to write a post on citation distributions, though from the point of view of the community most hurt my misused metrics – young researchers https://www.peerageofscience.org/a-tale-of-two-distributions/
I don’t think a median calculation would be terribly helpful. However, I would note that for journals that use Crossref the calculation of any of these numbers or extracting the full distribution is very easy – everything has a DOI, so there should be no problem with missing the citation links between articles. Unfortunately not everybody is on Crossref.
I think a more serious problem with Impact Factor is the temporal filtering. Journals that publish highly variable numbers of papers (for example ones that are just starting up or going through other major transitions) will have very skewed numbers because no account is taken of the distribution of published articles between those published early in the two-year time window and those later on. From the data I have seen, a far better measure is an average count of citations divided by the number of months since publication. For our journals (in physics) this rises from an initial value to about triple that value over the first three years, but then remains remarkably steady over about a 10-year window. I.e. citations to a given article tend to accumulate almost linearly for about 10 years, after an initial linear + quadratic piece. If most of a journal’s publications are at the end of the two-year IF time window, that will artificially deflate the impact factor; if the journal’s publication count has been declining by contrast that will inflate it.
I don’t know how universal the pattern we see is, but at the least taking an average of (citations divided by number of months since publication) gives a far more stable measure than just lumping total citations across a range of publication dates.
Not only does a publisher need to be a member of CrossRef, but they need to participate in their Cited-by Linking service (http://www.crossref.org/citedby/index.html):
Because [Cited-by-linking] is an optional service for our members and only a subset of the membership is currently participating, you will only be able to retrieve a partial list of the DOIs that actually cite your content. Crossref cited-by links are not intended for use as a citation metric.
Thanks for the clarification – the point remains that the errors with establishing per-article data which Thompson Reuters runs into as described in the above post are completely absent when you use the permanent identifiers the way across ref does. Why are more publishers not making use of this aspect of Crossref (or why isn’t Thompson Reuters using DOI’s??)
I computed standard errors for impact factors and median citations for all economics journals in the Web of Science:
https://ideas.repec.org/p/een/crwfrp/1302.html
Yes, breaking ties with decimal points makes little sense.
This is an extremely valuable insight into how TR collects their data! I wonder if the process would sound equally antiquated and arcane if TR would describe it themselves (not likely). You make it sound like the have hordes of helpers who sit in offices with a pen over reams of paper with type-writer written reference lists. It makes me think of Monty Python’s Crimson Permanent Assurance employees laboring away…
In a day and age when every blog (including this one) can automatically list pingbacks and all kinds of other citation-like actions, the scientific community relies on the phone-operators of the digital age to find out who should become a professor. That beats anything Monty Python ever came up with in terms of absurdity.
Scientists are all too keen to blame Thomson Reuters, publishers, or generally anybody else than themselves. The real problem is caused by all those professors sitting in grant committees, tenure boards, or doing research assessments (granted, some in the last category are pure administrators, not scientists).
Given that this issue has been known and talked about for years, it is hard to avoid the suspicion that people who have attained tenure in a flawed system are reluctant to challenge or change that system, in the fear that it would at least implicitly and privately question the idea that they have rightfully earned the right to sit where they sit now.
The interesting thing is Thompson will sell these divisions and the potential buyers do not care about the IP factor except it has to be part of the deal. The IP Factor is still the most trusted measurement tool for researchers, departmental review committees, and supports the peer review process. Don’t look for it to change any time soon.
Indeed, the section of a paper that commonly has the most edits when a page proof comes back from an author is the reference list. And even it always winds up in better condition once published than it was when first received in manuscript form, I know that these reference sections are still not perfect. From a practical production standpoint, in terms of cost vs. benefit, sometimes it is just not worth the investment of time and trouble to continually prompt authors to investigate every suspected error. Often, it seems, an author will simply pull a reference from another list that wasn’t carefully vetted and is all the author has for the reference — and thus perpetuates the error.
Thank you for this interesting post about median citation metrics. First, a bit of context about our (Scopus) current approach to providing journal metrics. We recommend Two Golden Rules for the appropriate use of research metrics: 1) Always use both qualitative and quantitative input into your decisions and 2) Always use more than one research metric as the quantitative input. A “basket of metrics” is the logical consequence of Golden Rule 2; there are different ways of being excellent and one metric will never give the complete view. You can see this in Scopus – besides regular document and citation counts, the SNIP and SJR journal metrics are included as part of the basket. These two journal citation-based metrics have been developed by bibliometric experts, and measure contextual citation impact by normalizing citations for the subject field (SNIP) and prestige based on the idea that ‘all citations are not created equal’ (SJR). As an immediate next step, in addition to these journal metrics, we are adding a simple citation-based metric looking at the average number of citations per document to our journal basket of metrics. The release of this new metric is planned within the next two months. Then, about the future. At the moment median citations is not a metric that we offer, and we do not currently have plans to add a median citation metric to our offering, but we are always open to suggestions from the research community if they give an extended, complementary view of journal performance. We would also consult our users to see whether adding a median citation metric would be useful to them, and to see how we should prioritize it. This is something we will consider. We have already assessed that median citations is technically something that can definitely be done in Scopus. I think you have made clear that the structure of the database is important to be able to calculate median citation metrics. In the Scopus database, citations are matched to articles that can then be rolled up to the journal (or any other) level. The number of citations for each individual article which is needed to calculate median citations is available in the database and therefore it would be possible to calculate median citation metrics for the journals in Scopus.
Wim, considering that you have the ability to calculate and report medians, it might be useful to investigate whether a median journal citation score would provide a useful addition to your “basket of metrics.” Would, as @JWRHARDCASTLE suggests above, most journals be assigned a median value of zero, one, two, or three? Would elite journals decline in rank when using a median metric versus an average metric? And, most importantly, would senior researchers–the ones who demanded such a reformulation of an average journal metric–adopt such a metric? While Scopus has worked very hard in developing indicators based on field normalization and network analysis, there is a real demand for a simple and intuitive indicator.
The coming weeks we are working hard to get this new, simple journal metric added to our basket of metrics. This will be a metric based on the average number of citations not medians. The fact that technically we would be able to calculate medians does not mean that we have already done it. But if we identify a strong need from our users, the analyses that you and James suggest to see what these median scores will look like and if there is possible adoption of the metric, would definitely be something we will have to do first.
There are two ways to create journal metrics: 1) Journal-level aggregation – like JCR. Including partial and/or unlinked citations if they are reliably identifiable to the journal; 2) Sum-of-Articles metrics where you are dependent on successful linking (InCites and SciVal – and a bevy of analytics providers).
Each, obviously, has advantages.
Each, obviously, has disadvantages.
The real challenge is to capture the advantages of both.
Waiting still to see that happen.
Median or mean is irrelevant for the actual problem at hand: The journal based metric doesn’t give any indication about the impact of an individual article, and, hence, any rating of the work on a scientist or a group of scientists based on journal level metrics does not truly assess the quality of their work. Yet another journal based metric will not change this. The only good that could come out of a median based journal metric is, that many journals would end up with the same rank, thereby the showing how ridiculous the system is.
A better analysis on why we are stuck with the “Impact factor” is given in this article:
http://mbio.asm.org/content/5/2/e00064-14.full
and they even propose how this could be changed.
Journal based metrics can tell us something useful about authors. Getting into a high ranked journal is like getting into a high ranked school. One has won a competition based on perceived quality.
No, you are wrong. Judging authors based on impact factor of journals is stupid. Beyond that, if your judgement carries any consequences, it is also highly irresponsible. https://www.peerageofscience.org/a-tale-of-two-distributions/
Sorry but other than name calling you have offered no argument. One of the functions of the journal system, perhap its highest function, is to rank articles based on the journals they can get into. This is the core function of peer review. The people who are using journal ranking to judge authors are neither stupid nor irresponsible.
The article you cite is just wrong. It claims that the following decision rule is needed: “Any article in a journal with a higher impact factor is more important than any article in a journal with a lower impact factor.”
Nothing of the sort is needed in order to make using the journal one publishes in a reasonable piece of evaluative information. In fact this proposition is preposterous, a strawman of the highest order.
I agree that that it is preposterous. And oh, I so wish it was a strawman. Sadly, it’s just preposterous. The name calling is aimed at my own community – the scientists – not SK authors, publishers, indexing services, or any other companies.
I have yet to find that any decision making body that is using such a categorical rule. Do you have any cases, or even any evidence that there are such? The rule actually being used is that an article published in a high ranking journal is likely to be more important than one published in a low ranking journal. Given the relatively high rejection rates common in the industry this informal, probabilistic rule makes sense. Nothing stupid about it.
What I have noticed is that anti-IF advocates tend to greatly exaggerate their case (just as OA advocates do). This is a common trait of advocacy. I doubt that IF ever controls decision making, although it may occasionally beused to break a tie. No one is making promotion or funding decisions based solely on the IF of publications. We are talking about very smart people here, most of whom understand statistical reasoning. The IF is just a reasonable piece of evidence of researcher quality.
Fortunately, even the editorial leadership of high-impact journals themselves disagree with your point of view David. IF abuse is on its way out. http://www.pnas.org/content/112/26/7875.full.pdf
This article is a case in point for what I am saying. It claims with no evidence whatsoever that people are relying “exclusively” on the IF in making judgements. I doubt this ever happens. Repeating claims which provide no evidence is not evidence. To paraphrase Wittgenstein, it is like buying two copies of the same newspaper to verify its truth. Repetition of unfounded claims is not evidence for them.
It is especially interesting that the people making these claims are far removed from the people they are making the claims about. Editors do not grant tenure or funding. Let us hear from the people who do, that they rely exclusively on IFs. I cannot imagine such a case, but I am always prepared to be surprised, because I am a scientist, doing the science of science.
One simple thing that all citation index services should include, is ranking of articles based on citations per time unit. Article from 2000 that has been cited 100 times is probably less interesting for me than another article from 2014 that has been cited 20 times.
When I want to quickly get an overview of a topic I know little about, this is what I do: 1) identify the seminal articles on the topic (keyword search, look for old articles with hundreds of citations) => 2) fetch articles that cite the seminal articles => 3) rank the list based on citations per year. In my experience, if you do the above and start reading articles from the top of resulting list, you are pretty quickly on the map regarding current relevant science on the issue you’re interested in.
It’s a simple enough procedure to do manually, but it feels like it should be simple for service providers to automate much of that task for me.
Interesting algorithm. Have to think about it. Re #3 do you mean the article with the highest average citations per year ranks first or that with the highest one-year citations (the hottest year, as it were)?
I mean the highest average per year, not hottest year. High one-year rates could be interesting too, but maybe targets different thing than what I want to find. I could imagine that research that is initially very exciting but is then proved wrong would generate such a “mayfly” signal. Like many Nature and Science articles.