How well does TrendMD work to drive eyeballs and citations to your journal? It depends on how you analyze the data.
A recent paper authored by the founders and employees of TrendMD, a recommender system for academic content, reported an overall citation benefit of 50% — a net gain of 5.1 citations within 12 months. I took issue with how the company reported its findings in a way that is consistent with good marketing, just not good science.
After several conversations with the first author, Paul Kudlow, I was able to secure a subset of their dataset for validation purposes. This was the best I was able to achieve as the journal in which their paper was published does not have an explicit data sharing or archiving policy.
The subset represents eight journals publishing in the Health and Medical Sciences. This is an important detail, as it is here where the authors reported the largest citation effect from their study: A mean increase of 20.45 citations per paper, which translates to an 82% benefit. Even a novice to citation analysis will realize that this is a very large effect.
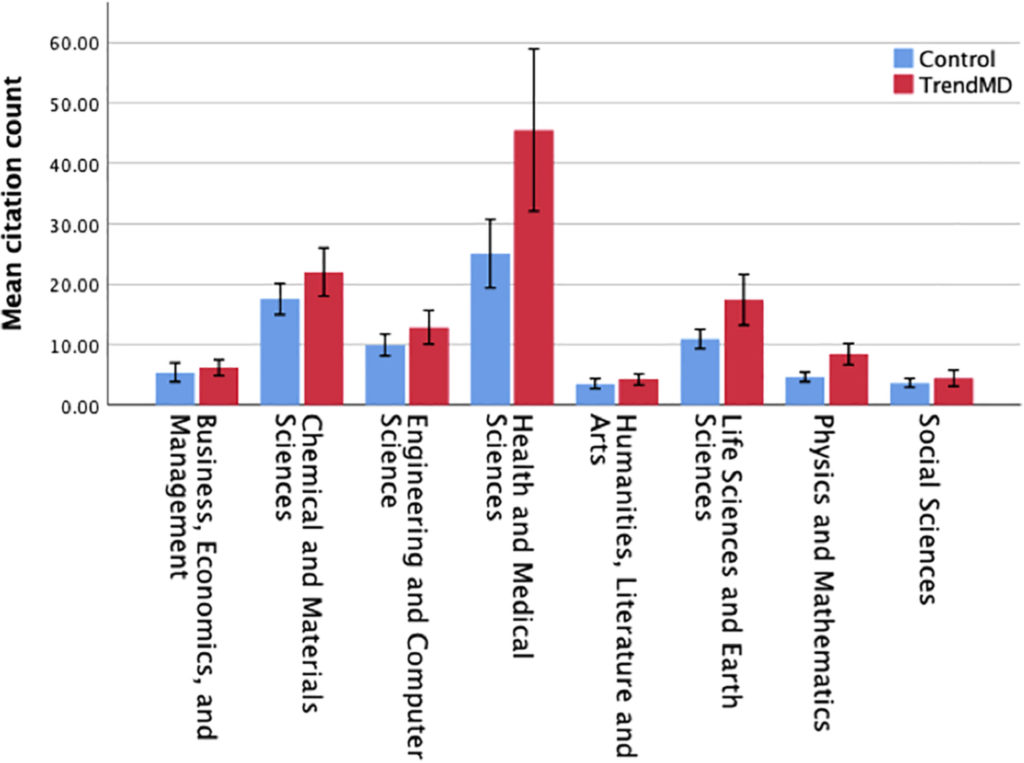
The effect of a single outlier
As it happens, there was one extreme outlier in the TrendMD arm that exerted a huge influence on their overall findings. The paper, published in Circulation, received 1,013 citations over the 12-month observation period (Fig. 1 below). While the dataset did not include article titles, it is pretty clear what this paper was: Heart Disease and Stroke Statistics Statistical Update 2018, an annual report issued by the American Heart Association, which typically receives thousands of citations over its lifetime. Remove this one paper and the red bar in the above figure drops by 5 citation points or 20%.
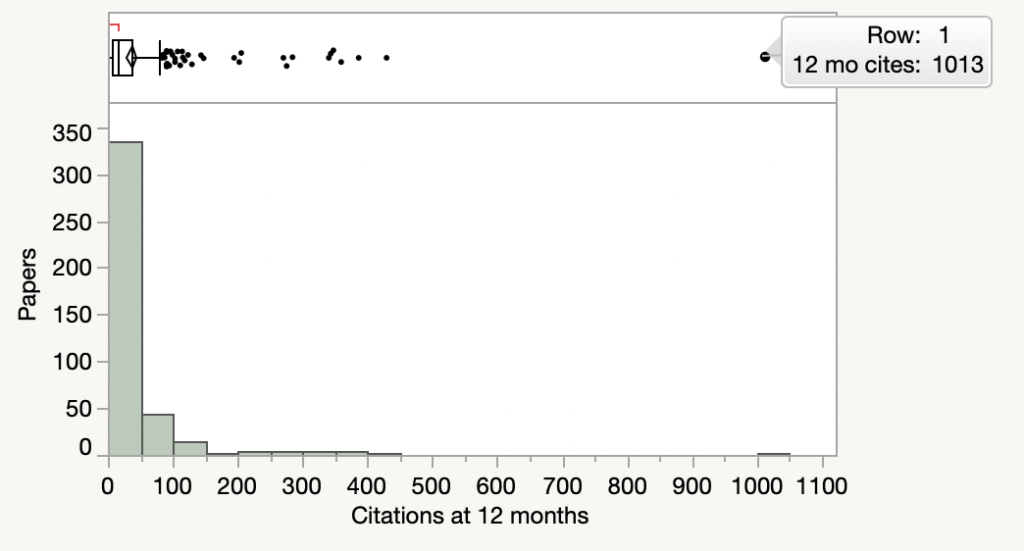
What is the real TrendMD effect?
First, I need to clarify that by using the word “real” I am not accusing the researchers of data fabrication. By “real” I mean reporting a statistic that is both appropriate to their data and meaningful to current and prospective clients of TrendMD services.
Based on a simple linear regression that normalizes the distribution of citations (i.e. converts it from a highly skewed distribution to a bell-shaped distribution and therefore minimizes the influence of outliers), we arrive at an overall citation benefit of 17% (Table 1), still positive and significant, but a far cry from the reported effect of 82%. Seven of the eight journals demonstrated a positive effect, while one estimate was negative. Don’t read too much into the exact performance of each journal as we expect high variation given the limited size of each journal subset.
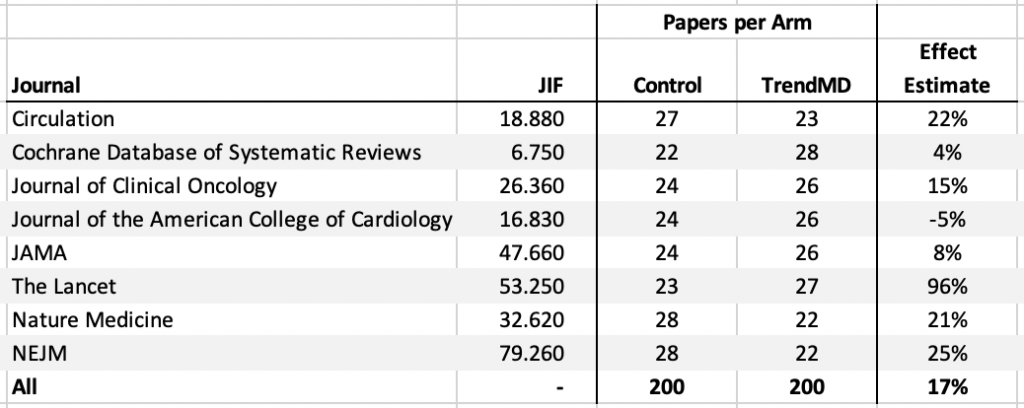
Rich get richer
In spite of the high variation in results, there are some general trends in the data that may help us understand how TrendMD works and whether preferential bias is built into their algorithm.
Papers published in higher impact (JIF) journals appear to receive a stronger citation effect. Based on a more elaborate regression model, the TrendMD effect increases by 0.5% for each 1 point increase in JIF. Given that there are many cumulative advantage (Matthew) effects known to operate in science, how does this affect TrendMD’s recommendation system?
Opening TrendMD’s black box
Even after controlling for the number of click-throughs (readers clicking on a recommended article link from the TrendMD widget), the number of impressions (the number of times an article is recommended to a reader) remains a significant predictor of citations. How can this be?
From their paper, Kudlow and others describe that the impression predictor could be explained through indirect effects: Just by seeing a recommended paper, a user may be more likely to read it, save it, and cite it at some unspecified later date. To me, this seems highly speculative when there is a more direct explanation.
Preferential bias built into the algorithm
While we don’t know exactly how TrendMD’s recommender system works — it is essentially a black box — we know from the company that it includes three components: 1) keyword overlap between papers, 2) the reader’s clickstream history, and 3) the clickstream history of other readers.
However, neither #1 (keyword matching) nor #2 (reader’s clickstream) can explain the residual effects of link impressions or why higher impact journals received a stronger TrendMD effect. But #3 (community clickstream) can.
For analogy, consider a restaurant recommendation app on your phone that operates similarly to TrendMD. You search for “Thai food” and the recommendation algorithm finds kitchens in your vicinity that include words from the restaurant name (e.g. Taste of Thai, Bangkok Express) and menu choices (Pad Thai, Tom Yum, Pla Goong). The recommendation also knows that you search for Thai food regularly (who wouldn’t?). However, the recommendation system also weights your results based on what other users have searched and rated. When it comes to restaurants, users really want to know where other people dine and enjoy, which is why this component is featured so prominently on apps like Yelp. Recommendation drives traffic and traffic influences recommendation.
So, if TrendMD is recommending papers based on community clicks, it is recommending papers published in more popular, frequently-read, wide-distribution journals. Put another way, TrendMD is biased toward recommending papers from prestigious journals. To explain their results, Kudlow and others don’t need to speculate on what readers are doing when not using TrendMD; they simply need to look more closely at how their algorithm is working.
Final Thoughts
After reanalyzing a subset of TrendMD’s dataset, I am still confident in the efficacy of this product: It appears to work, just more moderately than reported by the company. Nevertheless, most media companies would be thrilled with an effectiveness of 17%, as studies of the effect of social media on readership and citations report few (if any) effects.
Placed in context, TrendMD may have developed a highly effective tool for driving traffic to relevant content. What makes it different from other products with recommendation systems (PubMed, Scopus, Web of Science, ProQuest, EBSCO, among others) is that TrendMD operates from within the content itself, obviating the need for keeping readers engaged in a large siloed platform. This platform-neutral model allows TrendMD to grow organically and, in theory, benefit from scaled network effects. TrendMD also allows customers to actively promote their content in the recommendations, similar to paid search ads showing up within Google’s organic results or sponsored product recommendations on Amazon. Publishers should take interest, only cautiously and skeptically.
This is my own recommendation.
Discussion
17 Thoughts on "Data Analysis: How Effective Is TrendMD?"
If there truly is a “TrendMD effect”, then anyone counting citations as a comparative measure of research quality/impact now needs to adjust for it. Otherwise a significant part of the citation count is just measuring whether the journal happens to have implemented Trend MD.
Citation counting in all its forms is increasingly outdated, gameable, and counterproductive. Research funders need journals to do better a better job at evaluating research outputs.
Richard Wynne
Rescognito
Richard,
I think you’re missing the bigger picture here. If promotion of articles in TrendMD confers a citation benefit, then isn’t the product also helping readers discover more articles?
To your second point, I think most would agree that evaluation using citations alone is a flawed metric. Instead of saying someone else should think about how to evaluate research – can you offer some alternative suggestions?
Regarding your point that citations are ‘gameable’ by promotion:
Promotion of scholarly articles, whether through TrendMD or other channels, simply improves the likelihood that relevant information is visible to readers; promotion in and of itself does not cause individuals to use and/or cite the work. I believe that stakeholders in the scholarly communication ecosystem have a responsibility to ensure that content is maximally visible to those who could potentially make use of it.
Paul
Thank you for responding. Some inline responses below. I’m happy to continue the conversation through direct message on LinkedIn.
“I think you’re missing the bigger picture here. If promotion of articles in TrendMD confers a citation benefit, then isn’t the product also helping readers discover more articles?”
Richard>> TendMD is obviously a beneficial tool in the context of helping readers discover articles. But the “job” of a scholarly journal goes significantly beyond connecting readers and authors (Short blog posting on this topic here: https://www.copyright.com/blog/guest-post-unease-scholarly-publishing/ ).
To your second point, I think most would agree that evaluation using citations alone is a flawed metric. Instead of saying someone else should think about how to evaluate research – can you offer some alternative suggestions?
Richard>> Many people are working to improve research evaluation, for example the Declaration on Research Assessment (DORA) and Center for Open Science (COS). My contribution is Rescognito – a platform that seeks to recognize factual contributions to the research workflow in a granular, transparent and attributable manner: https://rescognito.com/about.php. (Thank you for the plug opportunity!).
Regarding your point that citations are ‘gameable’ by promotion:
Promotion of scholarly articles, whether through TrendMD or other channels, simply improves the likelihood that relevant information is visible to readers; promotion in and of itself does not cause individuals to use and/or cite the work. I believe that stakeholders in the scholarly communication ecosystem have a responsibility to ensure that content is maximally visible to those who could potentially make use of it.
Richard>> There are many ways to “game” citations beyond promotion. For example, publication of Review Articles of the type mentioned in Phil’s posting are frequently used by journal editors to improve their Impact Factors. (Review-type articles by their nature garner higher citations). Of course, any system can be gamed. The main antidote is improved transparency, granularity and verifiable attribution so that gaming behavior is either discourage or can be easily filtered out.
Phil,
Thanks for the interesting read this morning.
The observation about the Matthew effect is spot on. The question is whether the “TrendMD” effect is additive or synergistic for the author(s), the journal, or both. It will be interesting to see what happens as the sample size grows.
George Garrity
NamesforLife and
Michigan State University
Thanks for writing this follow-up piece, Phil!
Paul, a TrendMD client (who wishes to remain anonymous) asks me whether there was any effect for journals that had the TrendMD widget installed on their journal and whether the effect differed for those who paid for promotion and those who didn’t.
Phil — the current study looked at the effects of paid promotion in the TrendMD Network. We didn’t examine the effects of the widget itself or non-sponsored promotion of articles in the widget. I would imagine using the TrendMD widget would only amplify the positive results we found. We do have some evidence for amplification effect of the TrendMD widget. See https://onlinelibrary.wiley.com/doi/full/10.1002/leap.1014
Excluding journals that already had the TrendMD widget installed would have allowed you to isolate the effects of sponsored promotion from the widget itself and the effects of non-sponsored promotion. To me, this muddies the RCT trial and makes a simple comparison more difficult to make. Even if this were an oversight in the experimental design, it could (should) have been in the analysis.
Phil – I don’t agree that the TrendMD widget muddles the results of this RCT. Without analyzing this further, because this is an RCT, the use of the TrendMD widget should be similar across the control and intervention groups — so how would this confound the results?
In terms of the medical subject area, at the time of the study, only 3 of 8 of the publications had TrendMD installed; these were JAMA, Journal of Clinical Oncology, and the Journal of the American College of Cardiology. Articles from these journals in both the control and intervention arms would have the TrendMD widget implemented — so there should be no confounding here.
What am I missing?
Yes, it was an RTC, but the randomization was done at the subject level and not the journal level. I point this out in Table 1 of my post. Furthermore, its clear that the TrendMD effect does not appear to act equally across the dataset. Adding an indicator variable (has widget=1; no widget=0) to the analysis would have answered this question and not put you in a defensive position. Remember, I have no conflicts of interest in answering this question and would be willing to re-analyze a full dataset (all journals) if you included the widget indicator.
Phil – I’m happy to have you re-analyze the dataset I provided to you. I gave you the journals with the widget at the time of the study. Let us know what you find!
Phil – see comment above. I provided you with the 3 journals in the Health and Medical Sciences that *I think* had the widget enabled during the time of the study. So you now have the widget indicator for this subset; with this, you should be able to answer your question at least from within the medical subset.
As I just mentioned to you over email, we unfortunately did not record which journals in the cohort were using the widget versus those that were not using the widget during the time of the study. So these data do not exist in our dataset, nor could they be ascertained with any degree of certainty.
Again, I am genuinely curious. Please let me know what you find given the data you currently have. If you find something within the medical subset, I am happy to go further digging with you.
Unfortunately, 3 journals equals a total of 150 papers (78 intervention vs. 72 control, unbalanced). This sub-subset is statistically unpowered to detect anything but a very large main effect and almost zero chance of finding an interaction with the widget. I don’t need to do an analysis to tell you this.
I looked through the dataset again just now. Although I am not 100% certain because it was never recorded in our dataset, it doesn’t look like any of the journals in the other 2 categories (physics and life sci) where we found a positive citation effect at 12-months were using the widget at the time of the study.
Dear Paul,
many thanks for publishing this study and the former one on Mendeley reads. I appreciate this level of transparency by a private company.
Is there any change you would be willing to share the data? I’d guess more people would be interessted in (re-)analysing it to verify your findings.
Thanks,
Stephan
Hi Stephan –
The primary reason why we haven’t released the data publicly yet is because we are planning on a follow-up study comparing the citations accrued in this cohort of articles at 2 and 3 years. Once this is complete, the entire dataset will be made public.
That said, so long as your use case is academic and not commercial, I would be happy to give you, or anyone access to the 12-month citation dataset (without article DOIs for the reason I mention above). If you would like access, feel free to DM me on Twitter @PaulKudlow (I prefer not to give out email publicly on this thread).