Editor’s Note: Today’s post is by Isaac Wink. Isaac is the Research Data Librarian at the University of Kentucky. He recently graduated from the University of Illinois at Urbana-Champaign with an MS in Library and Information Science and an MA in History.
Generative artificial intelligence offerings such as ChatGPT are being retooled and developed so rapidly that anyone who attempts to write about them risks their words being outdated before they reach publication. As we reckon with how generative AI is shaping our relationships with work, information, and one another, it is worth trying to analogize our current experience to others, real or imagined, to see what perspective we might find.
I see one such opportunity to navigate issues of the veracity of AI-generated information in the short story “The Library of Babel” by Jorge Luis Borges. The story invites readers to imagine a universe composed of a massive number of hexagonal rooms, each of them containing walls of books, and each of those books containing a set number of pages with the same number of characters on each page. Borges establishes four rules for the Library and its contents (all quotations from the “Library of Babel” are taken from Anthony Kerrigan’s English translation found in Jorge Luis Borges, Ficciones (New York: Grove Press, 1962):
- “The Library is a sphere whose consummate center is any hexagon, and whose circumference is inaccessible.” The Library is likely not truly infinite, but from the perspective of the librarians who wander its rooms, it may as well be.
- “The Library exists ab aeterno.” Its relationship to the human librarians within it is unclear, but it is undisputed that it exists independent from humanity. It was not constructed; it simply is.
- “The number of orthographic symbols is twenty-five.” Specifically, the contents of the books are limited to an alphabet of twenty-two letters, a space, a period, and a comma.
- “There are not, in the whole vast Library, two identical books.”
From these rules, as the narrator explains, it follows that every possible combination of characters must exist somewhere in the vastness of the Library. Even though most of the books are incomprehensible to the librarians — save for a few snippets of coherent text on a single line — there must be some books that are perfectly legible and cover every imaginable subject, including revelations about the nature of the Library. (For a taste of navigating the Library, visit Jonathan Basile’s libraryofbabel.info.) The truth, every possible truth, is already written down somewhere in the pages of the right book. The problem, however, is that because every possible book can and does exist, even those books that are legible are not necessarily true.
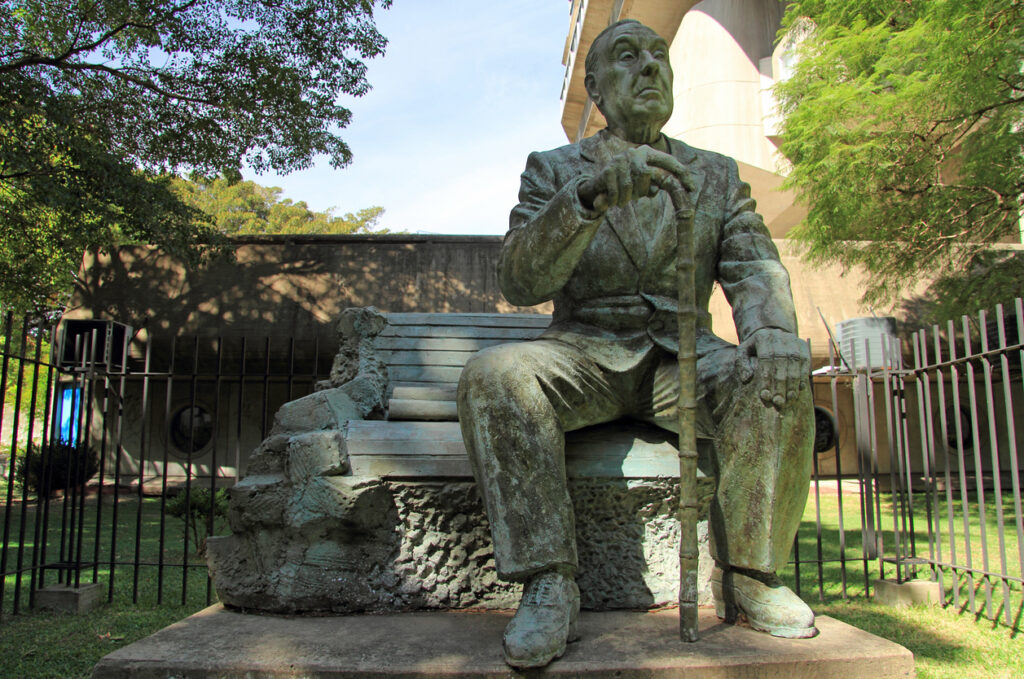
This situation has led to the formation of a number of sects among the wandering librarians. One of them seeks out the Vindications, “books of apology and prophecy, which vindicated for all time the actions of every man in the world.” The narrator claims to have discovered two such Vindications but admits that they may apply to fictional people who never have and never will exist. Applying a term that’s currently in vogue, we might say that the library has hallucinated.
The narrator is part of a sect that seeks a “catalogue of catalogues,” a guide containing the names and locations of other important books in the Library, which would enable the reader to identify other books that contain meaning. Even with the knowledge that every book is an accident of combinatorics, the sect still believes that it can locate truth inside one. And they are right! Based on the rules of the Library, there must indeed exist such a book.
Unfortunately, the narrator does not confront the problem that the contents of the Library have absolutely no relationship to any notion of truth. The librarians may successfully locate books that contain true information, but they will not be able to distinguish these books from ones that contain falsehoods that plausibly appear to be true. The contents of the books themselves will never be enough to confirm anything as true; they must be checked against circumstances out in the world. The only way to distinguish the true “catalogue of catalogues” against its nearly endless false versions would be to confirm that each book it references is in its designated place.
Moreover, each individual unit of information in a book must be taken independently. The fact that a catalogue has correctly listed ninety-nine books does not change the probability of its hundredth listing being incorrect. The narrator even takes this as a source of comfort. A rival sect has been destroying books in the Library, but even if they have destroyed the one true catalogue, there are plenty of books that are almost the one true catalogue, save for a few letters of gibberish on a page in the middle.
ChatGPT and other AI interfaces built on large language models are not quite the Library of Babel. Their outputs are not randomly assembled combinations, but rather predicted results based on training corpora that comprise massive amounts of human-created writing. They do not contain (nor are they capable of producing) everything, and when discussing them, we should be careful to keep in mind that they are technologies with a history, contingent both on the biases of the data upon which they are trained, the humans who make countless tiny choices that shape their development, and even the legal and cultural landscape that leads their creators to try to prevent certain kinds of outputs.
But I believe that as sources of information, large language models are more like the Library than they are other analogies we may reach for. They are not, for example, like calculators. I trust a hand calculator to give me consistently correct responses. And even for those calculations that are not fully correct — such as when a repeating decimal is rounded off — I trust the combination of the calculator’s result alongside my own knowledge of its heuristics to get me to the answer that suits my need. In other words, when a calculator is incorrect, it is incorrect in consistent and predictable ways. By contrast, when an LLM generates incorrect answers, it may do so much less predictably.
Nor is an LLM like a search engine (although it should be noted that companies are already strapping generative AI tools onto search results). Google can lead users to incorrect or overly simplistic information, but it typically does so with sources attached. Savvy searchers can evaluate the quality of a source returned and determine if it matches their information need. Furthermore, while search engine algorithms are constantly changing and difficult to understand, the healthy industry of SEO bait — sites optimized to appear at the top of search results — demonstrates that there are at least some basic rules that are well understood by website designers. While it is possible to understand why search engines rank certain webpages higher than others, it is significantly more difficult to understand the precise chain of causality that leads to a given output from a generative AI tool.
And finally, an LLM is not like a human. On a daily basis, we ask the humans in our lives to provide us with information, and on a daily basis, we receive responses that are as incorrect as they are confident. Clearly, we understand that we cannot take all information at face value and need to be skeptical of it, so why can’t we simply train ourselves to do so with ChatGPT?
The difference is that when it comes to human beings, we are generally able to distinguish between different individuals as accurate sources of different types of information. I trust my friend who has worked in a bike shop to give me high-quality information on bike maintenance and usually take his advice without checking it further, but I may be more skeptical of his recommendations in other areas and want to supplement them with my own investigation. Part of the marketed promise of tools built atop LLMs is that they can be a single source that can produce reliable information on any topic. OpenAI encourages this sense of endless possibility with sample prompts whenever a user starts a new session of ChatGPT: “Brainstorm edge cases for a function with birthdate as input and horoscope as output.” “Plan a trip to experience Seoul like a local.” “Tell me a fun fact about the Roman Empire.” This framing nudges us to trust ChatGPT as omniscient, a claim which many of us may want to believe and which makes it more difficult to identify the areas in which it comes up short.
But if individuals are growing more comfortable turning to generative AI as a source of information, we should be careful not to fall into the same trap as the librarians of Babel, who mistakenly believe that a true catalogue generated by accident is more valuable than an incomplete one created based on knowledge from the librarians themselves.
The act of trusting any source of information — a book, a website, a data collection instrument, a human being — is an act of believing that someone has checked the information against real-world circumstances. Reporters have spoken to witnesses to an event; researchers have conducted experiments; and engineers have tested and calibrated instruments to ensure their readings are sufficiently accurate. Of course, people lie or misinterpret observed circumstances, but in doing so, they are still making an appeal based on some form of external validation. By contrast, neither the books in the Library of Babel nor the outputs of ChatGPT have ever been checked against real-world circumstances. In a post announcing the public release of the research preview of ChatGPT in November 2022, OpenAI warned that it would generate “plausible-sounding but incorrect or nonsensical answers,” a difficult problem to fix because “during [reinforcement learning], there’s currently no source of truth.” ChatGPT’s predictive responses based on training data certainly prove more accurate than random combinations of letters, but they both lack a firm relationship to truth.
In addition to the issue of truth (which could end up being a solvable problem), another noteworthy similarity between the Library of Babel and generative AI tools is their quasi-religious promise to contain infinity. Borges’s narrator celebrates (or perhaps despairs) in the fact that in the Library,
“[e]verything is there: the minute history of the future, the autobiographies of the archangels, the faithful catalogue of the Library, thousands and thousands of false catalogues, a demonstration of the fallacy of these catalogues, a demonstration of the fallacy of the true catalogue, the Gnostic gospel of Basilides, the commentary on this gospel, the commentary on the commentary of this gospel, the veridical account of your death, a version of each book in all languages, the interpolations of every book in all books.”
Considering the training data behind LLMs is a similarly dizzying experience, so much so that the creators of a significant open-source corpus of English text for LLM training have simply called it the Pile. The Pile may not contain everything, but it nevertheless contains quite a lot: the OpenWebText2 web scrape corpus; the contents of repositories such as PubMed Central, ArXiv, and GitHub; subtitles for movies, TV shows, and YouTube videos; all of English Wikipedia; collections of math problems; the Enron Emails dataset (one of the earliest high-quality sources of textual data for model training); and most controversially, Books3, a collection of nearly 200,000 books, including in-copyright titles.
The collectors of these datasets are groping towards the infinite, or at least a complete corpus of all human writing. When considering generative AI as a sociocultural phenomenon, whether they get there is beside the point. The text going into LLMs already feels as infinite to us as the books of the Library of Babel feel to the librarians. Lately, complaints about ChatGPT’s abilities often blame tweaks by OpenAI attempting to limit harmful outputs, the implication being that the model is capable of significantly more than its guardrails allow. Questioning the glaring gaps in the data that power generative AI tools as well as the ethics of including content without creators’ consent is an important pursuit, but we should also ask whether attempts to endlessly expand their training data — even with proper consent from creators — would even be desirable.
The tragedy suffered by the librarians of Babel is that they are so tantalized by the certainty that anything they could possibly hope to read exists somewhere in the Library that they have oriented their society around attempting to find the right books, not realizing that there is so much text with the aesthetic appearance of truth that the books themselves will not serve their goals. As social, cultural, and individual relationships with generative AI tools continue changing rapidly, we should be careful avoid the same tragedy. We may view generative AI as one method among many for understanding the world, but we should not mistake it for the world itself.
Discussion
1 Thought on "Guest Post — The Truth Is in There: The Library of Babel and Generative AI"
I love this analogy! What a fascinating way to reframe how we think about these tools. Another reframing of the topic that I’ve found particularly useful was to stop saying, “AI did X” or “AI made Y,” because in doing so we attribute a certain amount of autonomy to the tools and do not then hold accountable those who developed / trained / prompted the tools for their outputs.