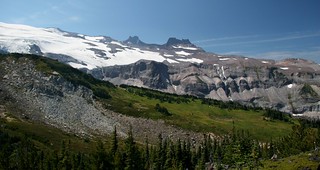
The recent data policy outlined by PLOS and now being enforced is important not only owing to its prominence, but because it makes real for many a good number of the data-centric ideas swanning about these days, many of which have to do with data sharing and data publication.
It spurs an important discussion that may be a bit overdue.
The idea of sharing data has immediate appeal. It sounds scientific, commendable, and direct, as opposed to written reports, which are one step removed from data, and have been criticized in the digital age for obscuring data, hiding it behind old-fashioned graphical charts and tables.
Mainlining data sounds better than reading papers.
Yet, data may turn out to be just as variably applicable and elusive as anything. In some fields (e.g., astronomy), data provide the lifeblood of the field. Most modern astronomers perform their research by analyzing data rather than peering through lenses. Other fields also have strong linkages to direct data observation, such as computational biology and various mathematical fields. For these researchers, data and empiricism are tightly linked.
In fields where direct observation of physical reality can’t be as direct, empirical observations yield data that can be difficult to reconstitute into the conditions that generated them. In these fields — medicine, molecular biology, other biological fields, physics, and the humanities and social sciences — the results of observations can lead to some interesting data, but direct observations either can’t be captured in sufficient detail to be saved in anything approaching a complete manner, or are subject to so many conditions that the data can only provide a basis for interpretation and correlation. This is where statistics can save the day, but that is just another form of interpretation at some level — how you choose to analyze the data is an interpretation, and others might use different techniques. Combining data from separate observational occurrences can be extremely treacherous, given all the conditions, confounders, and temporal aspects to biological systems and populations.
In medicine, approaches to combining the findings from multiple trials have not lived up to expectations. Evidence-based medicine has proven of more modest utility than its proponents envisioned when they started, and clinical guidelines have devolved into a confusing array of competing interpretations of the underlying datasets, leaving clinicians with the chore of choosing which guideline to follow. If data spoke as clearly as we believed, there would be no difference.
How much data to share is unclear. PLOS attempts a definition in its policy when it comes to a “minimal dataset” or:
. . . the dataset used to reach the conclusions drawn in the manuscript with related metadata and methods, and any additional data required to replicate the reported study findings in their entirety. This does not mean that authors must submit all data collected as part of the research, but that they must provide the data that are relevant to the specific analysis presented in the paper.
As one blogger points out, even this carefully crafted statement leaves a lot of room for interpretation and creates potentially significant burdens or unrealistic expectations for researchers in some fields:
Most behavioral or physiological analysis is somewhere between “pure code” analysis and “eyeball” analysis. It happens over several stages of acquisition, segmenting, filtering, thresholding, transforming, and converting into a final numeric representation that is amenable to statistical testing or clear representation. Some of these steps are moving numbers from column A to row C and dividing by x. Others require judgment. It’s just like that. There is no right answer or ideal measurement, just the “best” (at the moment, with available methods) way to usefully reduce something intractable to something tractable.
Data sharing initiatives come down to “what is the point.” Some argue that providing the underlying data is about validation of a published study. But data can be fabricated and falsified, and sharing falsified data that fit published conclusions does little but validate a falsehood. Also, if your analysis of my data doesn’t agree with my analysis of my data, where does that leave us? Am I mistaken, or are you?
Arguing over data can be a form of stonewalling, as the fascinating scandal at the University of North Carolina (UNC) shows. The whistleblower, Mary Willingham, is not a researcher, but used research tools and methodologies to identify reading and writing deficiencies in high-profile athletes attending the university and making good grades in sham classes. An administrator attempted to discredit her research by selectively and publicly picking apart the data. As Willingham is quoted in an excellent BusinessWeek article:
Let’s say my data are off a little bit. I don’t think they are, but let’s say they are. Set aside the data. Forget about it. The . . . classes were still fake, and they existed to keep athletes eligible.
Data don’t always contain the most salient conclusions of a study. Conclusions can rely on data, but sometimes they go beyond the data.
While data sharing for some is about validating results, for others, publishing data is about enabling big data solutions and approaches.
David Crotty’s post yesterday points to this cultural belief system around data-sharing, one which emanates from Silicon Valley — namely, the belief that data can be accurately parsed and understood if you have enough of it. This belief system has led to some amazing inventions and tools when the data are actually close to some empirical reality — GPS, text searching, and so forth — but data accumulation has many potential weaknesses, not the least of which is the problems of how far data can become divorced from reality over time, how flawed data may go uncorrected, or how reliance on data might impede simpler and more direct empirical observations.
We’ve become somewhat beholden to these technocratic, data-driven impulses in our daily lives. If you’ve ever watched the weather radar on television or on your phone rather than looking out the window — or, more likely, ever argued with your device when it’s not raining outside but the radar shows your area covered with a green splotch — you were substituting data for empiricism. Or, if you’ve ever arrived at an address using GPS to find that the store or facility you expected to be there has either ceased to exist or has moved, you’ve seen the power of empiricism over data firsthand.
What is not measured is also a concern. It’s easy to miss an important fact or skip measuring phenomena in a comprehensive, thoughtful way. Michael Clarke covered this well in a recent post, noting that many measurement tools fall short in rapidly changing environments. In medicine, many long-term studies have reached a point of diminishing returns not only because their populations are aging and dying, but because the study designs did not take into account how relevant race or gender or class might prove, resulting in studies that are dominated by Caucasian men or affluent women. After decades of data collection, such data sets are not aging well, and ultimately will become relics. What factors are the large data sets of today overlooking? What regrets will our medical research peers have in their dotage? What biases are shared when we share data?
Privacy is also a concern (as noted in a comment yesterday, with an emphasis on the Helsinki Declaration), and big data invites unanticipated consequences. In yesterday’s comments, we were also reminded of the researcher who in 2002 was able to use anonymized patient data and publicly available records to identify the health records of the Massachusetts Governor at the time, William Weld. We also all know the story of the Target pregnancy reveal, where Target sent a teenager marketing normally reserved for pregnant women, based on heuristics around her purchase habits (switching to unscented lotions, for example). Her angry father was chagrined a month later when it became clear Target had been correct.
Publishing data can lead to unintended consequences, including privacy violations. PLOS’ data policy has a statement about this, and the policy’s authors were definitely thoughtful on the point. But this only confirms that there is a big problem with any broad data-sharing policy — namely, that any single data provider cannot definitively know if their data, in combination with other data, could enable privacy violations. A single relatively benign-appearing data set might be the key unlocking a half-dozen other data sets. Hence, policies that require publication of data leave the door open for major unintended consequences, as they create scads of data with no single point of accountability but many potential points of exploitation.
The siren song of data is seductive. However, an environment filled with published data needs to have a clear purpose. Is it to validate published reports? If that’s the case, then it has a time-limited value, and should be treated accordingly. Is it to enable big data initiatives? If so, then we need controls around privacy, usage, and authorization. As with everything else, the relevance and utility of data depends on who you are, what you do for a living, and what is possible in your field. Assuming that “data are data” can obscure important subtleties, major issues, and leave us unprepared for dealing with flawed or fraudulent data. And we need to compare the risks, costs, and benefits of these initiatives in science with the risks, costs, and benefits of simply recreating published experiments using actual, empirical reality.
In some fields, data are strongly tied to empirical observations. Those fields already have robust data-sharing cultures, and are actively seeking to make them better. Other domains are more driven by hypotheses, overlapping observations, and intricate interconnections of incomplete datasets that practitioners have to weave into a knowledge-base. Is their approach wrong? The technocrats who believe that “data are data” might argue it is. But unless we’re sure that “data are reality,” it’s probably best to keep our central focus on the best possible direct empirical observations. Data are part of these, but they shouldn’t become a substitute for them.
Discussion
39 Thoughts on "Data Sharing and Science — Contemplating the Value of Empiricism, the Problem of Bias, and the Threats to Privacy"
In regulatory parlance the PLoS difinition of the required dataset is “void for vagueness.” In most cases the concept of data is too vague to support mandates like this. Even worse the definition seems to reflect a simple minded view of science, namely that the results of research are obtained by turning a well defined crank on a bunch of measurements. The reality is that research results are based as much on judgement as on data, often more so.
Given this vagueness the likely result is that people will simply dump something into an archive and cite it in their Data Availability Statement. There is also the potential for harassment in the form of complaints that that author’s stated results do not all follow simply from the data. They seldom do.
Why data’s limitations should be an argument in discussions on data sharing policies? Each data set has it’s limitations, but they are clear if we know the methodology of researches that have generated particular data. If description of methodology is provided, access to data set is always a plus and could only help. The privacy is, in my opinion, the only problem with data sharing in humanities and medical sciences. But I am an optimist. Open Data pilot, which is a part of Horizon 2020, may bring more guidlines that will help us to solve this issue.
I wrote more about Open Data pilot here:
http://openscience.com/horizon-2020-funding-opportunity-sensible-approach-open-data/
Access via a repository is not necessarily a plus because you have to include the cost and burden of the many datasets that are not accessed. If only one set in a thousand is ever used then this is a large unit cost.
Nor does sharing require a repository. Getting the data from the author is sharing and far simpler than requiring repository deposit.
Repositories may come into play for use by groups looking to utilize large quantities of data. Just the other day Google Genomics was announced (https://developers.google.com/genomics/). A company like Google or a pharmaceutical company looking to do bulk approaches, or an insurance company looking for data to use to set policy rates would all be better served by access to everything in one spot, rather than having to go to each individual researcher to ask for data.
But as you note, there’s still the question of cost. Should researchers and authors be paying the price for private companies to use their data to drive profits?
These are other points I thought about including, but the post was so long I decided to punt on them. Basically, there are commercial interests that want free data just like General Motors wanted cheap gas — to drive adoption and reliance, and to maximize their profits. Google has a long history of supporting, through nudges and the occasional elbow, free information market tendencies. It all sounds good until journalism goes away in your locality because nobody was paying, or until wages stagnate while stock prices go through the roof. The Matthew effect is huge in our world now, and technology companies seem unstoppable. Feeding them free data won’t slow them down.
For reviews of two books talking about these issues, see:
“The Entrepreneurial State: Debunking Public vs. Private Sector Myths” — http://scholarlykitchen.sspnet.org/2013/08/19/book-review-the-entrepreneurial-state-debunking-public-vs-private-sector-myths/
“Free Ride: How Digital Parasites are Destroying the Culture Business and How the Culture Business Can Fight Back” — http://scholarlykitchen.sspnet.org/2012/07/26/review-and-discussion-free-ride-how-digital-parasites-are-destroying-the-culture-business-and-how-the-culture-business-can-fight-back/
One point that hasn’t been mentioned is the source of the data. If researchers are accessing data collected by someone else, for example buying an existing dataset, there may be strict conditions over how it is used and disseminated. They may not have permission to publish it as raw data as part of their study. There may also be problems with giving data that is ‘free’ e.g. voter registration data, and giving it to a commercial entity e.g. PLoS as part of a paper.
The PLoS rule is that external data sources are cited in the Data Availability Statement, so external data is not included in the author deposited set. Which external data was used and how it was used will presumably be part of the author deposited set, a significant burden to specify I would think. Also where many sources are used I can see the DAS being longer than the research article. I have done projects with well over a hundred data sources.
My basic point is semantic, David C. People are using the term “data sharing” to mean repository deposit, along the lines of the PLoS mandate, which rules out simply sharing one’s data on request. Of course repositories can be important and useful. This is measured by the extent to which they can attract funding. The question is the soundness of forcing authors to pay.
Speaking of cost there is also the indirect cost of the burden. You pointed out yesterday that repository deposit takes a lot of a researcher’s time. A number of discoveries could be lost by that diversion. This is the symmetric counter argument to the pro-data argument that making all data publicly available in repositories will lead to some new discoveries. Not only is data not free, neither are discoveries.
Data’s limitations suggest the rules, policies, or practices that need to be put in place for sharing to work. Also, data’s purposes need to be more clearly enumerated. Blanket sharing with no suggestion that rules about data usage, data aggregation, privacy, or commercial use is probably asking to learn the hard way.
A good question that came up on the comments was the lifespan of data. When one puts data in a repository, is the plan to preserve that data and keep it available forever? I think of all the expense publishers go through when we update our systems, converting and reconverting old files to new types, modernizing back issues of journals to keep them available. Will anyone make a similar effort for archived data? I can think of lots of my old data that are all in no longer extant file formats or that would require proprietary software that is no longer available in order to access them.
Is there a reasonable lifespan for data? I think back to my thesis work, and if for some ungodly reason I were to pick it up again, it would be much faster and much more reliable for me to start from scratch and generate new data than it would be to try and use what I did 20 years ago. The costs of generating it anew would also be much lower than it would have been to store that data for the last 2 decades.
These large update expenses were a major issue with the IWGDD because some of the members run the Federal repositories that would be tasked with mass data storage. As I recall the rule of thumb is that everything has to be re-ported every 5 years. This is why I am skeptical of repositories with flat deposit fees.
You make a good point about file formats here: any data archived in a proprietary format isn’t. Archival formats must be fully published.
Much of the discussion over the new PLoS policy is getting stuck in the nirvana fallacy (http://en.wikipedia.org/wiki/Nirvana_fallacy). It’s clear that many papers published by PLoS would be stronger if the data were also shared, as then readers can validate the results and conclusions or use the data to test new ideas. There are certainly cases where data sharing is difficult or where the data are hard to define, but that doesn’t meant that overall the policy is a bad thing. The current situation is that results in most papers cannot be checked and most scientific data are lost after a few years, so if the PLoS data policy improves that then it’s a step in the right direction.
Next, it’s impossible to define what constitutes ‘the data’ for the massive range of papers published by PLoS, but, as PLoS correctly identifies, it’s much easier to define ‘the data’ for a particular paper. This requires that the authors state what data they plan to share and where they plan to put it, and then the editors and reviewers weigh in on those plans. This in turn will build a consensus for each field on which types of data ought to be shared.
There is a lurking unintended consequence: some datasets are very labour intensive to produce and can take many years to come to fruition. If authors feel that they can only realistically get one publication from these data before being forced to release it to the public, this demotivates the collection of these important datasets. This fear stems from the idea that authors will immediately be scooped (without being offered authorship) on all future publications based on the data, and only time will tell if this is a realistic. Preliminary data collected by Heather Piwowar on data reuse for GEO datasets suggests that there’s a lag of 2-3 years before anyone else starts using the data (slide 41 on here: http://slidesha.re/1ncRybl).
Where do you see the nirvana fallacy Tim? Can you give an example?
Regarding your claim that “It’s clear that many papers published by PLoS would be stronger if the data were also shared, as then readers can validate the results and conclusions or use the data to test new ideas.” This is not at all clear to me. Isn’t this precisely the claim that we are debating (given the cost and burden of course)?
Also I note that you seem to be using “shared” to mean deposited in repositories, rather than shared on request. Lots of data is already shared among researchers. The question is whether we need mandates specifying universal repository deposit, a very specific and expensive form of sharing. Sharing per se is not the issue.
This is where I see the nirvana fallacy: commenters are comparing the new PloS policy to some mythical perfect data policy, and denigrating it on those grounds (drugmonkey’s post is a good example: http://bit.ly/1cniIpo). The correct comparison is the current situation, where almost no PLoS papers archive their data.
Are papers that archive their data better than papers that don’t? This is a non-question, in my opinion. Are papers that contain tables and figures better than those where the authors make the tables and figures available upon request? If the raw data and the analysis code are not shared, the authors are effectively saying ‘trust us, we’re sure we did it right’. Experience suggests this is not true for at least a quarter of published papers. Moreover, some data are irreplaceable, often because they are unique to a time and place. Trusting authors to preserve these datasets more or less doomed them to be lost within twenty years, and it’s only after that much time do they start to become really valuable.
And yes, we need mandates stating that data must be placed in public repositories at publication. Trying to get data from authors is extremely difficult, particularly for older papers.
Well Tim, I cannot speak to the rest of the blogosphere but that is not true of the discussion here in the Kitchen, which is what I thought you referring to. No one here is “comparing the new PLoS policy to some mythical perfect data policy.” We are questioning the PLoS policy as it is. I personally question the need for any new policy whatever, which is the very opposite of what you allege.
As I see it you have made no argument for a new policy except an argument by assertion. As with any regulatory scheme the key issues are cost and burden versus benefits. You seem to ignore the issues of cost and burden completely, even though they have been discussed repeatedly here in the last two days. At the same time I find you claims about benefits to be fairly mythical.
If you are claiming that it is somehow cost beneficial to collect and archive all the data from every scientific study done in the world for twenty or more years then I simply disagree. Pointing out that there are instances when having such data might indeed be useful misses the point completely, because most of that data will in fact never be used. Thus there is a huge element of waste to these policies, waste of money and waste of researcher’s crucial time. Waste is not good policy.
“I personally question the need for any new policy whatever”.
OK. Which of these points do you find mythical:
1) Some papers contain mistakes in their analyses that render their conclusions invalid. Some of these papers are published in PLoS.
2) This is a problem for scientists trying to base their own research on the conclusions of previous papers
3) It is very hard to definitively identify flawed papers unless you repeat their analyses
4) It is impossible to repeat the analysis unless you have the raw data.
5) All papers should therefore have their raw data and code available so that their conclusions can be verified.
6) At present, less than 20% of PLoS papers make their raw data publicly available
7) The new policy is needed to increase the proportion of papers that have their data publicly available.
With respect to costs and benefits, we’ve discussed this before:
“Thus there is a huge element of waste to these policies, waste of money and waste of researcher’s crucial time.”
This paper lays out the cost of storing the data from a typical paper on a public archive for ~50 years, and it’s about $100: http://researchremix.wordpress.com/2011/05/19/nature-letter/
A broader point is that it’s a bigger waste of researchers’ time to base their new research on flawed work than it is to make the original researcher get their data into a shareable format and put it on a server.
Number 4 is where you lose me, because you’re talking about a small subset of a particular type of research. It is 1) quite possible to repeat the analysis for many types of research without access to the raw data, and 2) access to the raw data does not automatically enable one to find every possible mistake that was made.
1) If I’m a cell biologist, and I’m stating that chemical X causes increased cell division in cell type Y, you can’t repeat that analysis from looking at the list of numbers that makes up my raw data. To repeat my experiments, you must grow the cells and expose them to the chemical and record the results. If anything, you’re better off without my data potentially biasing your results.
2) For experiments where the data I have collected is flawed in some way, then re-analyzing that flawed data will lead you to the same flawed conclusions I have previously reached. Let’s say I made my cell culture medium wrong and it was way too acidic because I grabbed the wrong bottle off a shelf. If you just look at my data, you’d have no way of knowing that, and my incorrect conclusions would seem fully justified.
Point number 5 above is indicative of the sort of experimental myopia to which scientists often fall prey. Not all experiments should have their code available because not all experiments include any code whatsoever. To the computational researcher, the world is just a bunch of numbers to be run through an algorithm. To the psychological researcher, a careful set of observation notes that makes up a case study is not something that can just be re-run.
Hence the issues with this policy. I think you and I are in agreement–in the big picture, the policy is a good thing as it causes debate and will move things forward to creating better policies. But as it stands now, it’s not a realistic policy for many reasons, discussed in yesterday’s post and all over the blogosphere. It works really well for some types of research and some types of data, and is completely irrelevant, if not deeply problematic for others.
I think we’re talking about two different things here. I’m talking about repeating the _analyses_ that were conducted on the data that lead the authors to their conclusions. You’re talking about repeating _experiments_. Both are important if you’re basing your work on their results, but for the former you need the data, even if it’s just two rows of numbers.
For example, the cell biology paper you describe above contains a table with the average acidity needed to halt cell division, and there’s also a figure with the same data plotted against some other variable. Unfortunately, the means in the table don’t look like they’re right, given the scatter in the figure. Maybe they’re the median values and the authors got it wrong? Also, the scatter in the figure is very wide, and sometimes the pH needed to be much lower to stop division. The authors gloss over this and just present a single number.
Without the raw data, you shrug and hope to muddle through when you try to repeat their experiment yourself. If the raw data are available with the paper, you can a) recalculate the means and medians, and work out what happened, and b) see if the outlier data points have something in common. You can then make a much more informed choice on whether to base your research on their results.
The code is important in the above example as well. More and more data manipulation and statistics are carried out in R (a text based program), and even trivial files that calculate means and variances are useful to readers who need to get to grips with the paper. In the example above the authors may have typed tapply[data[,1],mean] when they actually meant tapply[data[1,],mean], and that explains why they got it wrong. The code is also incredibly useful if you want to see which replicates etc they excluded from their analyses, as this is seldom clearly stated anywhere else.
What if my data was in the form of time lapse movies, and I spent 4 hours a day every day for 2 years counting cell divisions in movies. Is it reasonable to expect anyone else to spend that same amount of time double checking my work? What if I recorded the numbers I counted on a spreadsheet. Do you need the raw data, the petabytes of movies, or do you need the spreadsheet? If the latter, then the statement, “It is impossible to repeat the analysis unless you have the raw data,” is indeed mythical.
And you’re talking about a small fraction of articles and a small fraction of the types of mistakes a researcher can make. If my article is flawed because I collected accurate data but analyzed it wrong, say I accidentally moved a decimal point over one space, then yes, you could catch that from checking my math. But if it’s any other kind of mistake–I used the wrong type of cells, I made my solutions incorrectly, the thermometer on my incubator is off, then reanalyzing my flawed data will still lead you to the same incorrect conclusions.
You suggest a valid case where having the data would be helpful, but it’s an edge case. As David W points out, do these rare events justify the enormous costs and efforts required in making everyone make everything available to everyone? Is it better instead to focus on particular types of data where these types of errors may be more common, types of data where this type of re-analysis is both relevant and readily done? Types of data where reuse is more feasible and promising?
There’s a lot of these ‘what if my data is massive’ questions going around. Sure, there are some types of data where it’s impossible to put the raw data onto a public archive, in which case the next step along (in your example the spreadsheet) should be shared.
Clearly, a GoPro movie of the entire experiment would be the ultimate way to find the majority of errors, but it doesn’t follow that being able to detect errors in the data analysis isn’t worthwhile. Having less than perfect stats is most certainly is not an ‘edge case’ – for example, it affects about 2/3 of our submissions, and I suspect that’s true of most fields where statistics are routinely used.
For what it’s worth, I think you’re massively underestimating the number of fields where most papers have a) a shareable dataset (<10GB), and b) stats complex enough to make reanalysis worthwhile. This would include all clinical trials in life sciences, and all sciences where there is substantial statistical noise in observations (genetics, evolution, ecology, agriculture, geography etc). I'd exclude 'hard' fields like maths, physics and some parts of chemistry, and others where conclusions are based on just a few observations (surgery etc). However you want to slice it, this is not a 'small fraction of articles'.
Lets eliminate the size question then–let’s say my data consists of 6,000 small jpegs. Likely a reasonable amount of file space to be up- or down-loaded. Again, it’s irrelevant to the kind of analysis you’re talking about, where you’re not reviewing my raw data, you’re reviewing my analysis. You’re looking at the spreadsheet derived from my raw data, so why should I be obligated to publicly post the raw data that you’re not going to delve into?
Again, this statement, “It is impossible to repeat the analysis unless you have the raw data,” is indeed mythical for the sort of analysis you’re talking about. Unless you’re going to go back to the original images and count cells and confirm every single number on my spreadsheet, you don’t need those raw images.
And for this type of analysis, shouldn’t the spreadsheet be part of the paper itself, rather than uploaded to an unrelated archive? Why not either publish it in the paper or in the supplemental data?
I don’t mean to belittle the number of articles with statistical and/or quantitative data where this sort of analysis might be helpful. What I’m suggesting is that those types of papers in those areas of research with those types of data might be a better starting point than declaring everything en masse must be made available. Much has been learned from making DNA sequence data, microarray data, crystallographic structures available. Why not continue to expand in an iterative fashion, building upon those lessons to include more and more data types, rather than asking for everything in one fell swoop, with the seemingly near-infinite number of complications that arise?
This sort of iterative approach is what the IWGDD opted for, because each field is handling its data needs in different ways. The idea is to see what each community will support (and fund) as a measure of the importance of each data type.
Regarding funding I have been looking into Dryad and found something interesting. Its start up was, and still is, funded by the US NSF. NSF is famous for starting operations like this then cutting them loose to see if they can survive. They appear to have a terminal spin off grant ending in 2016. See http://wiki.datadryad.org/NSF_grant_2012-2016. They hope to scale up enough to be self supporting, which means going far beyond their evolutionary biology origins, for which see http://wiki.datadryad.org/NSF_grant_2008-2012.
Perhaps NSF asked PLoS for help in this spin off; certainly somebody did. The point is that there may be a real possibility that Dryad will fold in 2016 when their grant runs out and that the PLoS mandate may be politically motivated. In any case the case is far from simple. Dryad’s overall grant structure is here: http://datadryad.org/pages/organization#funding.
Tim, your number 5 simply does not follow from 1-4. In fact whether 1-4 justify 5 is one of the central issues being debated. The key question is how often will verification be done? (In some fields I doubt these analyses ever occur.) Suppose verification is done on one in 1000 papers. Given your $100 number that is an archiving cost of $100,000 per verification. David Crotty mentioned a burden of two weeks of prep labor per article, which gives 2000 weeks or about 40 years of researcher data prep labor per verification, an astronomical number. Even if it is one paper per hundred, which I doubt, the cost and burden numbers are huge.
What I see as mythical is the implicit idea that science is going to devote huge amounts of time and money to verification studies (at the expense of discovery). Moreover there are well established methods for analyzing regulatory cases like this. These methods need to be applied.
David- go look at how often the average data file on Dryad is downloaded. I picked three random papers from Mol Ecol from 2012. The data files were downloaded 69 times (doi:10.5061/dryad.6pq7jg8p), c. 32 times (doi:10.5061/dryad.ks6g0), and c. 35 times (doi:10.5061/dryad.27q72). These numbers exclude bots etc, so it’s real people each time. Clearly, people are interested in these datasets, and I’m willing to bet that someone has tried re-analysing them each at least once each.
Under your “suppose verification is done on one in 1000 papers” scenario, I’d have needed to search through hundreds of datasets before finding one that had been downloaded more than a few times. That’s clearly not the case, at least in this field of science (where data archiving is now the norm).
So, suppose verification is done on 1 in 1 papers (as it appears from the Dryad downloads above). That’s $100 per verification, or about two weeks of labour prep per verification. For my own experience, I think David’s two week estimate is quite high – prepping these two (admittedly simple) datasets for sharing took 3-4 hours each: doi:10.5061/dryad.q3g37 and doi:10.5061/dryad.6bs31 ; that may be because I knew I had to share the data so curated it accordingly from the start.
I agree that formal verifications appear to be rare, but perhaps because there’s no real way to make these public at the moment? We also likely only hear about verification attempts when readers are sure they’ve found a fundamental flaw and feel that it’s serious enough to warrant a formal write-up.
“What I see as mythical is the implicit idea that science is going to devote huge amounts of time and money to verification studies (at the expense of discovery)”
I’m going to contend that this is because you’ve never actually done any science. Informal verifications are part of day to day research – if a paper is published that’s absolutely core to your research, you go get the data and fiddle around with it. Maybe you replot it for a talk you want to give. Maybe you try an analysis that the authors didn’t include. This time is most certainly not wasted, as it allows the researcher to develop a deeper understanding of what was discovered, and moreover helps identify papers that are flawed and should be ignored.
More broadly, applying cost/benefit analyses to each component of science is ridiculous. It’s not a factory. Researchers (especially junior ones) spend years battling failed experiments and pursuing duff ideas, and this has to happen if you’re trying to fumble your way towards an understanding of the natural world. Spending a few hours fiddling with the data from an important paper is a bargain by comparison.
Given that what’s on Dryad now consists of early adopters, how well do the data types represented reflect the overall nature of the broad and diverse research community? Essentially, who puts their data up on Dryad, and what type of data is it? Is it representative of all data types?
I think a desire to ferret out the cause of strange numbers or analyses in a published paper is going to be fairly universal, particularly for junior researchers trying to get to grips with their area. As the Rogoff-Reinhart take-down shows, there’s also plenty of reputation to be gained from picking apart a cherished result. So, yes, the data on Dryad comes mostly from ecology and evolution, but I don’t think that researcher in that field are unusually interested in exploring others’ data.
One other point I’ve not made above and that I think is important: the prospect of having to share your data should make sure that researchers are more careful in their analyses and conclusions. This additional care will raise the quality of published papers even if the verifications don’t happen – the prospect is motive enough.
Tim, I am not trying to apply cost benefit analysis to science, rather to a specific form of regulation of scientists. (And this application itself is science.) You are basically claiming that every published paper, about two million a year, will be subject to verification under your regulatory scheme. The obvious question is what evidence do you have that these Dryad downloads are for the purpose of verification? Given that PLoS named Dryad as their default repository I would expect great interest in what a Dryad deposit looks like. Have any of the papers you looked at issued retractions or corrections?
Another way to approach it is what fraction of published papers have issued corrections or retractions? My understanding is it is very small, less than one in a thousand. Are you claiming that on the contrary most published results are actually seriously wrong? If not then all this verification effort you seek would itself be waste, would it not?
But it now sounds like you are suddenly interpreting verification to mean simply looking at the data. We know there are lots of downloads but they are probably a form of usage, not verification. What they are for is a very different issue from verification, another type of purported benefit to be explored separately.
I hope at least that you can see the empirical assessment issues here. Regulatory cost benefit analysis is science, not something you can simply wave off with guesses.
And by the way I have done a lot of science, unless you do not recognize cognitive science as science.
We started having this discussion (see above) because you thought that researchers would only use a tiny fraction of publicly shared data: “Pointing out that there are instances when having such data might indeed be useful misses the point completely, because most of that data will in fact never be used.”
Given the Dryad downloads, there’s no support for your assertion that most of the data will never be used.
I also not claiming that researchers will perform formal verification attempts on all published papers.
For the proportion of papers where there is a substantial amount of data and subsequent analysis, I expect that the data will be downloaded at least a few times. For ones where readers have issues with the results, the data will get a closer inspection, and if they smell a rat, this may evolve into a formal verification that may end up being made public.
Using the frequency of published comment pieces as a proxy for how often researchers attempt verification is therefore going to be a massive underestimate: only a subset of the cases where there are major errors in the analysis will result in a public comment.
As you recognise, researchers aiming to do examine new ideas with the data will be another source of downloads.
All in all, it looks like archived research data will get used – most of it a little bit, some datasets much more. Since it costs very little per use [(cost of data prep + cost of preservation)/no. uses], it seems like a good investment. Do you have any data that contradicts this reasoning?
I do not have the contradictory data before me Tim, but as I understand it, it goes like this.
1. The majority of papers are not read at all. Surely their data is not going to be downloaded for verification.
2. Most of the remaining papers are only read a few times. Moreover most reading is cursory. So it is unlikely that most of these remaining papers will generate laborious verification attempts.
3. 1 + 2 equals almost all published papers, for which it is unlikely that very few if any will generate verification attempts.
4. Papers generating 30 to 60 data downloads are therefore outliers, even if most Dryad papers fall into this category. Why they are getting so many data downloads is an interesting question.
On the other hand if the average paper is read 100 times or more then I am just wrong and have misunderstood the industry data.
BTW I never mentioned published comment pieces.
Tim, regarding your three sample papers the numbers indicate that these must be very highly read papers, which in turn are very rare cases. If say one in ten readers then goes to the trouble to explore the data then your examples are read from 350 to 690 times. The fraction of papers that are read that many times is very small, probably along the lines of my one in one thousand estimate.
My understanding is that the majority of papers are never read at all and there is a power law distribution thereafter so the number of papers drops very quickly with times read. This suggests another way to approach the problem of estimating how many papers will actually receive verification analysis.
Like I said, I recommend going to Dryad to see for yourself. I picked another ten papers (from any journal) that put data onto Dryad in 2012 and none of the datasets were downloaded less than 30 times, and most were closer to 50. The probability that the frequency of papers with highly downloaded data is indeed 1 in 10, is (based on these observations) 1×10^-13, which is a very small probability indeed. Much more likely is that the majority are downloaded this much.
I don’t have access to the Dryad download numbers. I expect that downloads of datasets also follow a power law, but even less popular papers still attract a fair number of downloads over time.
http://libraryattack.com/?p=519
A common issue with data scientists is that they often have the analytic and technological skills, but lack the domain expertise.
It seems to me that we are discussing technological solutions to a sociological problem. Specifically, how much of this conversation would be necessary if scientists actually engaged in open discussions among ourselves rather than communicating only in smoke-filled back rooms of grant review- and tenure committees, or worse via press releases?
How many academic scientists now speak openly about their work at conferences? Has this always been the case? For me, face to face conferences are fast becoming irrelevant, since most data presentations are either already published or are purposely kept so vague and opaque that I can get more information from a Google search. Mechanisms are in place already to facilitate post-publication peer review, but I cannot recall ever seeing a single comment in an open access article in the biomedical sciences.
On a different but related note, I suspect that comprehensive data disclosure requirements will ultimately choke off what is already an extremely limited flow of information from commercial labs. Clinical trials data aside, there is a wealth of high quality information that goes unreported by biotechs and Pharma because of potential risks to intellectual property, fear of litigation, and concerns that scientific second-guessing will hinder regulatory approval.
Putting on my hippie hat, perhaps we scientists should act a bit less like hyper-possessive, thin-skinned cave dwellers and focus instead on our shared goal of reducing suffering and ignorance? This would likely require a re-jiggering of our present risk-rewards system, and we would probably have to share more of our Mastodon meat than we would prefer, but that seems worth the risk of an alternative fate, irrelevance and unemployment.
Is it a sociological problem or an economic problem? The behaviors you discuss above come from there being more people who want to be scientists than there are jobs, and more people wanting money to do science than there is funding for experiments. The market is overcrowded and underfunded, and results in competition for resources. Under such conditions, sharing information with your competitors harms your ability to compete.
The question then, is if you’re going to end competition and switch to something else, what is that something else? How will you determine who gets the job or the money?
I agree that battles for scarce resources likely drive much of human behavior, and I’m not denying that the career situation for many scientists is quite dire. I also believe that a significant portion of the “want” you describe can be attributed to egotism, not necessity. Given that, I find our collective refusal to constructively engage one another using readily available tools all the more apalling.
Unless something changes, I expect that PLoS-style data sharing requirements will (and should) become the norm.