At the annual meeting of the Society for Scholarly Publishing held in Portland, Oregon last month, the closing plenary session was a formal debate on the proposition “Resolved: Artificial intelligence will fatally undermine the integrity of scholarly publishing.” Arguing in favor of the proposition was Tim Vines, founder of DataSeer and a Scholarly Kitchen Chef. Arguing against was Jessica Miles, Vice President for Strategy and Investments at Holtzbrinck Publishing Group.
I organized and moderated the debate, and am very pleased to be able to share here the prepared texts of both debaters’ opening statements and responses. One of the highlights of the program was the vigorous discussion between debaters and audience, which took place following the formal statements – and our hope is that the discussion might continue here in the comments.
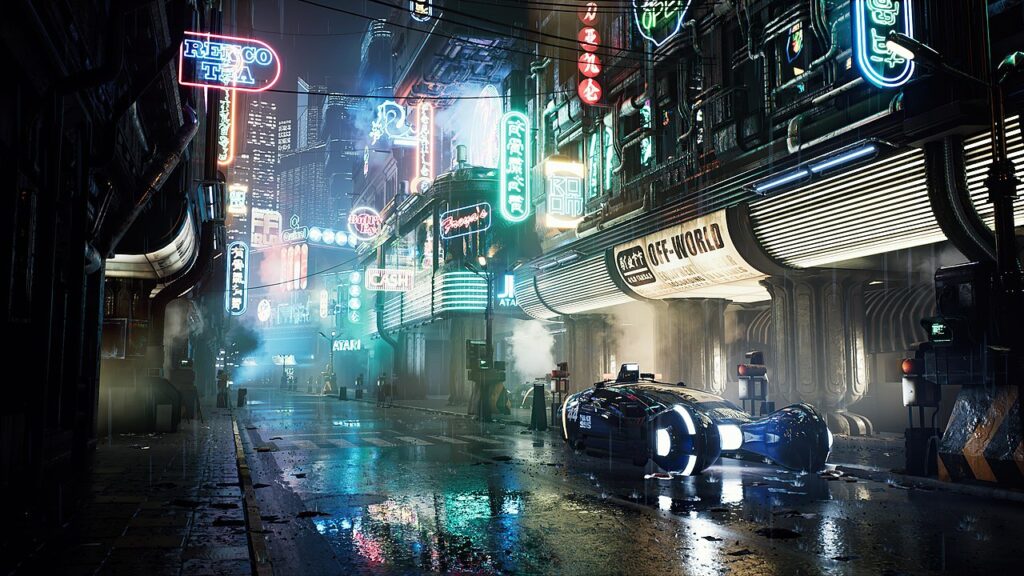
Tim Vines – Opening Statement
You’re in a desert when you see a tortoise. You reach down and flip the tortoise onto its back. The tortoise lays on its back, beating its legs trying to turn itself over. But it can’t. Not without your help. But you’re not helping. Why is that?
The cinema enthusiasts among you might recognize this quote as part of the Voight-Kampff test in the original Blade Runner. The test is used to pick out artificial humans (aka ‘replicants’) by probing for unexpected emotional responses. As the movie progresses, it becomes clear that even this test struggles to identify some advanced replicants.
I’m going to argue here that AI will fatally undermine the integrity of Scholarly Publishing, and a great many other things besides. There are three reasons why scholarly publishing is particularly vulnerable.
First, as with Blade Runner’s replicants, it will soon become almost impossible to distinguish the products of Artificial Intelligence from products made by humans. Unscrupulous researchers will be able to conjure up convincing research articles without the trouble of picking up a pipette.
I sense that some of you have a spark of hope that new tools or better screening can detect these faked articles. Let me snuff out that spark right now. Humans are relatively good at spotting AI generated pictures of people and things because our ancestors spent millions of years ‘learning’ to spot uncanny faces or strange shadows, but we have no such evolutionary history with scientific text or datasets. We must therefore rely on technology.
An image has a multitude of elements that must all be exactly right for it to pass as real. Text is simpler by several orders of magnitude, and hence there is far less that AI can get wrong. Even if we do find some bug that’s a giveaway that an article was made by AI, that bug will be fixed in the next version. The arms race of using technology to spot fake research texts is a race we have already lost.
Second, the task of spotting AI generated articles will fall to the Editorial Office. Yes, the perennially under-resourced, under-trained, and under-staffed Editorial Office. If you have faith that the Editorial Office has the tools and ability to weed out artificial articles, you may want to reflect that ORCID is now ten years old and most journals still don’t require that all the authors have one. If we as an industry are so delinquent in implementing basic author identifiers, what chance do we have to consistently detect research faked by sophisticated AIs?
Another route to detecting articles generated by Artificial Intelligence is to require that the authors provide the datasets and code objects that underpin the conclusions presented in their article. Faking these outputs individually imposes a significant extra burden on authors, but having them all be sufficiently interoperable to generate the results in the article is very challenging indeed.
If – and only if – scholarly journals insist that authors provide their datasets and code during the peer review process and then test the reproducibility of the authors’ analyses can we expect to weed out faked research.
Will this approach work long term? Given the breakneck pace of AI development, it seems certain that the capacity to fake datasets and functional code to go along with a fake article is not far off. Open science buys us time to develop new approaches, but by itself it will not save us from the corrosive effects of AI generated fake research.
Moreover, even if many publishers adopt and enforce rigorous open science policies, scholarly publishing has a dirty secret: a substantial fraction of the industry prefers not to ask awkward questions of their authors. These publishers are instead happy to receive the author fee in return for publishing the article and have no incentive to weed out plausible fake articles. Why would they? The fakes are unlikely to be spotted by readers, and every author is a paying customer.
Even if some part of the scholarly publishing industry makes best efforts to spot fake papers, the proportion of the literature that is entirely AI generated will grow relentlessly.
And that brings us to the third reason why artificial intelligence will – in time – fatally undermine the integrity of scholarly publishing: once the scholarly record is contaminated with many thousands of plausible but fake articles, how can researchers build on previous work? How can we train useful AI research assistants to draw new insights from the scholarly record, when that record is deeply contaminated with nonsense?
This dilemma has echoes of a story by Jorge Luis Borges about the fictional Library of Babel, a near infinite library containing all the knowledge in the universe. Despite this wealth of knowledge the library is useless: it also contains every other possible book containing every other arrangement of letters, such that the useful information can never be found.
As the trickle of AI generated fake research grows into a flood, we must ask ourselves this: is scholarly publishing willing to do whatever it takes to act as a source of truth? To fight a constant battle to ensure that at least some published research is created by real humans in a real lab?
Given our abysmal progress with implementing measures like ORCID and Open Science, the answer is clearly ‘no’. We will be fatally undermined and we will fall.
Jessica Miles – Opening Statement
I’m so appreciative of the invitation to participate in this debate. I would also like to thank Darryll Colthrust, Arjan Grootenboer, Leslie McIntosh, Henning Schoenenberger, and Reshma Shaikh for being so generous with their time and insights as I prepared my remarks for today. And, of course, I thank all of you for being here – for carrying on through the end of the meeting, through these whirlwind few days devoted to “Transformation, Trust, and Transparency.”
Although this theme seems designed to examine the rise of AI in publishing, it was, improbably, announced in October 2022, predating the release of ChatGPT and the ensuing generative AI frenzy. Prescient as this choice seems, I think what the timing really reflects is the reality that scholarly publishing must constantly manage technological revolution. As Todd Carpenter reminded us several weeks ago in a post in The Scholarly Kitchen, “The publishing process has always relied on technology, from the paper or ink with which scribes noted their work (yes, pen, paper and ink are all technologies), to the earliest typesetters and printers, to the digital markup and repository tools of today.” Trust and transparency have been critical for weathering centuries of transformation: in response to upheaval, we have come together as a community to create transparent and reliable systems and processes, informed by shared commitment to safeguarding the scholarly record. We will continue to do so in this new age of AI.
Over the last few decades, the publishing community has prioritized trust and transparency in the face of radical change. In response to the advent of Internet, World Wide Web, and other technologies like HTML, SGML, and XML, scholarly communities established clear, industry-wide infrastructure, protocols, and standards, like Digital Object Identifiers and Crossref to establish trust by sustaining a reliable, persistent system for linking scholarly references across the publishing ecosystem.
According to STM, publishers have collectively invested over £2 billion since the year 2000 to digitize the scholarly record, make it more findable and accessible, and safeguard its integrity, by developing tools to identify plagiarism and other forms of fraud. As research has become increasingly more collaborative and its outputs more diverse, we developed and implemented the Contributor Roles Taxonomy – more widely known as CRediT – bringing more transparency to the myriad roles researchers have and increasing trust in authorship attributions. More recently, STM formed a working group in 2019 to explore the implications of AI technologies for scholarly communications. The group has since released a standard-bearing white paper on “AI Ethics in Scholarly Communication”. These examples of how we’ve sustained trust and transparency by supporting industry-wide standards and developing technology and infrastructure in response to transformation provide a blueprint for how academic publishing will continue to evolve and endure in response to AI.
Yet, even in spite of these inspiring activities, we must acknowledge scholarly communications, does face critical, potentially existential challenges – ensuring research integrity, evolving business models, and sustaining peer review among them. However, it is people, not technology like AI, that fuel these threats. And people can, working collaboratively, develop and implement strategies for overcoming these crises.
Digital transformation, far from undermining publishing, has made the publishing ecosystem resilient in the face of continued change. Writing for The Scholarly Kitchen earlier this year, Hong Zhou and Sylvia Izzo Hunter detail how automation, big data, and cloud computing have expedited and improved submission and peer review for authors, reviewers, and editors. Publishers have invested in technological infrastructure to develop and enhance platforms and services for submission, peer review, and production. Automation has been instrumental in accelerating production processes, including automated recognition and disambiguation of authors and institutions, as well as automated typesetting.
At this point, some of you may be saying – wait, aren’t some of these so-called “automation” developments you’re referring to actually based on AI or machine learning technologies?
Yes. Yes, actually, AI is ubiquitous in scholarly publishing. I’ll repeat this: AI is ubiquitous in scholarly publishing. Recently, discussions of AI – like this one – have focused on Generative AI, a type of artificial intelligence technology that can produce various types of content. Large language models (LLMs) are one type of generative AI since they generate novel combinations of text in the form of natural-sounding language. While this emerging technology has the potential to profoundly change scholarly communications, we should not lose sight of the earlier, “classic” forms of AI and machine learning technologies that are 1) prevalent across publishing workflows and 2) importantly, have not undermined the integrity of scholarly publishing, despite their prevalence. The “AI Ethics in Scholarly Communication” white paper highlights a few examples from prominent commercial and society publishers:
SpringerNature uses these technologies to identify facts, concepts, and relationships in scientific manuscripts and transform these data into structured databases for downstream applications, such as inferring additional facts or identifying patterns. Elsevier maintains a data integration platform that, following FAIR Principles, helps users access clean, reusable data and metadata to optimize decision making, improve data governance, and refine subsequent AI/ML-based approaches to discovery. ACS integrates AI-powered transfer tools, which leverage semantic analysis and publishing history, with their peer review system to drive insight for authors, editors, and peer reviewers.
As these examples illustrate, AI is making publications more accessible and usable, demonstrating how publishers’ continued commitment to safeguarding the scholarly record informs responsible engagement with technological innovation, past and present. And we should learn from the past as we continue to confront persistent risks. There is still a meaningful risk that publishers will create siloes by relying on undisclosed technologies and internal standards of ethics and governance, rather than industry-wide protocols and guidance. There is still a meaningful risk that small publishers will be excluded from this wave of change because of the price of developing and deploying digital resources. [Post-debate note: Due to time constraints, I didn’t discuss this point as thoroughly as I would have liked – those interested in additional perspectives should view the “Current applications of AI in production workflows” panel from ConTech 2021.] It will be difficult for us to ensure accessibility, and it will undoubtedly require a cross-publisher approach. But we have shown, as a community, that we are capable of innovation and collaboration and that we can leverage our existing infrastructure to mitigate the risks associated with AI and realize its potential.
How? In the conversations I had with colleagues working at the forefront of integrating these technologies in scholarly publishing, several key themes emerged:
First, we must preserve trust by putting humans at the center of everything we do, with the mission of accelerating research, eradicating bias, and fighting fraud to ensure quality and integrity. Both classic and gen AI approaches can give us additional tools to improve and scale these activities. For example, AI tools for text summarization and translation can help authors who find it challenging to meet the requirements associated with publishing in English, broadening the accessibility of scholarly communications.
Second, we must ensure transparency in the production and use of AI. Specifically, this includes an understanding of what data are used to develop these systems, how they are being deployed, and how they make decisions. At this point, you may be thinking of recent remarks from Google’s CEO, Sundar Pichai, when he said that there were aspects of AI systems that experts and developers don’t fully understand. More recently, a research team at Stanford refuted these claims in a preprint posted on arXiv. Their work provides evidence that so called emergent abilities are not a fundamental property of AI models, but arise from the researcher’s chosen metrics for analysis. Related to this finding is the idea of explainable artificial intelligence, which seeks to develop tools that allows human users to understand and trust the outputs created by machine learning algorithms. These are valuable methods for fostering transparency and human-centeredness as we develop increasingly sophisticated technologies.
Last, we must improve the data that AI technologies are using. At a forum on Responsible AI in Berlin last month, the old adage “garbage in, garbage out” was a major point of discussion, with the panel — which featured data scientists, politicians, and scholarly publishers — agreeing that many problems we have encountered with AI are related to the data these systems are trained on. An approach that keeps humans at the center of technology development means that humans, not algorithms, innovate to source these data and collaborate to improve systems for evaluating data quality. One such approach could see publishers collaborating to build a cross-publisher corpus for training a large language model exclusively for scholarly communications, to ensure high quality input and training data for this shared resource.
In many ways, the path ahead is a promising one. If history is any indication, scholarly publishing will, as it has previously, adapt and evolve in this technological revolution. Importantly, we are supported in this work by the many countries whose governments are taking steps to regulate these technologies. The EU has led these efforts in developing the AI Act, with complementary efforts in the US and China. In short, most of us – within and beyond the publishing world – are striving to act responsibility. With our collective commitment preserving trust and establishing transparency in the face of this AI-driven transformation, we will develop collaborations, standards, and tools to ensure the long-term integrity of scholarly publishing.
Tim Vines – Response
Great generals are always prepared to fight the last war, not the current one. I’m sorry to say that my esteemed colleague arguing against the resolution has fallen into this trap: deploying the approaches we used to tackle yesterday’s problems against the entirely different crisis presented by Generative AI.
I absolutely agree with her assertion that there are good uses for AI – we can train great AI to support research, but that does not also mean that there are not bad uses for AI. There are people that seek to subvert and use up the process of research for their own benefit.
Moreover, it’s not as if we – the scholarly publishing community – have been particularly successful in tackling yesterday’s problems: the literature is infested with garbage from paper mills and image manipulation is rife, and we are only just waking up to the scale of this problem, having been warned about it for years.
Faced with Generative AI, each publisher has a choice to make. You can either invest heavily in ensuring that the work presented in your journals is real research that actually happened, or you can carry on as normal in the hope that the majority of work you publish is still real.
But here’s a warning: journals that don’t want to certify their research as real will steadily become repositories of fabricated junk, fatally undermined by AI. Will that be all of us? Or just most of us? That’s up to you.
Jessica Miles – Response
I want to start by acknowledging how important it is for us as industry to consider multiple viewpoints on AI and other emerging technologies, as doing so will ultimately help us mitigate the risks posed by these changes.
Nevertheless, I find several of Tim’s statements to be problematic.
First, he discusses science research to the exclusion of other domains. In doing so, he conflates domain-specific data integrity concerns with the matter of the integrity of the entire scholarly publishing enterprise. After all, an editor at a cell biology journal and an editor at a French studies journal may share concerns about emerging AI technologies, but the latter is likely less concerned about how to detect image manipulation in Western blots.
Even if we accept this myopic focus on science research, there are other issues with his account.
I find his assertion that “a substantial portion of scholarly publishers have no incentive to weed out plausible fake articles” to be not only morally troubling, but also not grounded in fact. I’ve already shared many examples of our community’s commitment to safeguarding the scholarly record – examples that contradict this account.
Beyond moral imperatives, STM publishers also have significant incentives to detect fraudulent submissions quickly. The time and money spent on these submissions represents an enormous waste of resource – including the precious, limited time and efforts of editors and peer reviewers, as well as costs of managing retractions and other integrity issues. Adding to this, publishers at publicly traded companies have seen earlier this year that the financial markets do not look kindly upon pervasive misconduct, the lesson being that they must do everything possible to prevent fraudulent research from being published.
He also claims, without evidence, that “the arms race of using technology to spot fake research texts is a race we have already lost.” As I said before, it is people, not technology like AI, that fuel these threats; as Dr. Bik noted in her opening: it takes a village. Publishers can, for example, continue to develop and scale approaches for detecting research fraud. Funders and institutions can decide to play a bigger role in oversight, by implementing their own systems for monitoring research integrity and imposing consequences for violations of publishing ethics or for retaliation against whistleblowers. In these ways, transparency begets trust, with trust leading to transformation.
Discussion
6 Thoughts on "SSP Conference Debate: AI and the Integrity of Scholarly Publishing"
Both points of view support the notion that trustworthy information is likely to become more expensive, due to the increased time/labor required to ensure its integrity.
In Borges’ tale, “On Exactitude in Science”, the mapmakers of an empire create a map so detailed it mirrors the world it represents, down to every leaf and pebble. It’s so precise, it eventually blankets the land it’s meant to depict, making the map itself redundant.
Applying this to Tim’s argument, he foresees an academic world so riddled with counterfeit knowledge, it’s like a Borgesian map of deceit. But he overlooks an intriguing possibility: what if technology could produce genuine scholarship, independent of human intervention? Pipettes and all!
While the threat of an overwhelming influx of fakes is disconcerting, there’s a deeper issue to ponder: What if we, like Borges’ cartographers, are engineering our own obsolescence? We might soon find ourselves living under the “map” of our own creation, with machines conducting both phony and legitimate science, and rewriting French history, be it fabricated or genuine. In such a world, will we still be necessary? Or will we just be vestiges of a bygone era, our roles claimed by the unerring accuracy of machines?
Thanks all, this was a fascinating live debate and discussion, nicely capture on TSK too – but aren’t you leaving us hanging a bit here on the audience poll results from SSP? it would be interesting to share the recording and see if a broader audience also came to the same conclusions.
I know Tim and Jessica put a great deal of thought and time preparing for this debate, and are great friends off the debate stage, we should all truly thank them both for their thoughtful contributions.
Finally Rick, I thought you very eloquently summed up the discussion at the end of the debate, paraphrasing that in society we can have open honest discourse and discussion, that can lead to people changing their minds, and respecting other opinions and perspectives. Great job everyone.
Seconding your statement that it would be great to see this debate.
” … journals that don’t want to certify their research as real will steadily become repositories of fabricated junk, fatally undermined by AI.” And this will be true of all publishers asserting a claim to content produced by humans: auto-biography, ethnography, etc. How will it be certified? Author certification, beginning with the publishing agreement? Auditing bodies?