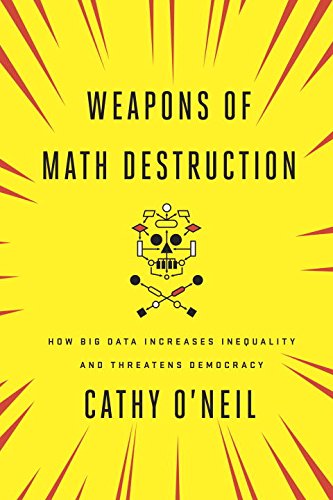
Cathy O’Neil is a mathematician and data scientist who writes a blog at mathbabe.org. Her new book, “Weapons of Math Destruction,” is just out, and it’s a blistering journey through big data’s already dangerous effects on society, and a sobering preview of what could be coming if we’re not careful.
Calling algorithms “an opinion formalized in code,” O’Neil starts by outlining her love of math, which led to a Ph.D. in mathematics from Harvard, a teaching stint at Barnard, then to a role as an analyst at the same hedge fund (D.E. Shaw) that spawned Jeff Bezos in the 1990s. Arriving at D.E. Shaw just before the 2008 financial meltdown, O’Neil became disillusioned as the separation between the algorithms and their real-life consequences vanished — what were just hypothetical tranches carrying risk-adjusted weightings became homeowners underwater, jobs lost, families displaced, and futures curtailed. Math exercises proved to be exercises in repackaging lies and deceptions that wreaked havoc on everyday people and everyday lives.
After the meltdown, hoping she could parlay her experience into more of a watchdog career, O’Neil was dismayed to find that little had changed thanks to lobbyist successes and government inaction, and that the firm she’d joined, which had been designed to provide oversight functions, proved to do little more than rubber stamp financial instruments that continued to carry undisclosed risks. Disillusioned, she left, and is now a data journalist.
As O’Neil defines it, a weapon of math destruction, or WMD, has three elements: Opacity, Scale, and Damage. Combined, these factors create traps with feedback loops, capturing victims in systems they can’t understand and can’t escape, all the while exploiting them. Of the three, Scale seems the most pernicious element, enabling Damage.
After a critique of value-add theory for teachers and a refresher course on the 2008 credit-default swaps and mortgage-backed securities that led to the Great Recession’s financial meltdown, O’Neil produces a trenchant analysis of the U.S. News & World Report college rankings. After reading this chapter about how higher education has become captured by a big data system, the limitations and difficulties of the Impact Factor seem downright charming.
Whatever U.S. News & World Report measures is what colleges seek to optimize. The almost-blind pursuit of improving these metrics increases marketing spending, leads to investments in new buildings and stadiums, and provokes a number of other expensive endeavors that ultimately end up costing students more without adding educational value (unless you count acquired cynicism as part of a formal education). Combined with the well-documented cuts in state funding of universities, all of this ends up on students’ backs at public and private colleges and universities.
Predictably, anything that can be measured can be manipulated, and universities are not above these hijinks. Noting how universities both game the system and have been caught cheating outright, O’Neil speaks to the unintended consequences, craven choices, and inherent unfairness of the rankings. For example, if affordability had been built in to the rankings from the beginning, Harvard and Yale likely would have been low-ranked, which would have kept the U.S. News & World Report rankings from having face validity in the early days, limiting their uptake and commercial success. Affordability had to be downplayed for the metrics to appear to match pre-existing notions of how universities ranked. Affordability measures have not been included ever since.
Sometimes, universities go too far in their efforts to manipulate the rankings. Some of the scandals O’Neil mentions were new to me. For instance, Baylor University once paid the fee for its incoming freshman to retake the SAT, so that Baylor could generate higher scores in its reporting to U.S. News. Bucknell and Claremont McKenna sent false data, inflating the SAT scores of their incoming freshmen. Iona College, in 2011, fudged numbers about test scores, acceptance and graduation rates, freshman retention, student-faculty ratio, and alumni giving. Other scandals in the chapter range from corrupt to sadly hilarious.
The U.S. News & World Report WMD is opaque because 25% of the score — a portion that is weighted to have even more influence — is based on subjective opinions of peers and students and alumni. It has scale, because everyone uses it. And it does damage by driving inequality in higher education. This happens for a number of reasons — the higher cost of college driven by the competition for rankings; the data-driven exercise of applying to universities, which only well-off students and families can really ace; and the emphasis on test scores and other academic measures, which students from marginalized areas or low-income areas struggle to achieve. In short, the system informed by U.S. News & World Report’s rankings ensures that the rich remain on top of the heap.
Big data in higher education is also eroding the concept of a “safety school,” as these schools proactively reject high-achieving students because they are unlikely to attend or matriculate, and because actively rejecting them drives up their standings. Combined with pressures at top schools to have low acceptance rates, this can leave good students with no options — their “reach” schools reject them, their “safety” schools reject them, and they end up caught in a data-driven no-man’s land.
There are consulting services designed to help universities to treat their student body like an investment portfolio, balancing assets (top students) and liabilities (students who need financial aid). These add yet more costs. As O’Neil writes:
If you think about it, the college admissions game, while lucrative for some, has virtually no educational value. The complex and fraught production simply re-sorts and reranks the very same pool of eighteen-year-olds in newfangled ways. . . . All of them, from the rich to the working class, are simply being trained to fit into an enormous machine — to satisfy a WMD.
The analysis of for-profit colleges is even more alarming. As the book was published just as ITT Technical Institute was being shuttered, this section is very timely. Again, the big data techniques for exploitation are amazing at times, and O’Neil employs the term “predatory” with good justifications. Using targeted online ads, for-profit colleges have been trolling for people who might fall for their questionable — and sometimes outright false — promises. They target veterans (who have an easier time getting federal educational loans) and people going through trying times (divorce, job loss, etc.), using Google searches for related words and phrases to put for-profit college ads in place.
Some companies go even further. In one instance, a company placed false job ads on Monster.com to glean contact information and details. As quickly as five minutes after filling out a bogus online job ad, a person might get a phone call from a for-profit college recruiter, who seemed to know everything about them. One person received 180 such calls in a single month.
Marketing spending in both cases — for traditional universities under the yoke of the U.S. News WMD and with for-profit colleges trying to use the ad targeting WMD to exploit federal educational grant money — is growing rapidly. For example, in 2010, the University of Phoenix spent $2,225 per student on marketing and only $892 per student on instruction. Meanwhile, Portland Community College in Oregon spent $5,953 per student on instruction and $185 per student on marketing. Clearly, priorities are different when a WMD is involved. And let’s not forget, the University of Phoenix has its name on a world-class football stadium, yet has no football team of its own. The stadium’s naming rights are all about marketing.
It’s not just higher education being distorted and changed by big data wielded with purpose. Workplaces, our justice system, the insurance marketplace, and politics are all being changed by WMDs of various types.
In one interesting example, O’Neil describes how retail and service employees are working stranger shifts and shorter hours in the era of big data, as owners understand and schedule workers based on how busy the store or restaurant is expected to be. Rather than a predictable 40-hour week, with the predictable pay and downtime this might bring, workers are finding their schedules and pay both made unpredictable as they are asked to work more and shorter shifts, at strange hours, and without much notice. The hardships — both in lifestyle and pay — can be significant, but the WMD is unrelenting. As O’Neil writes:
It’s almost as if the software were designed expressly to punish low-wage workers and to keep them down.
Algorithms created to improve efficiencies in policing, hiring, sentencing, and so forth lack one primary element — feedback. They render verdicts but without any follow-up to see if their judgments were correct. Worse, because they do this at scale, one decision point can become destiny for workers, who are flagged once and then in every system from then on. Gone are the idiosyncracies of a less data-driven economy, when a quirky applicant might catch the eye of a canny boss who can see potential. Instead, systems broker and break opportunities with impunity. Stories of their effects — surprising, rife with inequality, illustrative of exploitation — fill the pages of O’Neil’s book.
One particularly resonant section about big data and crime shows how recursive these programs become. O’Neil rightly, I believe, asserts that if police started looking for crime in white, affluent neighborhoods with the tenacity and big data algorithms they use in impoverished neighborhoods, we’d probably be inundated with stories of rampant drug use, drunk driving, petty theft, infidelity, financial crimes, and so forth in these neighborhoods. But the programs being used specifically ignore these areas and people. Because they rely on historical data, there is racism and class politics built into their assumptions. Big data is, in fact, doubling-down on historical biases against the poor and minorities.
O’Neil also points to big data as a potential contributor to our age of fragmented and polarized opinions and politics. By enabling the microtargeting of special interests, big data tools have ensured that some Americans will continue to be fed the idea that President Obama was not born in America, while others will continue to be fed the belief that vaccines cause autism, and yet others will be fed the theory that Hillary Clinton suffers from Parkinson’s disease. The list of micro-tribes being fed self-perpetuating lies in the swirl of big data is nearly endless. Microsites and microtargeting actively perpetuate these divergent groups. Big data’s scale actively fragments our society and stalls progress.
The solutions to these big data problems aren’t entirely clear, but O’Neil has more than a few ideas — give people the data they need in tools they can use, so that the data governing their lives aren’t opaque and don’t scale without accountability; begin enacting actual laws and social programs to make sure big data algorithms don’t become de facto social policies but rather put in place social policies that make sense for society rather than for businesses; make the objectives of data systems include concepts like fairness and equality; and build in follow-up and feedback, so that the system is not self-fulfilling but open for revision.
Her best idea is to demand that big data techniques and algorithms be audited — that is, their assumptions, sources, and techniques exposed and examined, and then compared to reality and to social concepts like “equal protection under the law.” Most big data practices would fail on this alone — either by showing themselves to be unwilling to be audited, having core statistical or conceptual flaws exposed upon audit, or being revealed as inherently biased against the poor and socially disenfranchised.
So-called “open data” is not one of her solutions. In fact, she writes in one section detailing the abuses involved in leveraging credit reports into hiring practices:
. . . “The more data, the better” is the guiding principle of the Information Age. Yet in the name of fairness, some of this data should remain uncrunched.
The misapplication of data within false premises, biased frameworks, or exploitative situations is perhaps a greater threat to our society than we’ve been led to believe.
“Weapons of Math Destruction” is an opinionated book, and it may leave you a bit distraught. However, it’s also important and timely. It hits on exactly how big data is being used to control behaviors, drive inequality, and change our society. Big data is not a novelty anymore — you are probably encountering WMDs every day, perhaps without knowing it or how they affect you.
Discussion
10 Thoughts on "Book Review — The Traps of Big Data Revealed in "Weapons of Math Destruction" by Cathy O’Neil"
Good stuff, but it seems to be more about Big Algorithm than Big Data. My favorite example is automated stock trading algorithms that precipitate crashes. As I understand the big data movement, it is more about discovery than decision making.
Nice review, Kent.
Part of what bothers me about predicting human behavior based on aggregate past behavior is that it assumes we are fixed and immutable, unable to change, remake ourselves, right our wrongs, grow and become new people. Today’s algorithms assume that our proclivities and predispositions are blueprints of our growth and potential. We all need to be given some slack and second chances. It is possible to build uncertainty into algorithms; however, the decision to do so is often left at the hands of the programmer.
NPR did a nice interview with the author last night: http://www.npr.org/2016/09/12/493654950/weapons-of-math-destruction-outlines-dangers-of-relying-on-data-analytics
Great review. Outlines the need for better data – skills, understanding and (recursive) approaches [all the best Star Trek algorithms are recursive]. Then we get to experiment with change – what will the data say if we do x? Use it not for discrimination but to help see the bigger picture.
Just to take partial exception to the critique of US News & World Report college rankings and the implication that these are highly subject to gaming: in the case of my alma mater Princeton, which has been ranked #1 for some years now, I believe a principal reason it gets that ranking is because it is graded as #1 in Best Undergraduate Teaching. Princeton has long prided itself on its strong focus on undergraduate education, among top research universities, going back to the days when Woodrow Wilson was president and came up with the idea for the “precept,” a small seminar that became a distinctive feature of the undergraduate education experience at Princeton. Also, all Princeton faculty, no matter how senior or distinguished, teach undergraduates–again, a longstanding practice. These were in place long before college rankings even began; hence there was no need for any manipulation of data.
Perhaps that is a factor in the current rankings, but a few points to consider. First, the rankings are opaque, so we don’t know whether the undergraduate teaching practices matter or not. This is a problem with the WMD — opacity. Also, as noted in the review and the book, “affordability” is not included. If it were, Princeton’s ranking may fall, as there are many other colleges and universities that are more affordable and doing the same thing when it comes to undergraduate teaching. Finally, there is no audit mechanism, so we don’t know if Princeton graduates fare better in life than other graduates, which could indicate whether the ranking is truly justified.
The book rails on algorithms because they do not have human intervention, no feedback, no way to capture idiosyncacies. But then there is this:
“The U.S. News & World Report WMD is opaque because 25% of the score — a portion that is weighted to have even more influence — is based on subjective opinions of peers and students and alumni.”
Puzzling, because it’s an attempt to incorporate the human element from people with direct experience with the universities. And of the course prospective applicants will also visit the campus and talk to alumni.
This book is an attack on the systematic use of data. We should make all decisions by anecdotes alone?!?